Image-to-image translation for satellite and UAV remote sensing: a use case for Cercospora Leaf Spot monitoring on sugar beet.
2023 IEEE International Workshop on Metrology for Agriculture and Forestry (MetroAgriFor)(2023)
摘要
In the field of vegetation remote sensing, the concept and conversion across scales has been addressed—but not concluded-in recent years. A large array of different sensors is deployed using various platforms such as uncrewed aerial vehicles (UAVs) and satellites. In this context, while multiple concepts of scale exist, the influence of spatial scale plays a major role when comparing and validating optical data derived from either of these platforms. Until to date, no satisfying method to allow cross sensor and scale studies exists. The presented work proposes a custom U-Net architecture to convert multispectral UAV imagery to multispectral satellite imagery. U-Nets are convolutional networks that have shown to be capable of learning mapping patterns between an input image and an output image. Therefore, artificially created images resembles original images at a high level of local detail and global semantic coherence. By using a multi-year, multispectral image UAV dataset, capturing a biologically and structural complex pathosystem (Cercosopora beticola + Beta vulgaris L.), it is shown that the developed custom U-Net can convert original UAV images with low structural and spectral error (MSE of 0.0001, SSIM test score of 0.0003, DWT test score of 0.0104, and a PSNR value of 40.3551) into artificial satellite images. Also, it is compared whether band center and bandwidth are accurately converted. To showcase an application, artificial images have been used to classify levels of Cercospora Leaf Spot in sugar beet. Classification accuracies are similar to those derived from the classification using original images. Therefore, it can be concluded that image to image translation using U-Nets can be a powerful tool for scaling studies in remote sensing. But they also offer to address the issue of data sparsity in machine learning as the conversion allows to generate images across different scales, therefore potentially adding valuable data to time-series studies.
更多查看译文
关键词
deep learning,drones,multispectral,plant pathology,scale
AI 理解论文
溯源树
样例
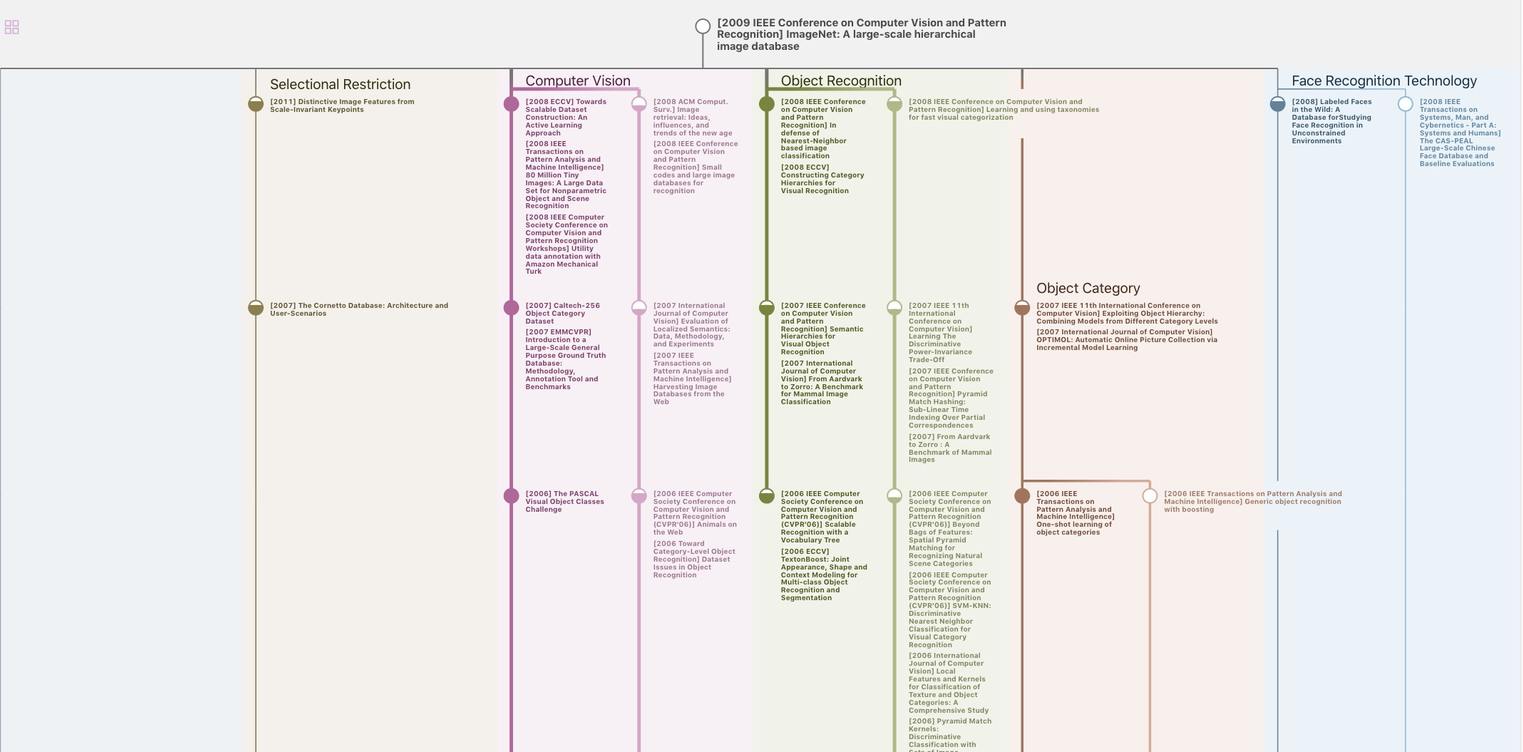
生成溯源树,研究论文发展脉络
Chat Paper
正在生成论文摘要