Spatial-Spectral-Temporal Deep Regression Model With Convolutional Long Short-Term Memory and Transformer for the Large-Area Mapping of Mangrove Canopy Height by Using Sentinel-1 and Sentinel-2 Data
IEEE TRANSACTIONS ON GEOSCIENCE AND REMOTE SENSING(2024)
摘要
Mangrove canopy height information is crucial to carbon stock and biomass analyses. However, estimation of this height is challenging because of the large areas involved, and the field conditions of mangrove forests. Remote sensing satellite imagery has been used for canopy height mapping because it offers several advantages. This study developed a spatial-spectral-temporal deep learning regression model with convolutional long short-term memory (ConvLSTM) and transformer (hereafter referred to as the SST-CLT model) to map mangrove canopy height over a large area. The SST-CLT model consists of two submodels trained simultaneously. The first submodel is a fusion extractor to extract spatial-spectral-temporal information from Sentinel-1 time-series data by using a ConvLSTM. It also extracts spatial-spectral information from Sentinel-2 data using a 2-D convolutional block. The second submodel is a regressor that contains a Swin transformer (SWINTF) and a final convolutional regression layer. Data from the light detection and ranging (LiDAR) canopy height model (CHM) were employed as the target data to train the proposed model. The SST-CLT model was tested on two datasets collected from Florida: a large dataset for the Everglades National Park (ENP) and a small dataset for the Charlotte Harbor Preserve State Park (CHPSP). The SST-CLT model achieved a mean absolute error (MAE) of 1.924 and 1.913 m for the ENP and CHPSP datasets, respectively. Moreover, it achieved root mean square error (RMSE) values of 2.471 and 2.440 m for these datasets, respectively. The SST-CLT model was compared with that of other regression models. The results indicated that the MAE and RMSE of the proposed SST-CLT were lower than those of the other models (https://github.com/ilhamjamaluddin/SST-CLT).
更多查看译文
关键词
Canopy height,deep learning,light detection and ranging (LiDAR),mangrove,regression,Sentinel-1,Sentinel-2
AI 理解论文
溯源树
样例
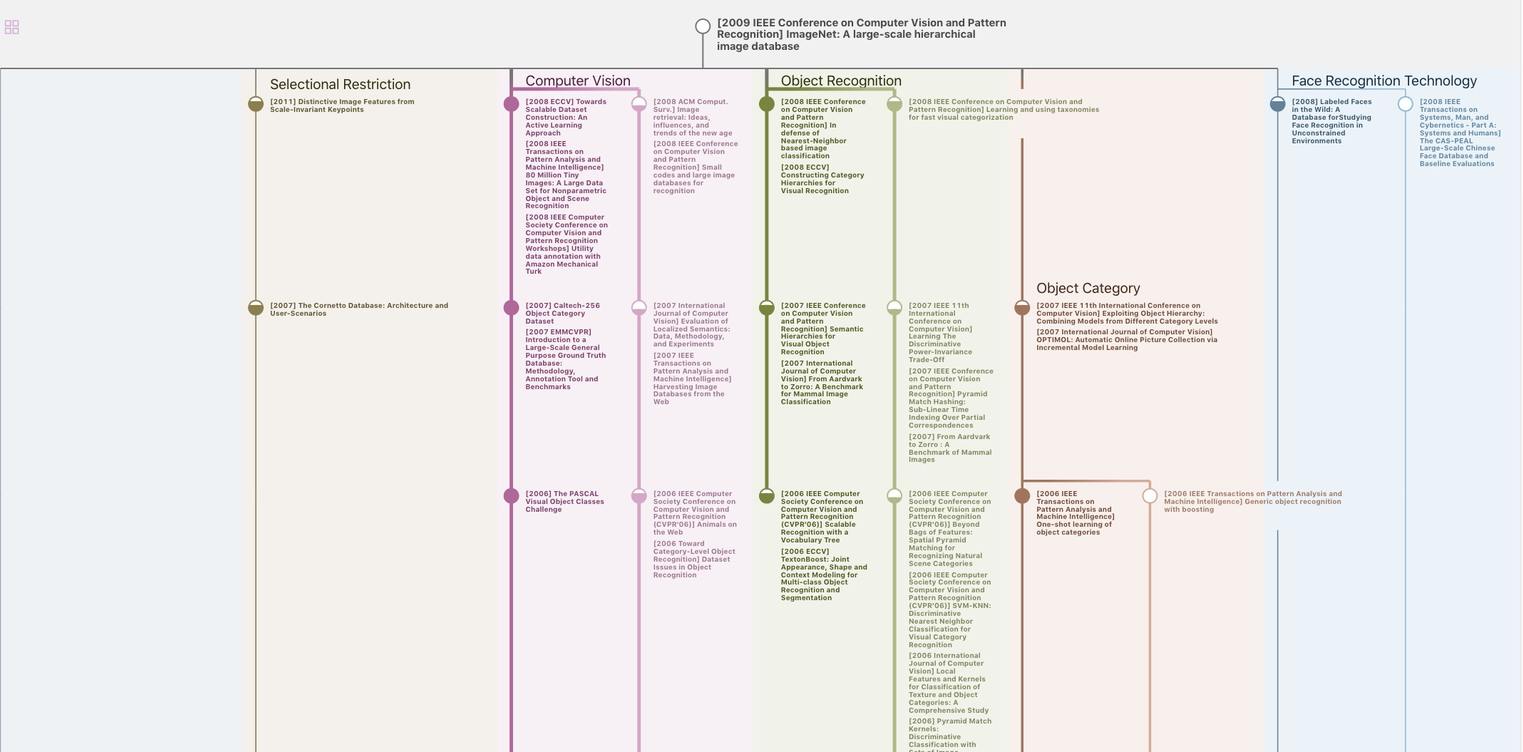
生成溯源树,研究论文发展脉络
Chat Paper
正在生成论文摘要