Point Cloud Completion by Larger Receptive Fields for Intelligent Vehicles.
2023 IEEE 26th International Conference on Intelligent Transportation Systems (ITSC)(2023)
摘要
Point cloud completion is a critical task for intelligent vehicles in the Intelligent Transportation Systems (ITS). Due to the disordered nature of point clouds, current point-based point completion methods utilize max pooling operations and Convolutional Neural Networks (CNNs) with a convolution kernel size of 1 to extract point features. However, a network with a kernel size of 1 has a small receptive field and can only extract one point feature at a time; as a result, such a network struggles to precisely extract highly abstracted features. To address this issue, we propose a point cloud completion network with larger receptive fields, termed PLRF-Net, which is composed of two parts: a reconstruction network and a completion network. The reconstruction network focuses on repairing the structures of partial points and includes an offset-attention embedding module, a rearrangement module, several convolution layers with varying kernel sizes, a pooling and repeating layer, and transposed convolution layers with different kernel sizes. Meanwhile, the completion network strives to improve the precision of the completed point cloud and consists of several convolution layers, along with a downsampling and upsampling module. Experimental results indicate that our approach achieves superior quantitative and qualitative outcomes on both the Multiview Partial Point Cloud (MVP) and KITTI datasets.
更多查看译文
关键词
Large Field,Point Cloud,Receptive Field,Large Receptive Field,Point Cloud Completion,Convolutional Neural Network,Convolutional Layers,Qualitative Results,Convolution Kernel,Feature Points,Complete Network,Network Reconstruction,Intelligent Transportation Systems,Partial Point,Max-pooling Operation,KITTI Dataset,Small Receptive Field,Transposed Convolution Layers,Local Features,K-nearest Neighbor,Point Cloud Features,Earth Mover’s Distance,Cloud Features,Voxel-based Methods,Transformer-based Methods,Input Point Cloud,Multilayer Perceptron,Semantic Segmentation,Close Points,Mechanism Module
AI 理解论文
溯源树
样例
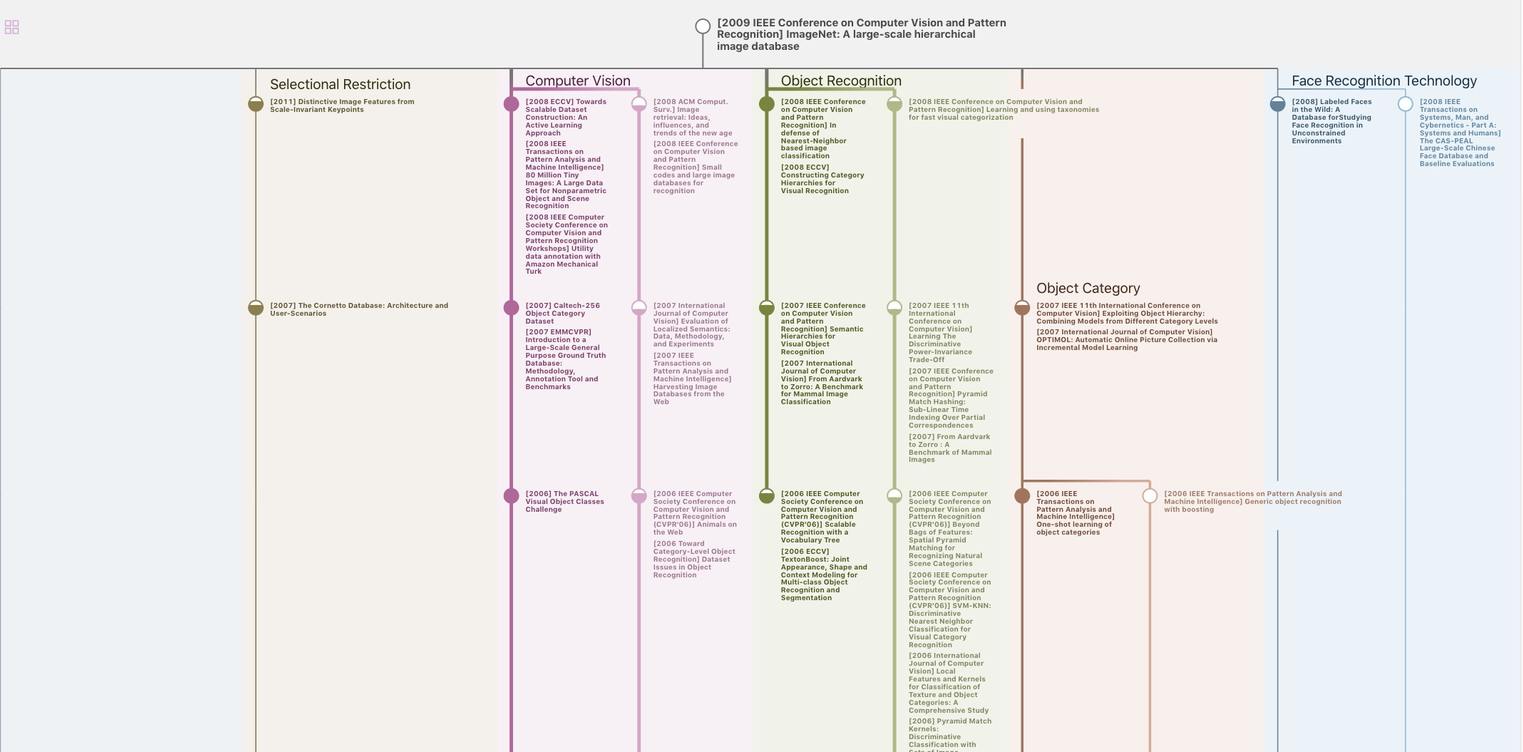
生成溯源树,研究论文发展脉络
Chat Paper
正在生成论文摘要