Diving into Clarity: Restoring Underwater Images using Deep Learning
Journal of Intelligent & Robotic Systems(2024)
摘要
In this paper we propose a learning-based restoration approach to learn the optimal parameters for enhancing the quality of different types of underwater images and apply a set of intensity transformation techniques to process raw underwater images. The methodology comprises two steps. Firstly, a Convolutional Neural Network (CNN) Regression model is employed to learn enhancing parameters for each underwater image type. Trained on a diverse dataset, the CNN captures complex relationships, enabling generalization to various underwater conditions. Secondly, we apply intensity transformation techniques to raw underwater images. These transformations collectively compensate for visual information loss due to underwater degradation, enhancing overall image quality. In order to evaluate the performance of our proposed approach, we conducted qualitative and quantitative experiments using well-known underwater image datasets (U45 and UIEB), and using the proposed challenging dataset composed by 276 underwater images from the Amazon region (AUID). The results demonstrate that our approach achieves an impressive accuracy rate in different underwater image datasets. For U45 and UIEB datasets, regarding PSNR and SSIM quality metrics, we achieved 26.967, 0.847, 27.299 and 0.793, respectively. Meanwhile, the best comparison techniques achieved 26.879, 0.831, 27.157 and 0.788, respectively.
更多查看译文
关键词
Underwater image restoration,Deep learning,Learning-based image enhancement,Intensity transformation techniques
AI 理解论文
溯源树
样例
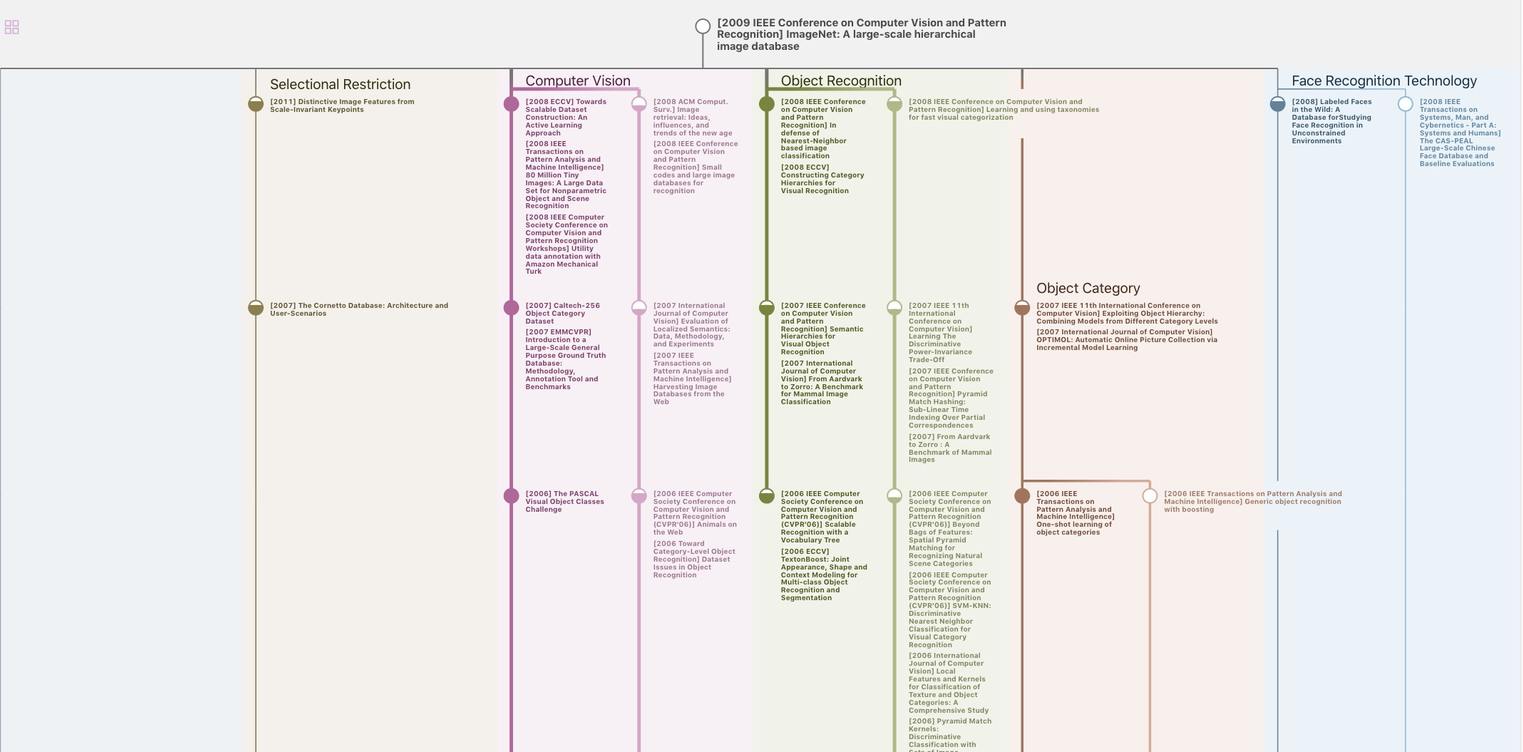
生成溯源树,研究论文发展脉络
Chat Paper
正在生成论文摘要