Traffic State Identification Based on Graph Neural Networks: Taking Tidal Traffic as an Example.
2023 IEEE 26th International Conference on Intelligent Transportation Systems (ITSC)(2023)
摘要
Traffic state identification is an important step before deploying many applications in intelligent transportation systems. Under the background of traffic big data and internet of things, traditional methods are unable to utilize heterogeneous data or deal with spatial-temporal correlations. Methods based on Graph Neural Network (GNN) are promising solutions to these issues, but are rarely used. This study applied a GNN-based model to the tidal traffic route identification. The traffic state identification was modeled as a node classification problem. A novel graph representation of road networks based on flow turns was proposed to represent heterogeneous data and tackle data missing issues. The Graph Attention Network (GAT) and Gate Recurrent Unit (GRU) were adopted to model the spatial-temporal correlations. Compromising on the difficulty of diverse real data collection, the model was trained with synthetic data and tested with real data. With tidal traffic as an example, results showed the good adaptabilities of GNN in traffic state identification, and the outperformance of proposed graph structure against two traditional representations. Overall, this study indicated the practical prospects of GNN in traffic state identification.
更多查看译文
关键词
Traffic Conditions,Graph Neural Networks,Graphical Representation,Internet Of Things,Heterogeneous Data,Road Network,Graph Structure,Network Representation,Traffic Data,Gated Recurrent Unit,Intelligent Transportation Systems,Graph Attention,Traditional Representation,Graph Attention Network,Spatial-temporal Correlation,Validation Set,Residential Areas,Time Series Data,Identical Results,Attention Mechanism,Dual Representation,Evening Peak,Graph Neural Network Model,Traffic Flow,License Plate Recognition,Peak Hours,Urban Road Network,Traffic Volume,Nodes In The Graph,Representative Methods
AI 理解论文
溯源树
样例
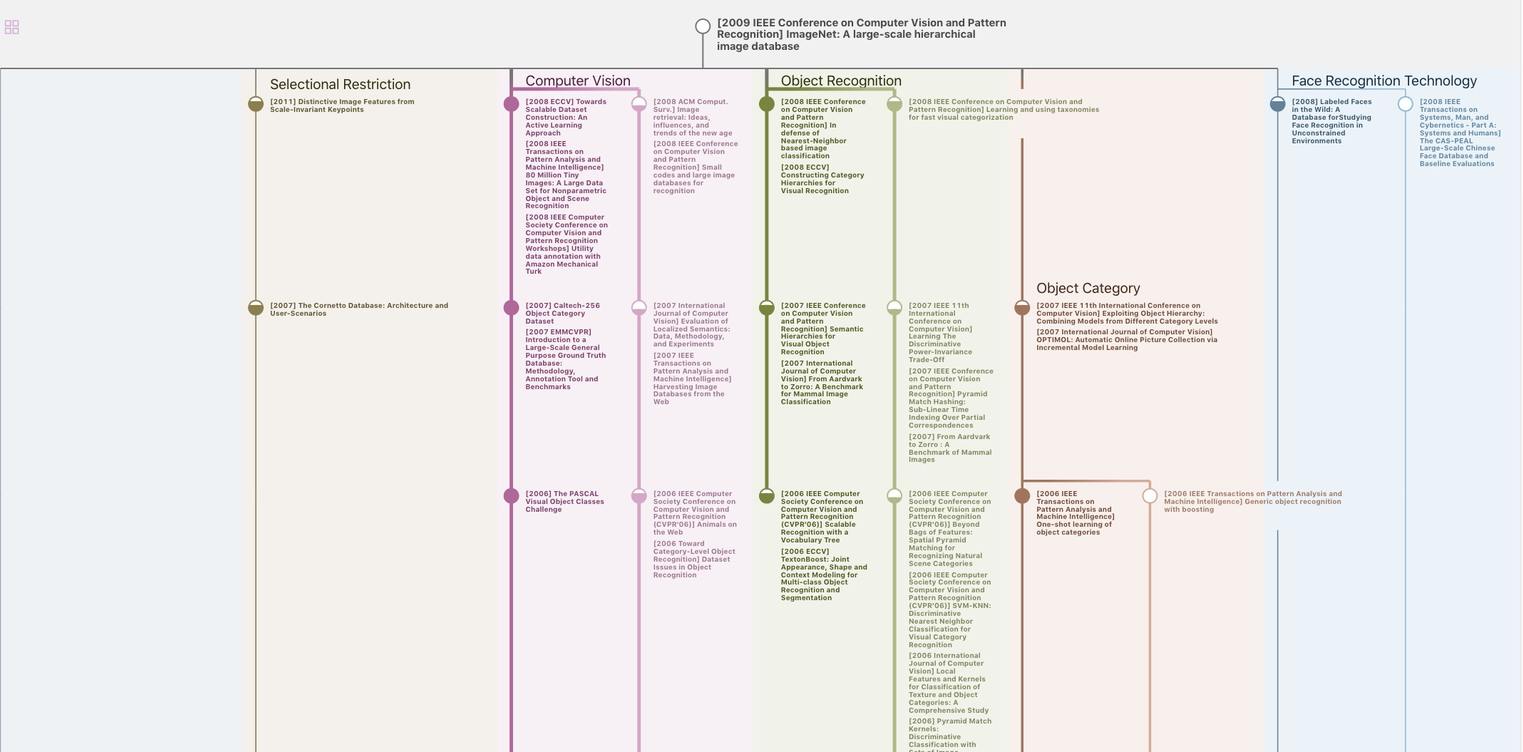
生成溯源树,研究论文发展脉络
Chat Paper
正在生成论文摘要