Reconstructing the Geometry of Random Geometric Graphs
CoRR(2024)
摘要
Random geometric graphs are random graph models defined on metric spaces.
Such a model is defined by first sampling points from a metric space and then
connecting each pair of sampled points with probability that depends on their
distance, independently among pairs. In this work, we show how to efficiently
reconstruct the geometry of the underlying space from the sampled graph under
the manifold assumption, i.e., assuming that the underlying space is a low
dimensional manifold and that the connection probability is a strictly
decreasing function of the Euclidean distance between the points in a given
embedding of the manifold in ℝ^N. Our work complements a large body
of work on manifold learning, where the goal is to recover a manifold from
sampled points sampled in the manifold along with their (approximate)
distances.
更多查看译文
AI 理解论文
溯源树
样例
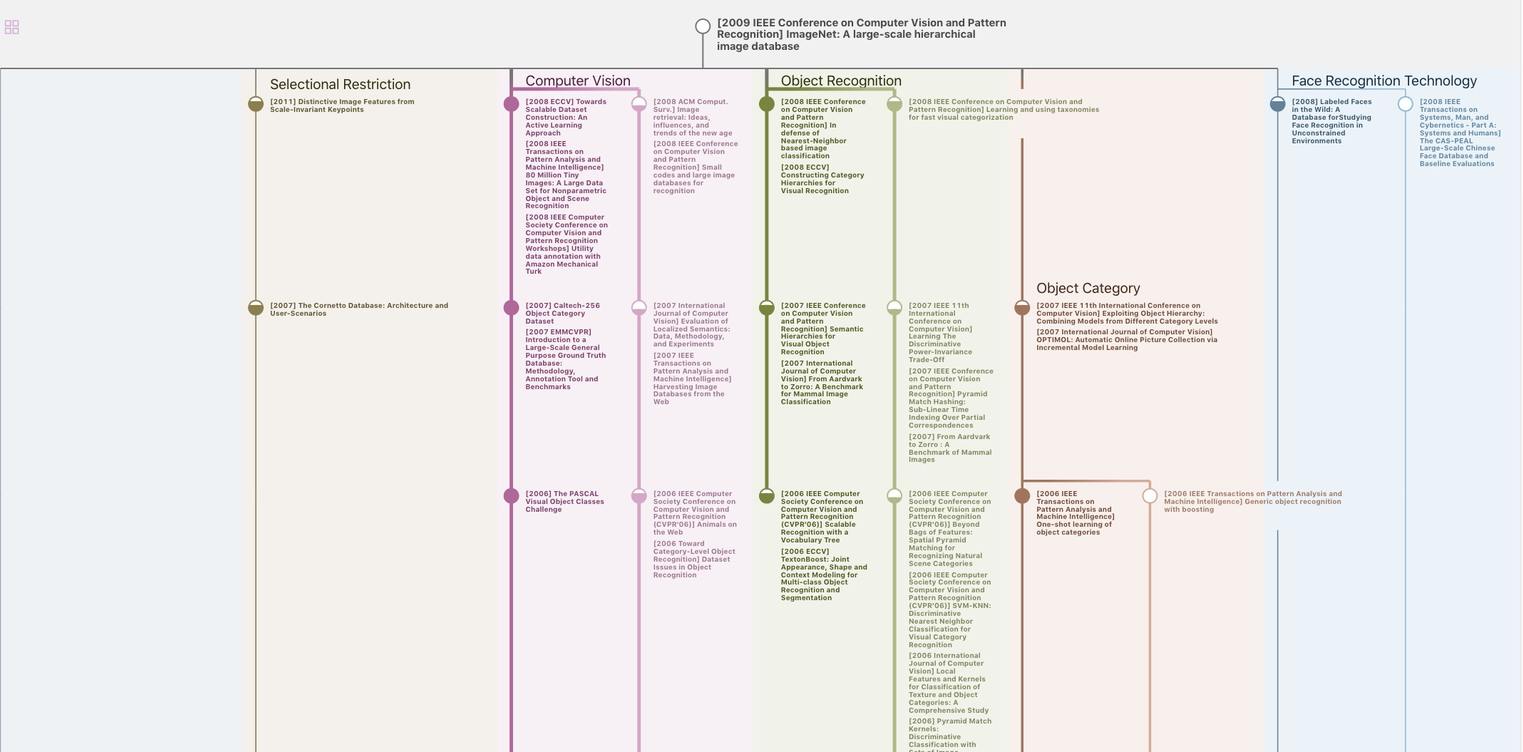
生成溯源树,研究论文发展脉络
Chat Paper
正在生成论文摘要