Cross-Domain Complementarity and Multi-Time Scale Fusion Based Resource Demand Prediction for Mobile Vehicles.
International Conference on Communication Technology(2023)
摘要
The prediction of mobile vehicle demands serves as an effective solution for addressing the communication resource scarcity caused by the expansion of vehicle-to-Everything (V2X) services, enabling resource scheduling based on future traffic flows. However, due to high mobility of vehicles and stochastic arrival of application demands, vehicular network resources exhibit variations at multiple temporal scales. Traditional resource prediction models based on a single data type often struggle to achieve accurate predictions. Therefore, the key challenge lies in leveraging the complementarity among multiple data types to achieve fusion at different temporal scales and enhance the accuracy of resource prediction models. In this paper, we propose a mobile vehicle resource demand prediction algorithm based on Deep Deterministic Policy Gradient (DDPG) and Dynamic Time Warping (DTW) algorithms, considering the diverse characteristics of multiple data types. In this algorithm, DTW is utilized to capture the variations at different temporal scales in road network traffic flow and user communication, while DDPG leverages their complementarity to improve prediction accuracy. Simulation results demonstrate that the proposed method achieves higher prediction accuracy compared to single-factor prediction methods.
更多查看译文
关键词
Cross-domain Complementarity,Multi-time Scale Fusion,Resource Prediction
AI 理解论文
溯源树
样例
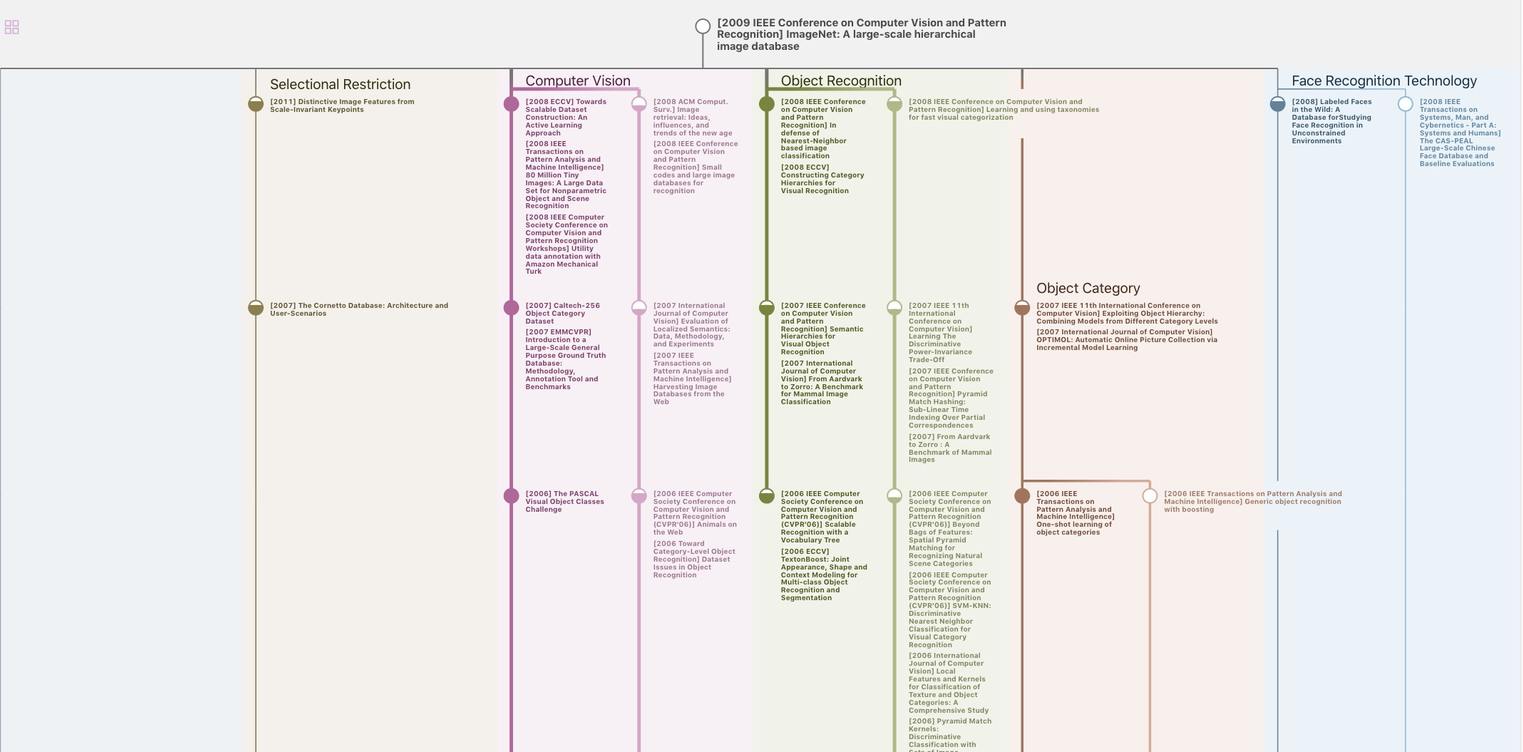
生成溯源树,研究论文发展脉络
Chat Paper
正在生成论文摘要