Design and Evaluation of Arabic Handwritten Digit Recognition System Using Biologically Plausible Methods
Arabian Journal for Science and Engineering(2024)
摘要
Automated handwritten digit identification has become essential for many daily operations such as verifying the authenticity of a cheque or deciphering a postal code. Researchers have been putting a lot of effort into figuring out how to automatically recognize and sort handwritten digits because of the advent of robotic technology in recent decades. Due to the complexity of the Arabic language and a lack of publicly available Arabic handwritten digit datasets, previous research has primarily concentrated on automating the recognition of English and European digits on availability of relevant datasets. Arabic handwritten recognition also plays a significant role in electronic-learning (e-learning) systems. It has not taken into account the recognition of handwritten Arabic digits. Arabic handwritten digits vary in size, form, slant, and image noise, which can cause changes in numeral topology. These factors make it difficult to classify and recognize Arabic handwritten digits. In order to overcome these challenges, we implemented a biologically plausible technique to classify Arabic handwritten digits. The objective of the research is to address the unique challenges of style, size, shape, slant variations, and image noise of Arabic handwritten digits in classification and recognition. To categorize Arabic handwritten digits, we employed convolutional spiking neural network (CSNN) and spike neural network (SNN) models. The reason for using SNN and SCNN is that the second-generation neural networks accumulate accolades on various computer vision tasks, i.e., pattern recognition, segmentation, and classification. However, there are certain challenges, i.e., energy inefficiency and computational cost, presented by these networks. The spiking neural network models also address these issues. These models are low-power, high-performance neural network models. We trained the spiking neural network model on Arabic digit datasets using a rate-based, non-spiking algorithm, i.e., backpropagation and soft-LIF, then integrated them with a spiking neural network. We have also used an STDP (spike time-dependent plasticity)-based spiking neural network for Arabic digit recognition. We attenuate the spiking variability in CSNN by adding noise during training, compensating the training errors, and bringing robustness against the spiking variability. We experimented with soft-LIF, a non-spiking version of the LIF neuron, and a variant of STDP rules for Arabic digit classification. Experimental results illustrate that CSNN achieves high performance at a significantly low energy and computational cost. We attained 98.98% and 91.16% recognition rates for the Arabic digit dataBase (ADBase) dataset using the convolutional spiking model and STDP spiking model, respectively.
更多查看译文
关键词
Spiking neural networks,Soft-LIF,STDP,Pattern recognition,Segmentation,Classification
AI 理解论文
溯源树
样例
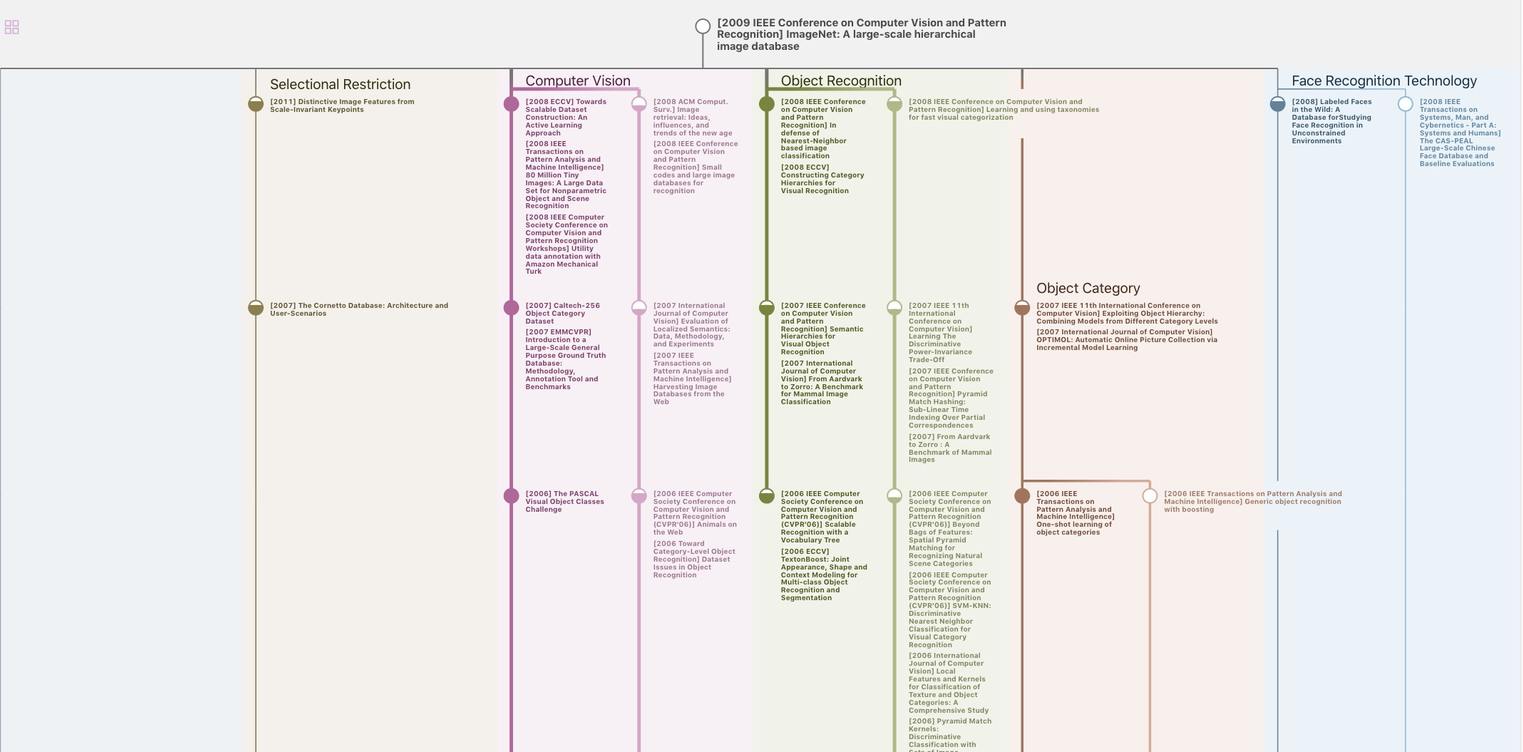
生成溯源树,研究论文发展脉络
Chat Paper
正在生成论文摘要