Augmented Reinforcement Learning with Efficient Social-Based Motion Prediction for Autonomous Decision-Making.
2023 IEEE 26th International Conference on Intelligent Transportation Systems (ITSC)(2023)
摘要
This paper presents an approach that improves the efficiency and generalization capabilities of Reinforcement Learning-based autonomous vehicles operating in urban driving scenarios. The proposed method introduces an Efficient Social-based Motion Prediction module, which predicts the future positions of vehicles within the scenario. These predictions serve as input to a Reinforcement Learning-based Decision-Making module, responsible for executing high-level actions. The Proximal Policy Optimization algorithm is employed to develop our approach. We conduct experiments in an unsignalized T-intersection scenario using the SMARTS framework, comparing our approach with and without the proposed state representation, as well as against various baseline methods. Through this study, we demonstrate that our approach achieves performance improvements, particularly in scenarios involving high velocities. Our code and qualitative results are available at https://github.com/Cram3r95/argo2goalmp.
更多查看译文
关键词
Motion Prediction,Efficient Motion,Optimization Algorithm,Autonomous Vehicles,Baseline Methods,State Representation,Vehicle Position,Proximal Policy Optimization,State Space,Multilayer Perceptron,Confidence Score,Residual Block,Reward Function,Markov Decision Process,Future Trajectories,Agent Interactions,Feature Pyramid Network,Position Of Agent,Reinforcement Learning Techniques,Road Structure
AI 理解论文
溯源树
样例
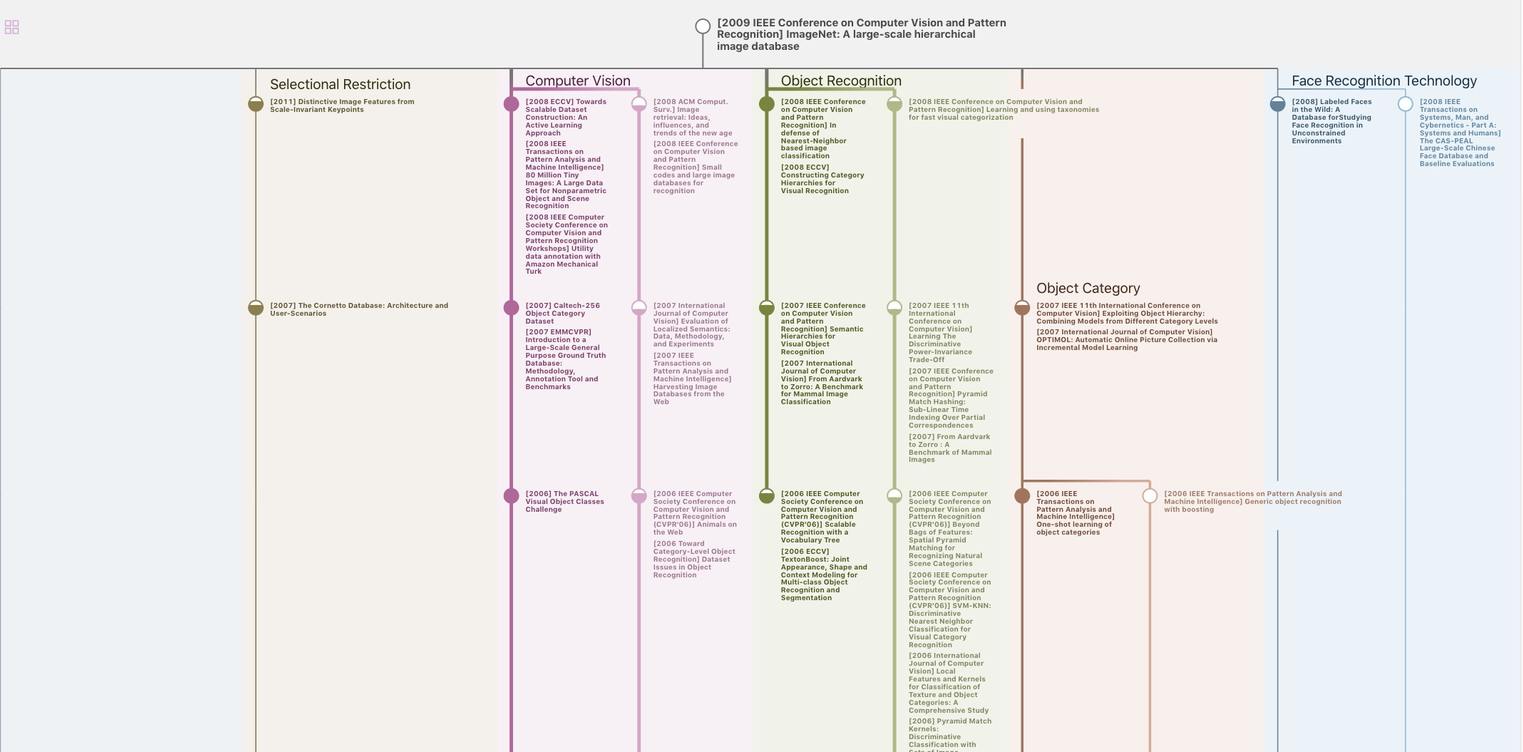
生成溯源树,研究论文发展脉络
Chat Paper
正在生成论文摘要