The Manifold Density Function: An Intrinsic Method for the Validation of Manifold Learning
CoRR(2024)
摘要
We introduce the manifold density function, which is an intrinsic method to
validate manifold learning techniques. Our approach adapts and extends Ripley's
K-function, and categorizes in an unsupervised setting the extent to which an
output of a manifold learning algorithm captures the structure of a latent
manifold. Our manifold density function generalizes to broad classes of
Riemannian manifolds. In particular, we extend the manifold density function to
general two-manifolds using the Gauss-Bonnet theorem, and demonstrate that the
manifold density function for hypersurfaces is well approximated using the
first Laplacian eigenvalue. We prove desirable convergence and robustness
properties.
更多查看译文
AI 理解论文
溯源树
样例
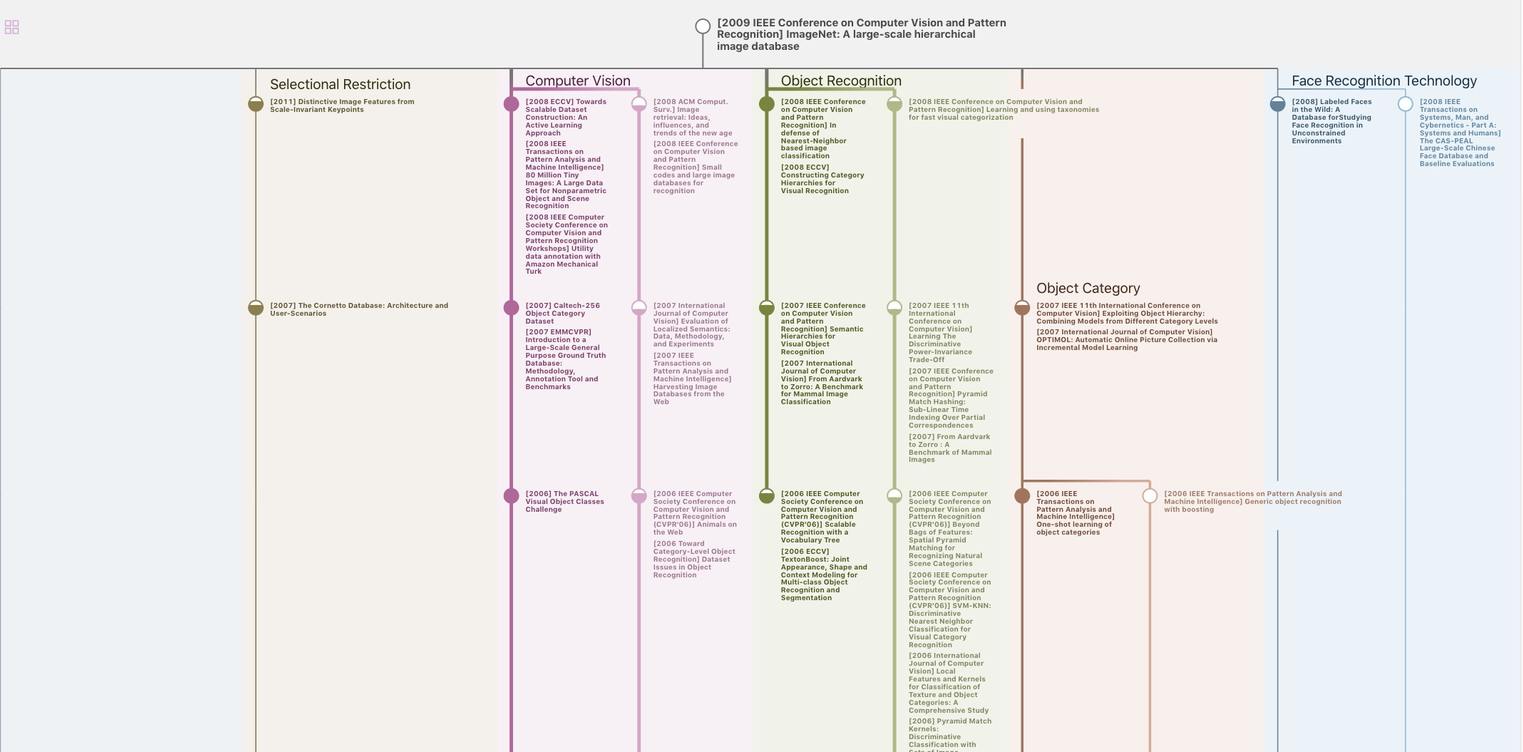
生成溯源树,研究论文发展脉络
Chat Paper
正在生成论文摘要