Problem-Parameter-Free Decentralized Nonconvex Stochastic Optimization
CoRR(2024)
摘要
Existing decentralized algorithms usually require knowledge of problem
parameters for updating local iterates. For example, the hyperparameters (such
as learning rate) usually require the knowledge of Lipschitz constant of the
global gradient or topological information of the communication networks, which
are usually not accessible in practice. In this paper, we propose D-NASA, the
first algorithm for decentralized nonconvex stochastic optimization that
requires no prior knowledge of any problem parameters. We show that D-NASA has
the optimal rate of convergence for nonconvex objectives under very mild
conditions and enjoys the linear-speedup effect, i.e. the computation becomes
faster as the number of nodes in the system increases. Extensive numerical
experiments are conducted to support our findings.
更多查看译文
AI 理解论文
溯源树
样例
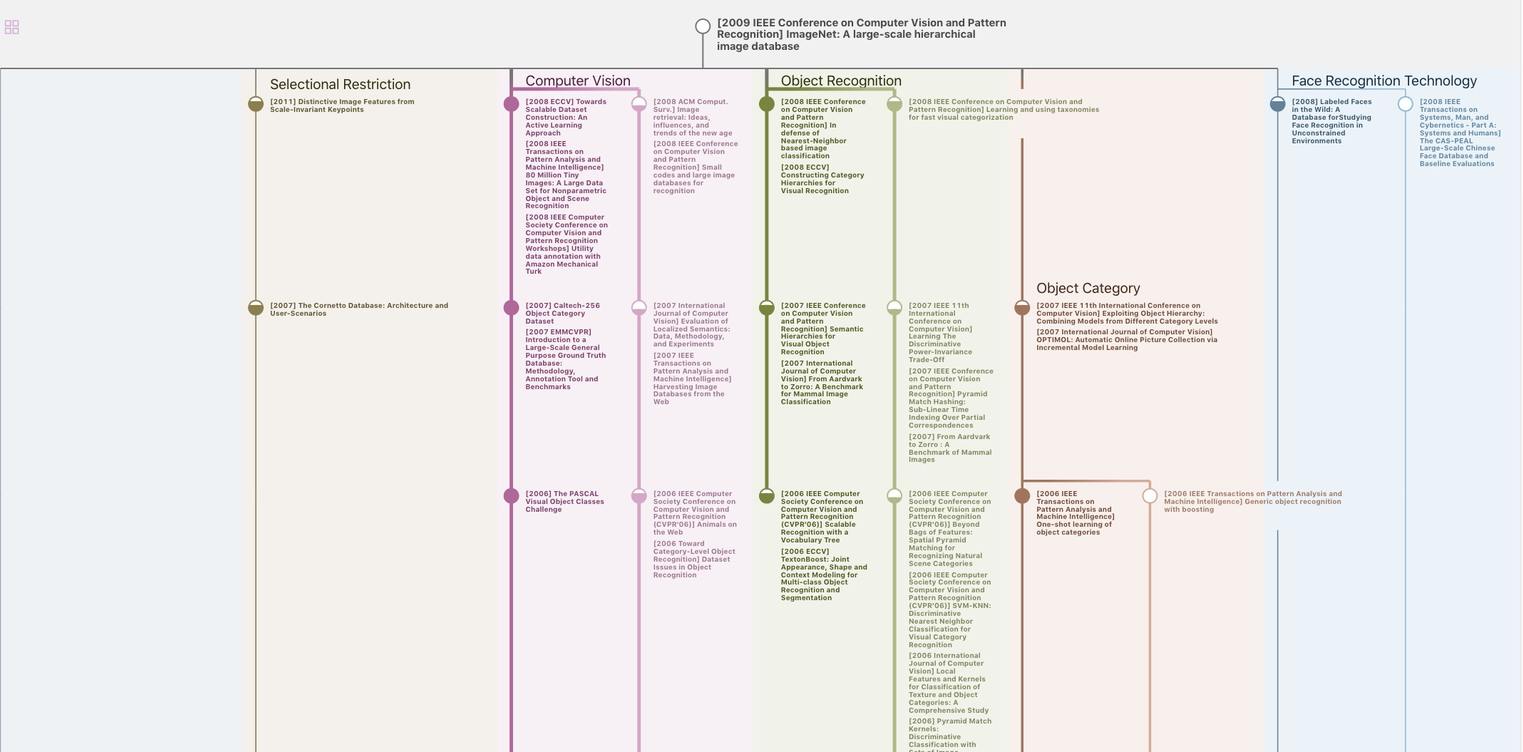
生成溯源树,研究论文发展脉络
Chat Paper
正在生成论文摘要