Flexible and Comprehensive Framework of Element Selection Based on Nonconvex Sparse Optimization
IEEE ACCESS(2024)
摘要
We propose an element selection method for high-dimensional data that is applicable to a wide range of optimization criteria in a unifying manner. Element selection is a fundamental technique for reducing dimensionality of high-dimensional data by simple operations without the use of scalar multiplication. Restorability is one of the commonly used criteria in element selection, and the element selection problem based on restorability is formulated as a minimization problem of a loss function representing the restoration error between the original data and the restored data. However, conventional methods are applicable only to a limited class of loss functions such as & ell;(2) norm loss. To enable the use of a wide variety of criteria, we reformulate the element selection problem as a nonconvex sparse optimization problem and derive the optimization algorithm based on Douglas-Rachford splitting method. The proposed algorithm is applicable to any loss function as long as its proximal operator is available, e.g., & ell;(1) norm loss and & ell;(infinity) norm loss as well as & ell;(2) norm loss. We conducted numerical experiments using artificial and real data, and their results indicate that the above loss functions are successfully minimized by the proposed algorithm.
更多查看译文
关键词
Optimization,Relaxation methods,Minimization,Signal processing,Dimensionality reduction,Sparse matrices,Indexes,element selection,sparse optimization,proximal operator,Douglas-Rachford splitting method
AI 理解论文
溯源树
样例
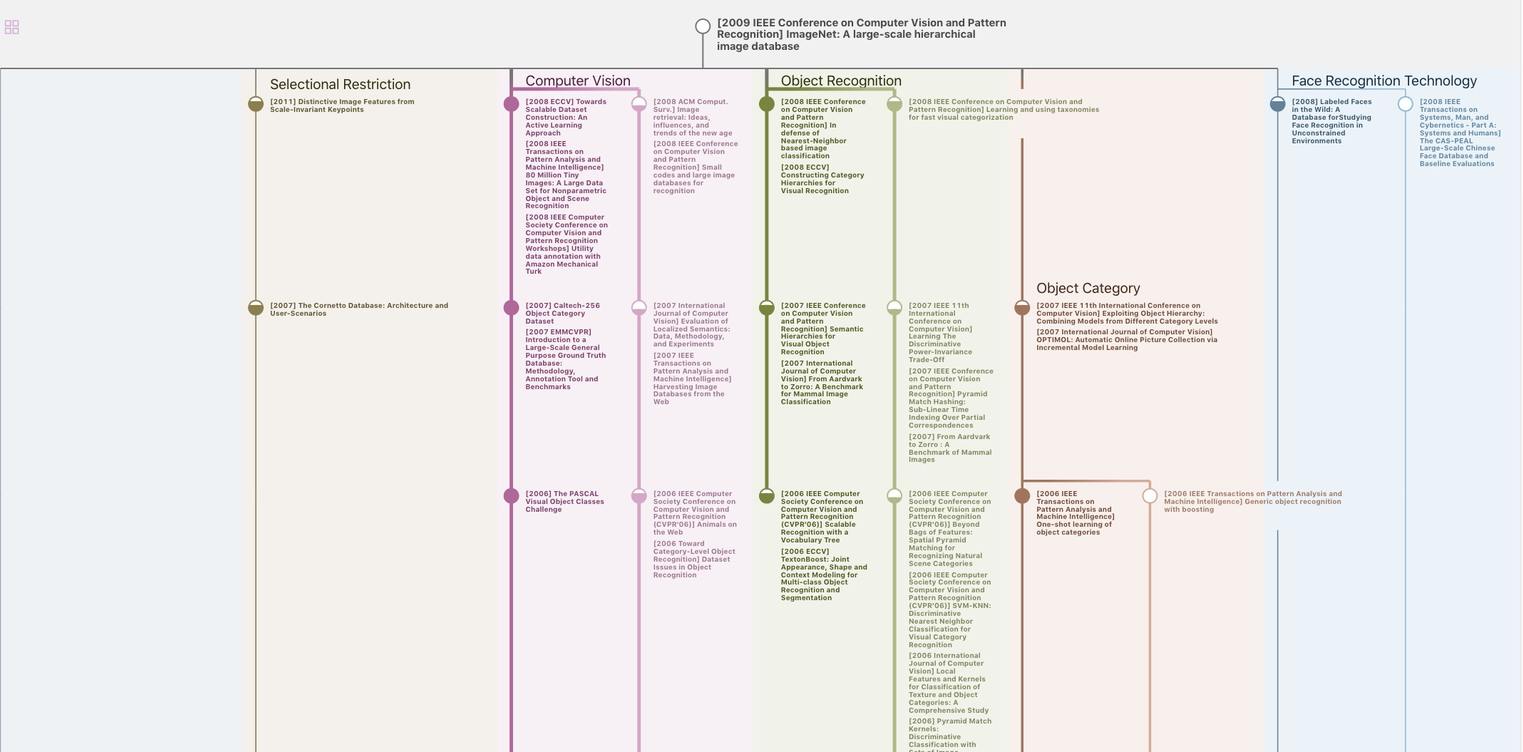
生成溯源树,研究论文发展脉络
Chat Paper
正在生成论文摘要