Physics-Guided Graph Convolutional Network for Modeling Car-Following Behaviors Under Distributional Shift.
2023 IEEE 26th International Conference on Intelligent Transportation Systems (ITSC)(2023)
摘要
Recent works on data-driven car-following modeling have shown that a Graph Convolutional Network (GCN)-based car-following model (CFM) can outperform other data-driven models such as Long Short-term Memory (LSTM) networks. Inspired by this result, a new physics-guided GCN-based CFM is proposed in this paper. The model has been extended from the previous GCN-based CFM by integrating a vehicle platoon graph along with other refinements. Furthermore, the first attempt to develop physics-guided graph-learning-based CFM is made in this paper by adopting physics-guided machine learning (PGML) in different modeling aspects. The effectiveness of the proposed model was demonstrated in both in-distribution and out-of-distribution scenarios, yielding safe and accurate car-following behaviors. The experiment results showed that under the most severe distributional shift, the proposed model outperformed the best pure data-driven model by approximately 26.25% and a well-calibrated physics-based model by about 11.45% in terms of collision-penalized gap root mean squared error. Future research can focus on improving the robustness of our proposed model under various distributional shifts, leveraging the insights and suggestions given in the paper.
更多查看译文
关键词
Domain Shift,Graph Convolutional Network,Car-following Behavior,Mean Square Error,Short-term Memory,Long Short-term Memory,Long Memory,Data-driven Models,Safety Behavior,Physics-based Models,Neural Network,Training Data,Time Step,Input Features,Traffic Flow,Past Conditions,Graph Neural Networks,Gated Recurrent Unit,Collision Events,Gap Distance,Gated Recurrent Unit Layer,Recurrent Neural Network Structure,Number Of Trajectories,Spatial Information Processing,Temporal Information Processing,Diagonal Degree Matrix,Model Parameter Space,Training Trajectories,Graph Learning,Speed Profile
AI 理解论文
溯源树
样例
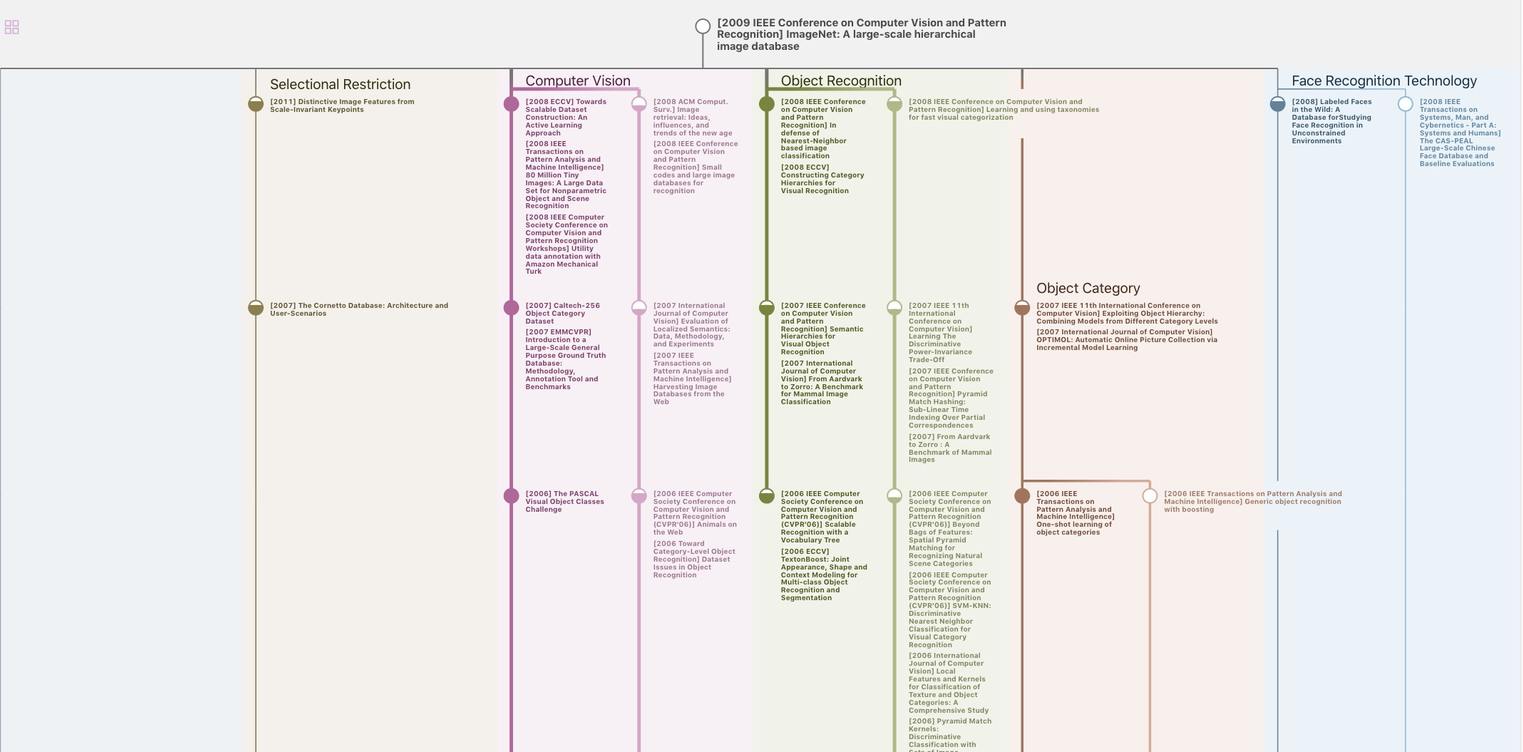
生成溯源树,研究论文发展脉络
Chat Paper
正在生成论文摘要