Statistic Ratio Attention-Guided Siamese U-Net for SAR Image Semantic Change Detection
IEEE GEOSCIENCE AND REMOTE SENSING LETTERS(2024)
摘要
Semantic change detection (SCD), which aims to locate land cover changes and identify their categories using pixel-level boundaries, has promising applications in Earth vision, including precise urban planning and natural resource management. This letter proposes a novel Siamese U-Net architecture for SCD in synthetic aperture radar (SAR) images, incorporating a residual network with weight-sharing as the backbone network. The network is capable of simultaneously yielding binary change detection (CD) and SCD results. Additionally, we have designed a statistic ratio attention module that utilizes statistical features from the original image as spatial ratio attention, coupled with channel attention, to extract change information from the bi-temporal SAR images. Furthermore, as there is currently no existing dataset for SCD in SAR images, we have constructed a dedicated dataset to facilitate model training and evaluation. Our experiment results demonstrate the superiority of our proposed model over other comparison algorithms.
更多查看译文
关键词
Change detection (CD),convolutional neural network (CNN),remote sensing,semantic CD (SCD),statistic ratio attention
AI 理解论文
溯源树
样例
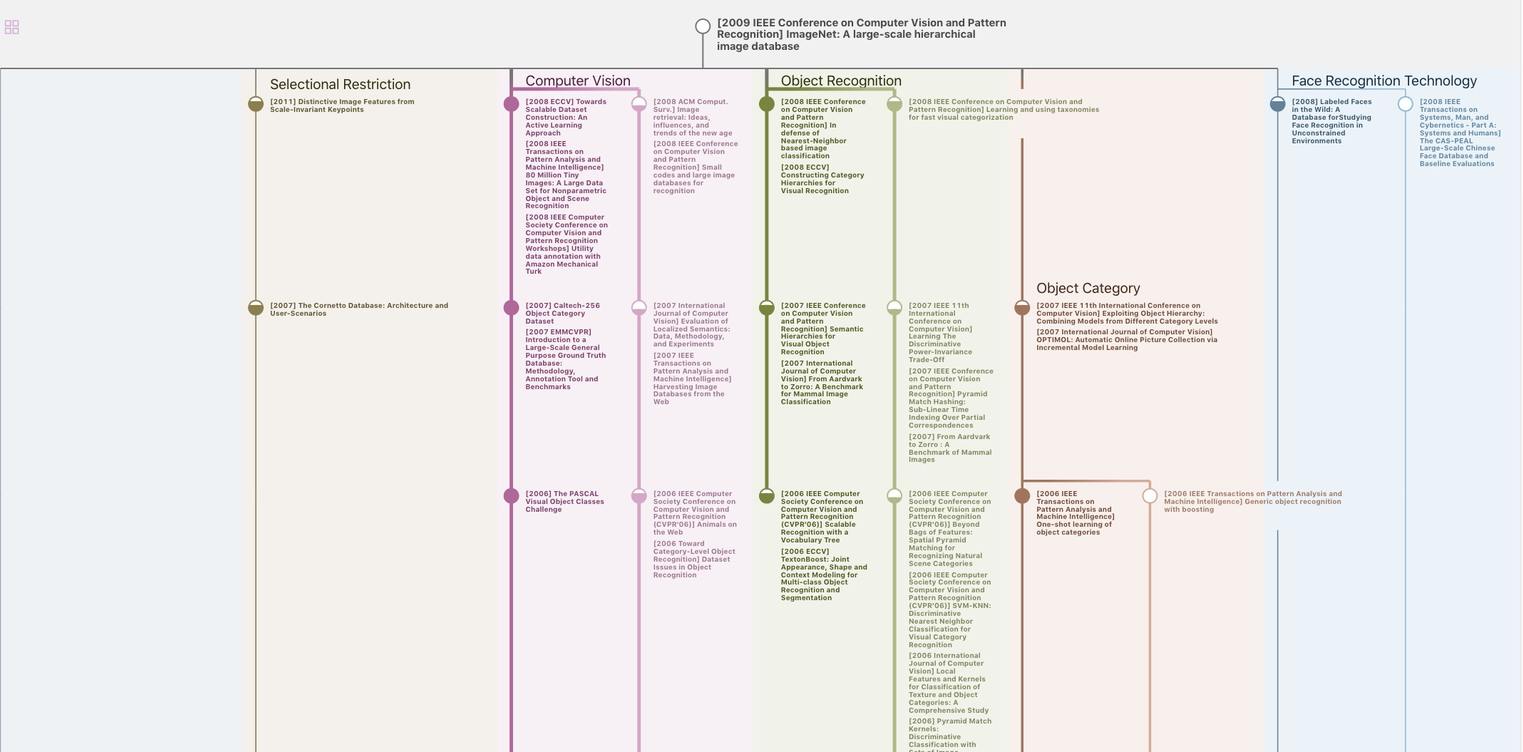
生成溯源树,研究论文发展脉络
Chat Paper
正在生成论文摘要