KL-Weighted Graph Sparse Self-Representation for Unsupervised Hyperspectral Band Selection
2023 International Conference on Image Processing, Computer Vision and Machine Learning (ICICML)(2023)
摘要
Unsupervised band selection (BS) methods have attracted much attention in hyperspectral imagery (HSI), which can select informative bands to solve the problems of information redundancy and high computational complexity. In this paper, we propose a KL-weighted graph sparse self-representation (SSR) method for unsupervised BS, in which the dissimilarity of bands measured via KL divergence is integrated into the superpixel-based graph SSR model by weighting the sparse representation coefficient matrix. An alternating optimization algorithm is designed to obtain the optimal coefficient matrix and the representative bands are finally selected by the norm ranking of rows of the coefficient matrix. Experimental results on HIS datasets show the effectiveness of the proposed BS algorithm and it outperforms other related BS methods in selecting proper representative bands for classification.
更多查看译文
关键词
hyperspectral imagery (HIS),band selection (BS),sparse self-representation (SSR),Kullack-Leibler (KL) divergence
AI 理解论文
溯源树
样例
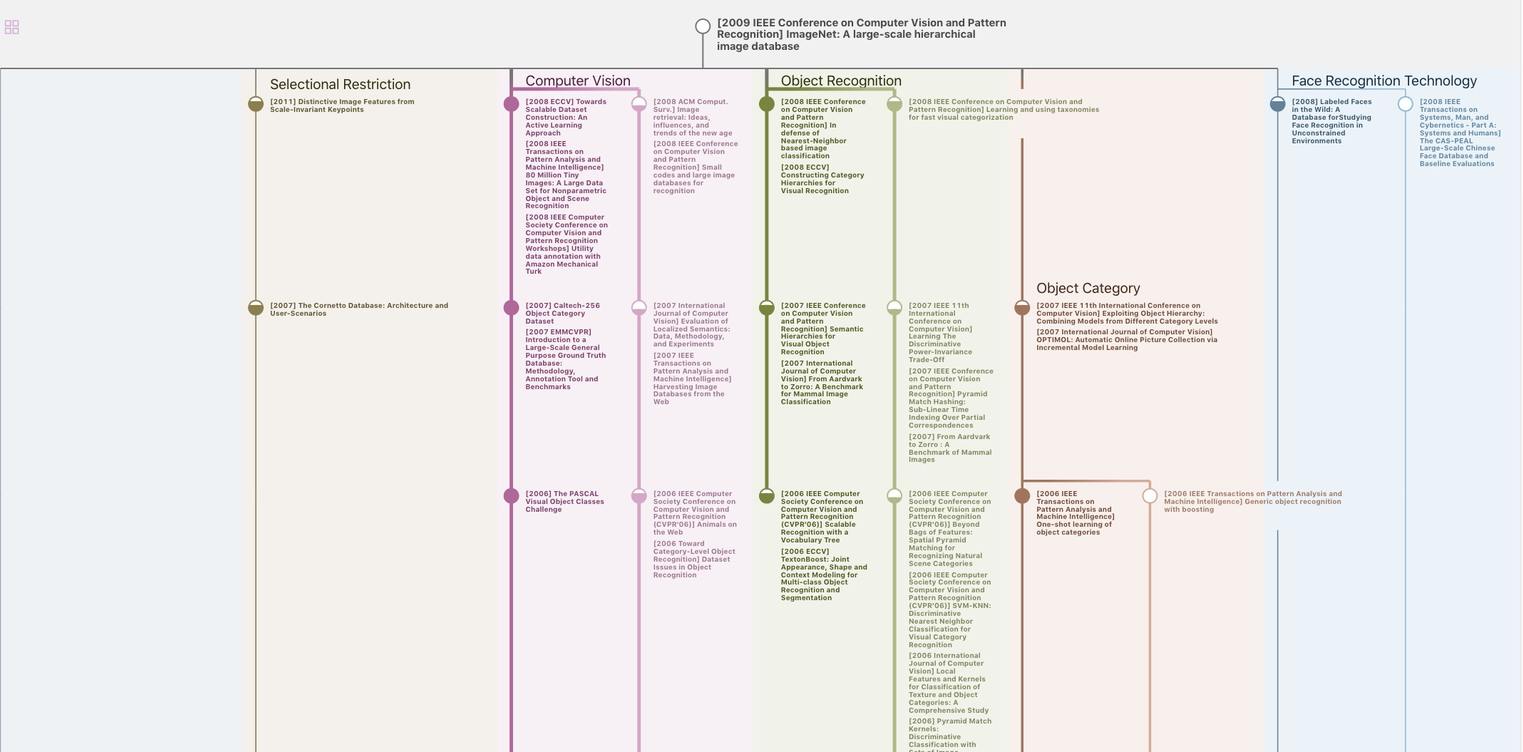
生成溯源树,研究论文发展脉络
Chat Paper
正在生成论文摘要