Joint Optimization of Multi-UAV-Assisted Data Collection and Energy Replenishment via Transfer Learning aided Deep Reinforcement Learning.
International Conference on Communication Technology(2023)
摘要
Unmanned Aerial Vehicles (UAVs) have been widely deployed in efficient data collection for the Internet of Things (IoT), UAVs can act as relays in data transmission, but also as energy sources to provide energy for low-power Sensor Nodes (SNs) on the ground. However, UAV flight is constrained by its battery capacity, so the issue of energy replenishment must be considered. This paper investigates the problem of multi-UAV assisted fresh data collection with energy replenishment in the IoT. On one hand, the SNs receive wireless energy recharges from the UAVs, and upload their sensing data to them. The UAV s fly across the SNs to collect fresh data and land at the charging stations to get energy replenishment as needed. The multi-UAV-aided data collection and energy replenishment problem can be modeled as a Partially Observable Markov Decision Process (POMDP), where each UAV acts as an intelligent agent learning the environment and independently makes decisions on its flight, SN association, and energy recharging. To find the optimal strategy, this paper combines the Value-Decomposition Network (VDN) algorithm with transfer learning to design a new Transfer-Fused VDN (TFVDN) algorithm for multi-agent scenarios. Simulation results demonstrate that the VDN algorithm exhibits significant advantages over traditional greedy algorithms. Additionally, the incorporation of transfer learning enhances the performance of TFVDN compared to VDN, while significantly accelerating the algorithm's training speed.
更多查看译文
关键词
UAV,Energy Replenishment,Deep Reinforcement Learning,Transfer Learning
AI 理解论文
溯源树
样例
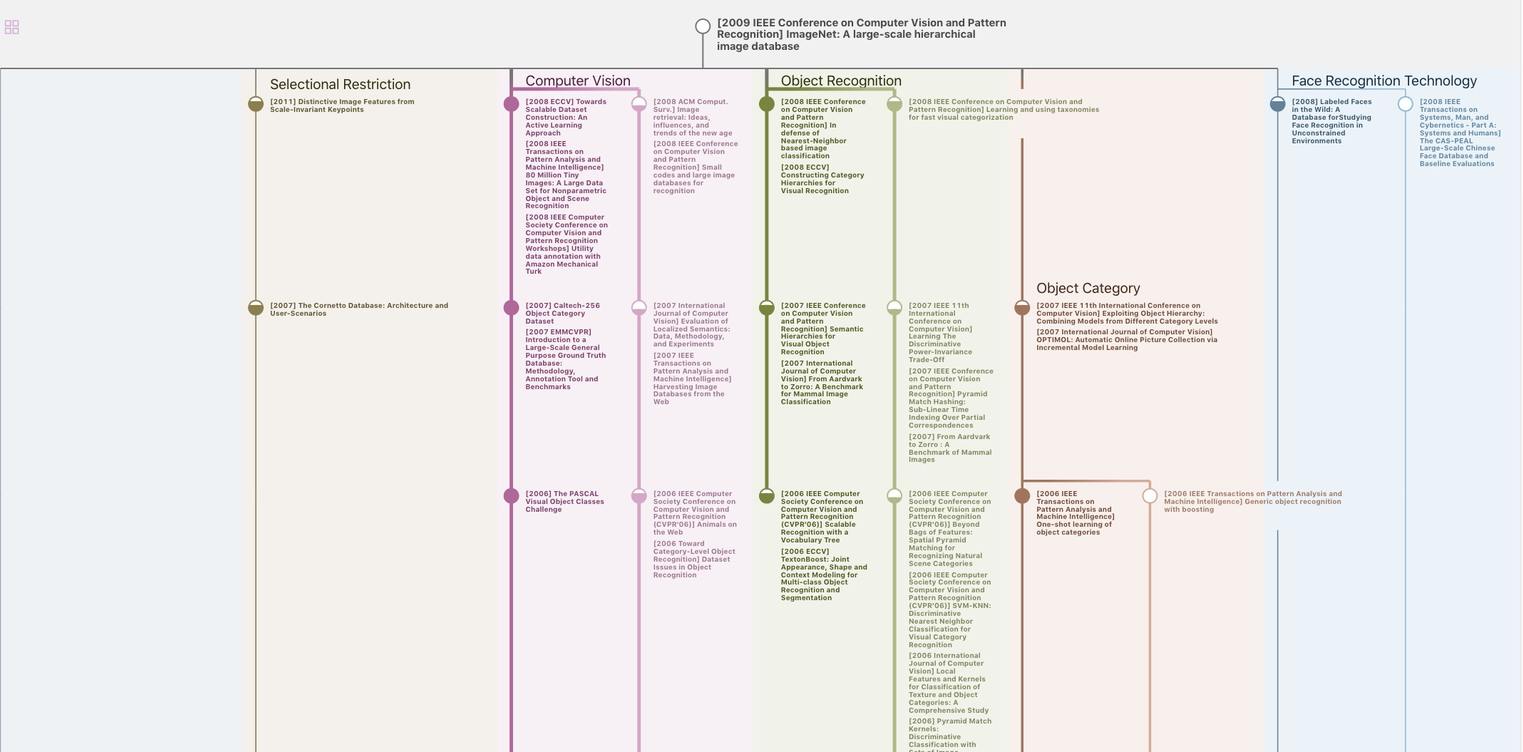
生成溯源树,研究论文发展脉络
Chat Paper
正在生成论文摘要