NfgTransformer: Equivariant Representation Learning for Normal-form Games
CoRR(2024)
摘要
Normal-form games (NFGs) are the fundamental model of strategic interaction.
We study their representation using neural networks. We describe the inherent
equivariance of NFGs – any permutation of strategies describes an equivalent
game – as well as the challenges this poses for representation learning. We
then propose the NfgTransformer architecture that leverages this equivariance,
leading to state-of-the-art performance in a range of game-theoretic tasks
including equilibrium-solving, deviation gain estimation and ranking, with a
common approach to NFG representation. We show that the resulting model is
interpretable and versatile, paving the way towards deep learning systems
capable of game-theoretic reasoning when interacting with humans and with each
other.
更多查看译文
关键词
Game Theory,Deep Learning,Representation Learning,Nash Equilibrium
AI 理解论文
溯源树
样例
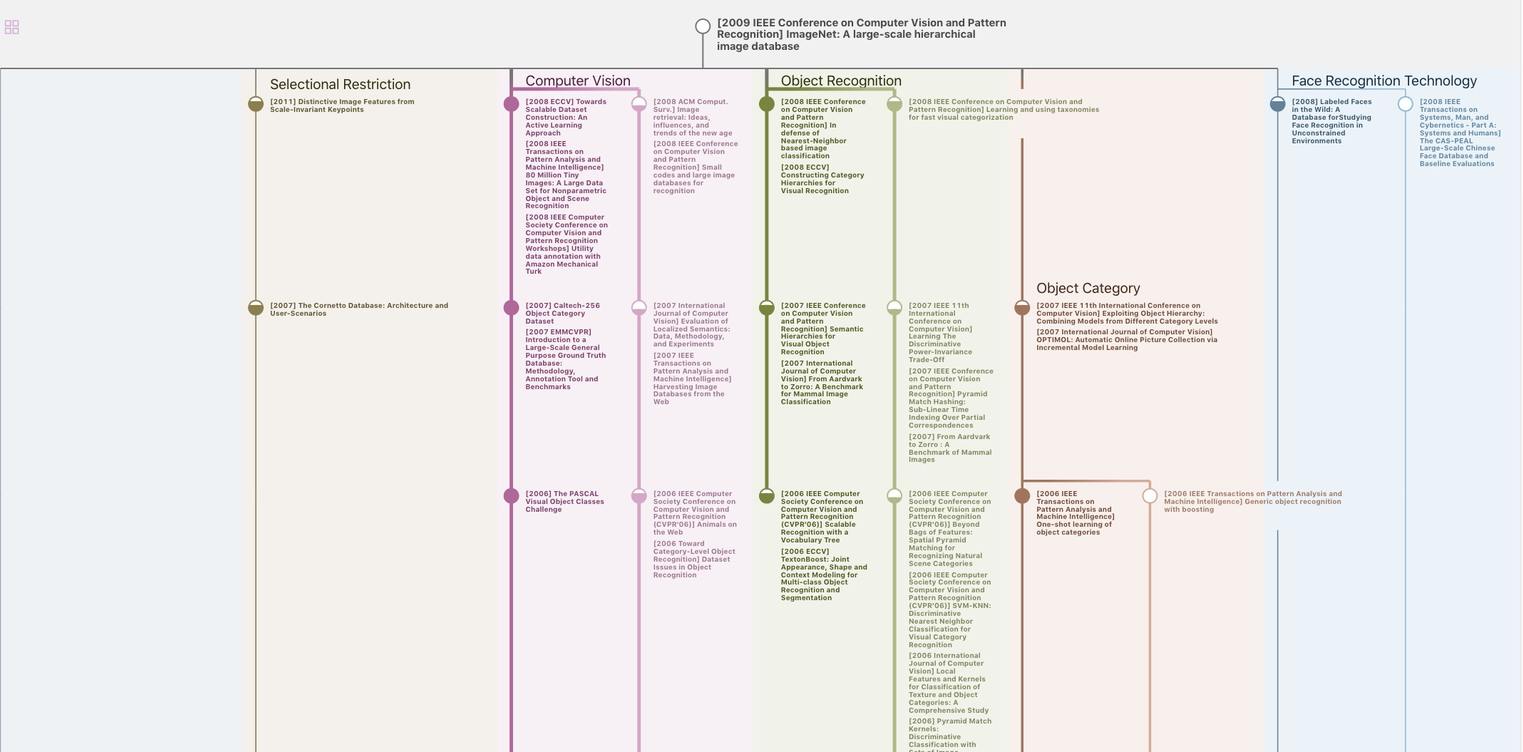
生成溯源树,研究论文发展脉络
Chat Paper
正在生成论文摘要