Artificial Intelligence Assistive Software Tool for Automated Detection and Quantification of Amyloid-Related Imaging Abnormalities
JAMA NETWORK OPEN(2024)
摘要
Importance Amyloid-related imaging abnormalities (ARIA) are brain magnetic resonance imaging (MRI) findings associated with the use of amyloid-beta-directed monoclonal antibody therapies in Alzheimer disease (AD). ARIA monitoring is important to inform treatment dosing decisions and might be improved through assistive software. Objective To assess the clinical performance of an artificial intelligence (AI)-based software tool for assisting radiological interpretation of brain MRI scans in patients monitored for ARIA. Design, Setting, and Participants This diagnostic study used a multiple-reader multiple-case design to evaluate the diagnostic performance of radiologists assisted by the software vs unassisted. The study enrolled 16 US Board of Radiology-certified radiologists to perform radiological reading with (assisted) and without the software (unassisted). The study encompassed 199 retrospective cases, where each case consisted of a predosing baseline and a postdosing follow-up MRI of patients from aducanumab clinical trials PRIME, EMERGE, and ENGAGE. Statistical analysis was performed from April to July 2023. Exposures Use of icobrain aria, an AI-based assistive software for ARIA detection and quantification. Main Outcomes and Measures Coprimary end points were the difference in diagnostic accuracy between assisted and unassisted detection of ARIA-E (edema and/or sulcal effusion) and ARIA-H (microhemorrhage and/or superficial siderosis) independently, assessed with the area under the receiver operating characteristic curve (AUC). Results Among the 199 participants included in this study of radiological reading performance, mean (SD) age was 70.4 (7.2) years; 105 (52.8%) were female; 23 (11.6%) were Asian, 1 (0.5%) was Black, 157 (78.9%) were White, and 18 (9.0%) were other or unreported race and ethnicity. Among the 16 radiological readers included, 2 were specialized neuroradiologists (12.5%), 11 were male individuals (68.8%), 7 were individuals working in academic hospitals (43.8%), and they had a mean (SD) of 9.5 (5.1) years of experience. Radiologists assisted by the software were significantly superior in detecting ARIA than unassisted radiologists, with a mean assisted AUC of 0.87 (95% CI, 0.84-0.91) for ARIA-E detection (AUC improvement of 0.05 [95% CI, 0.02-0.08]; P = .001]) and 0.83 (95% CI, 0.78-0.87) for ARIA-H detection (AUC improvement of 0.04 [95% CI, 0.02-0.07]; P = .001). Sensitivity was significantly higher in assisted reading compared with unassisted reading (87% vs 71% for ARIA-E detection; 79% vs 69% for ARIA-H detection), while specificity remained above 80% for the detection of both ARIA types. Conclusions and Relevance This diagnostic study found that radiological reading performance for ARIA detection and diagnosis was significantly better when using the AI-based assistive software. Hence, the software has the potential to be a clinically important tool to improve safety monitoring and management of patients with AD treated with amyloid-beta-directed monoclonal antibody therapies.
更多查看译文
AI 理解论文
溯源树
样例
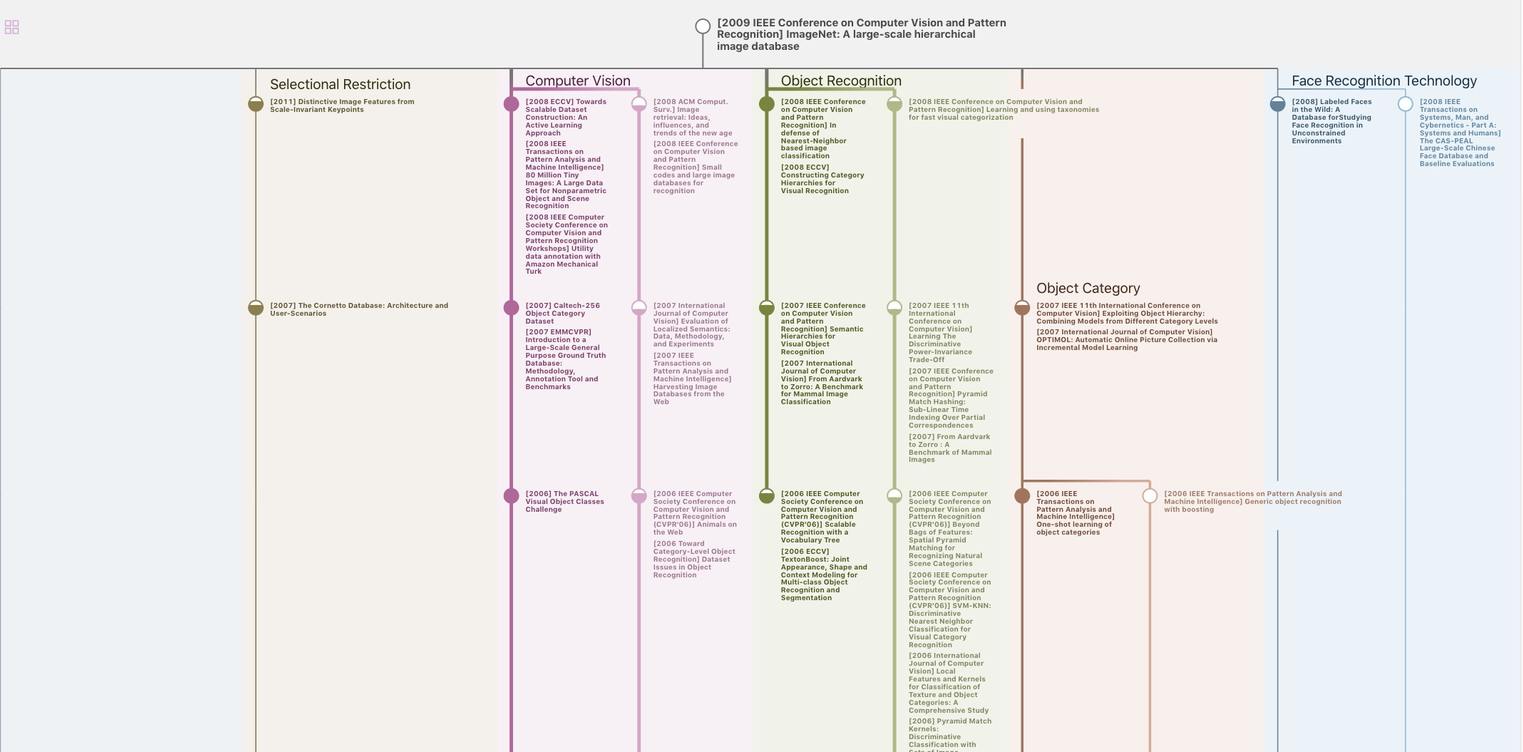
生成溯源树,研究论文发展脉络
Chat Paper
正在生成论文摘要