STMA-GCN_PedCross: Skeleton Based Spatial-Temporal Graph Convolution Networks with Multiple Attentions for Fast Pedestrian Crossing Intention Prediction.
2023 IEEE 26th International Conference on Intelligent Transportation Systems (ITSC)(2023)
摘要
Pedestrian crossing intention prediction is an important task for intelligent vehicles to enhance safety and reduce the risk of accidents. The high prediction accuracy and fast execution speed are essential requirements for this task. Existing studies on pedestrian crossing intention prediction have achieved good performance by using complex models and multiple modalities of input data. However, these approaches are limited in practical implications due to their high computational complexity and resource requirements. To address these, the paper propose the Spatial-Temporal Graph Convolution Network (GCN) with multiple attentions for fast and robust Pedestrian Crossing Intention Prediction (STMAGCN_PedCross). It utilizes easily accessible yet robust keypoints as input for predicting pedestrian crossing intention, making it both accurate and practical. To evaluate the effectiveness of the proposed model, we compared it with state-of-the-art models on a large-scale public Joint Attention in Autonomous Driving (JAAD) dataset. The results demonstrate that the STMA-GCN_PedCross model achieves comparable accuracy perfmance to the state-of-the-art models while having a higher robustness (recall rate) and requiring significantly less execution time. The ablation analysis further confirms the effectiveness of attention mechanisms in capturing spatial-temporal features from the skeleton sequence data by giving different attentions. Moreover, the analysis also reveals the significance of different keypoints in the prediction results.
更多查看译文
关键词
Convolutional Network,Graph Convolutional Network,Multiple Attention,Spatial-temporal Graph Convolutional Network,Attention Mechanism,Autonomous Vehicles,Data Modalities,Execution Speed,Recurrent Neural Network,Bounding Box,Attention Module,Spatial Attention,Temporal Dependencies,Segmentation Map,Sigmoid Activation Function,Dropout Layer,Attention Model,Channel Attention,Saliency Map,Cropped Images,Spatial Attention Module,Skeleton Data,Graph Convolutional Network Model,Hidden Representation,Attention Layer,RNN-based Models,Channel Attention Module,Walking Pattern,Convolutional Neural Network,ReLU Activation Function
AI 理解论文
溯源树
样例
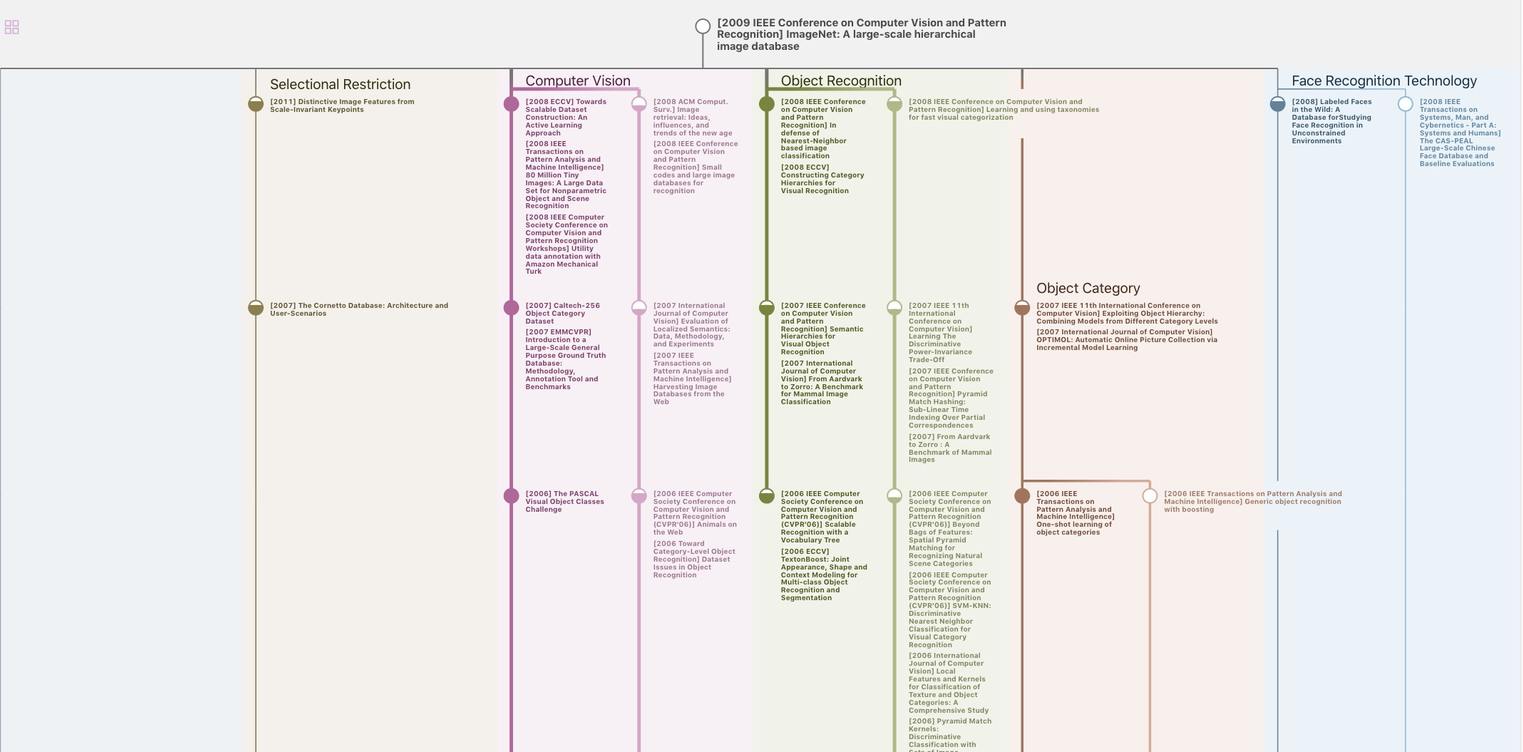
生成溯源树,研究论文发展脉络
Chat Paper
正在生成论文摘要