Sparsity via Sparse Group k-max Regularization
CoRR(2024)
摘要
For the linear inverse problem with sparsity constraints, the l_0
regularized problem is NP-hard, and existing approaches either utilize greedy
algorithms to find almost-optimal solutions or to approximate the l_0
regularization with its convex counterparts. In this paper, we propose a novel
and concise regularization, namely the sparse group k-max regularization,
which can not only simultaneously enhance the group-wise and in-group sparsity,
but also casts no additional restraints on the magnitude of variables in each
group, which is especially important for variables at different scales, so that
it approximate the l_0 norm more closely. We also establish an iterative soft
thresholding algorithm with local optimality conditions and complexity analysis
provided. Through numerical experiments on both synthetic and real-world
datasets, we verify the effectiveness and flexibility of the proposed method.
更多查看译文
AI 理解论文
溯源树
样例
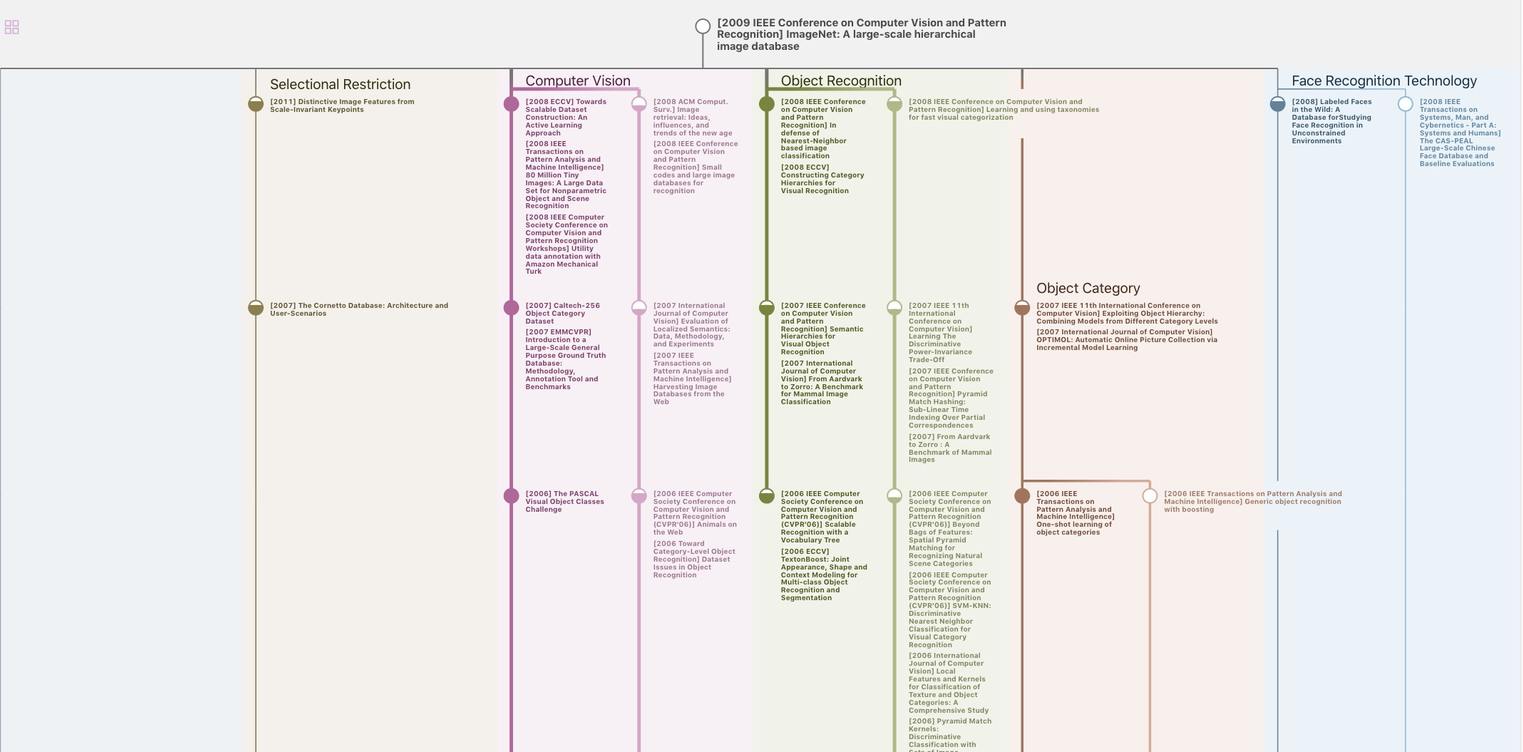
生成溯源树,研究论文发展脉络
Chat Paper
正在生成论文摘要