Data Augmentation for Pedestrians in Corner Case: A Pose Distribution Control Approach.
2023 IEEE 26th International Conference on Intelligent Transportation Systems (ITSC)(2023)
摘要
While data augmentation serves as a potent strategy to encompass corner cases in autonomous driving, scant research has attended to the imperative of maintaining categorical balance post-augmentation. Hereby, We introduce a novel data augmentation technique designed to proficiently regulate the fine-grained distribution of pedestrian poses within datasets, thereby amplifying the efficacy of pedestrian recognition algorithms in traffic situations. Our approach is founded on a unique quadratic categorization of human pose situations, using corresponding pose estimation confidence scores. This methodology strives to precisely adjust the proportion of poses within differing confidence score ranges. Experimental outcomes demonstrate that our approach not only enhances the distribution of pedestrian poses in traffic image datasets, but also significantly boosts the performance of pedestrian detectors in final.
更多查看译文
关键词
Data Augmentation,Corner Cases,Pose Distribution,Confidence Score,Pose Estimation,Data Augmentation Techniques,Human Pose,Pedestrian Detection,Human Model,S Phase,Aspect Ratio,F1 Score,Latent Space,Latent Vector,Data Augmentation Methods,Human Pose Estimation,COCO Dataset,3D Human Model
AI 理解论文
溯源树
样例
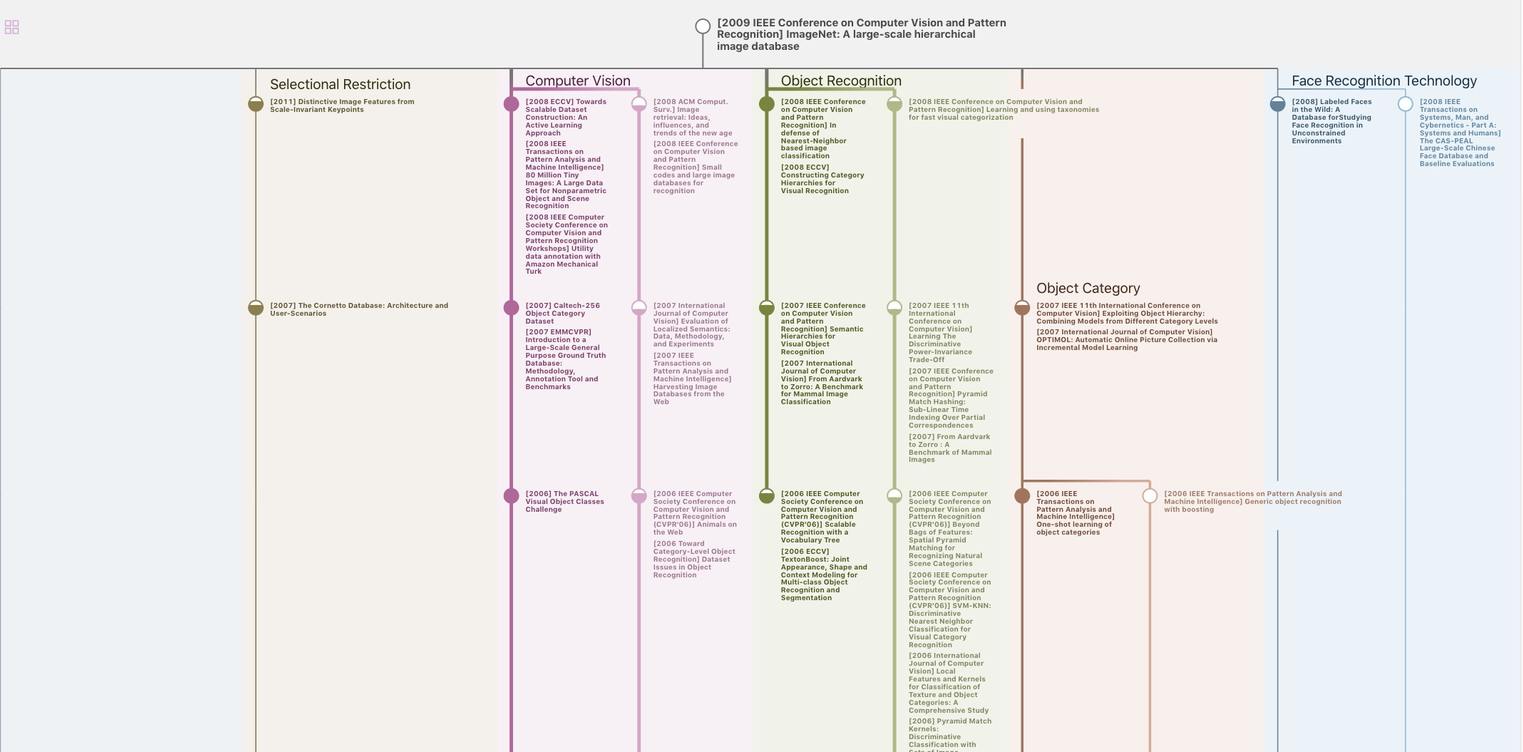
生成溯源树,研究论文发展脉络
Chat Paper
正在生成论文摘要