TSCAPE: time series clustering with curve analysis and projection on an Euclidean space
CONNECTION SCIENCE(2024)
摘要
The ever-growing use of digital systems has led to the accumulation of vast datasets, particularly time series, depicting the temporal evolution of variables and systems. Analysing these time series presents a tremendous challenge due to their inherent complexity and heterogeneity. Addressing an industrial need in the pharmaceutical wholesale sector, this paper introduces a new clustering method for time series: TSCAPE. The TSCAPE method uses a distance matrix calculated using dynamic time warping, followed by multidimensional scaling to project time series into a 2D Euclidean space, thus improving the last clustering stage by K-Means. Unlike conventional techniques, this approach, based on clustering of representation of distances in a Euclidean plane rather than on the curve shape, directly enhances the efficiency of the clustering process. The methodology exhibits significant potential for diverse applications, accommodating varied data types and irregular time series shapes. The research compares multiple variants and proposes metrics to assess their effectiveness on two open-access datasets. The results demonstrate the method's superiority over "only distance comparison clustering techniques", like dynamic time warping and K-Means, with future prospects aimed at predictive applications and refining the clustering process by exploring alternative, more powerful clustering algorithms.
更多查看译文
关键词
Time series clustering,curves similarity,dynamic time warping,Euclidean embedding representation,neighbourhood distance clustering
AI 理解论文
溯源树
样例
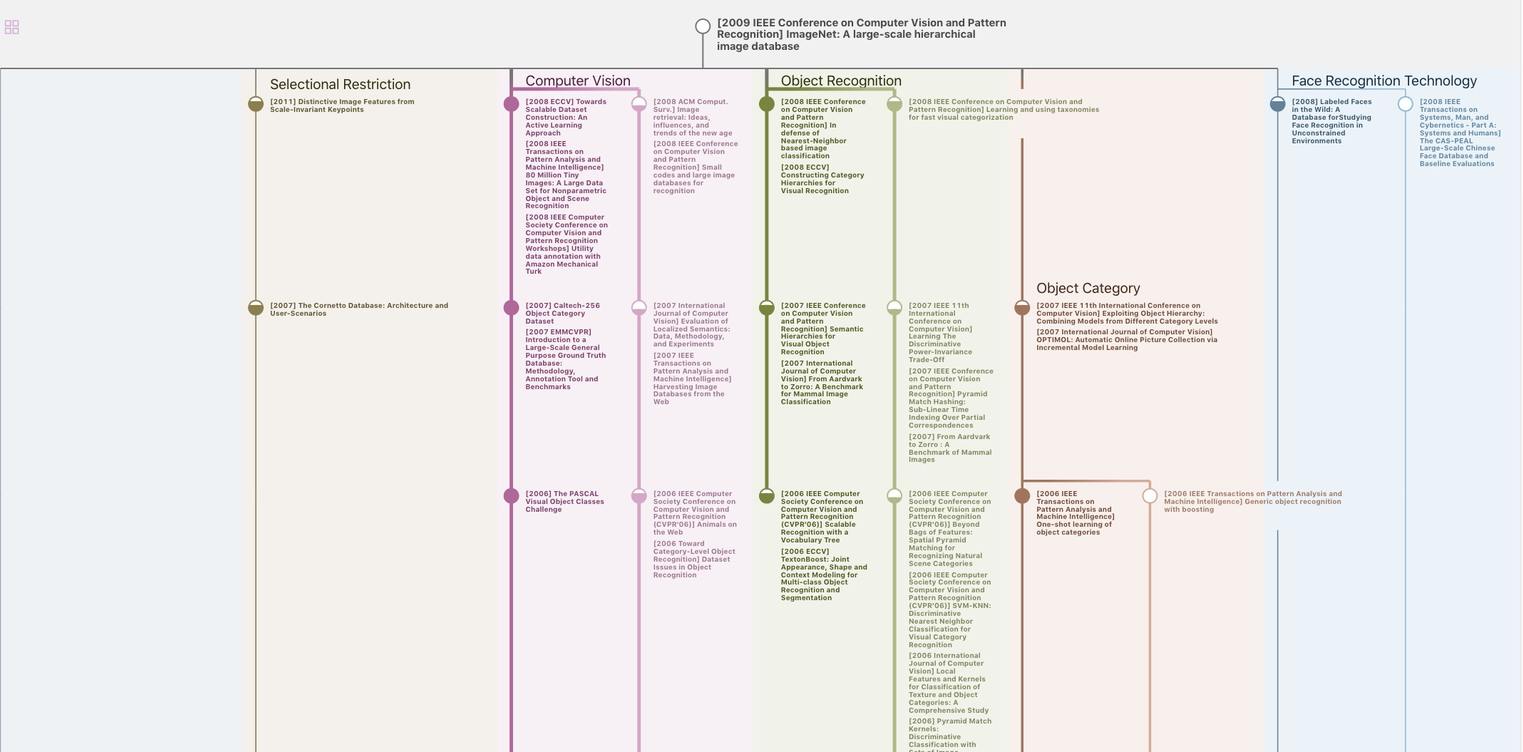
生成溯源树,研究论文发展脉络
Chat Paper
正在生成论文摘要