UVTM: Universal Vehicle Trajectory Modeling with ST Feature Domain Generation
arxiv(2024)
摘要
Vehicle movement is frequently captured in the form of trajectories, i.e.,
sequences of timestamped locations. Numerous methods exist that target
different tasks involving trajectories such as travel-time estimation,
trajectory recovery, and trajectory prediction. However, most methods target
only one specific task and cannot be applied universally. Existing efforts to
create a universal trajectory model often involve adding prediction modules for
adapting to different tasks, while also struggle with incomplete or sparse
trajectories.
To address these shortcomings, we propose the Universal Vehicle Trajectory
Model (UVTM) designed to support different tasks based on incomplete or sparse
trajectories without the need for retraining or extra prediction modules. To
addresses task adaptability on incomplete trajectories, UVTM divide the
spatio-temporal features of trajectories into three distinct domains. Each
domain can be masked and generated independently to suit the input and output
needs of specific tasks. To handle sparse trajectories effectively, UVTM is
pre-trained by reconstructing densely sampled trajectories from sparsely
sampled ones, allowing it to extract detailed spatio-temporal information from
sparse trajectories. Experiments involving three representative
trajectory-related tasks on two real-world vehicle trajectory datasets provide
insight into the intended properties performance of UVTM and offer evidence
that UVTM is capable of meeting its objectives.
更多查看译文
AI 理解论文
溯源树
样例
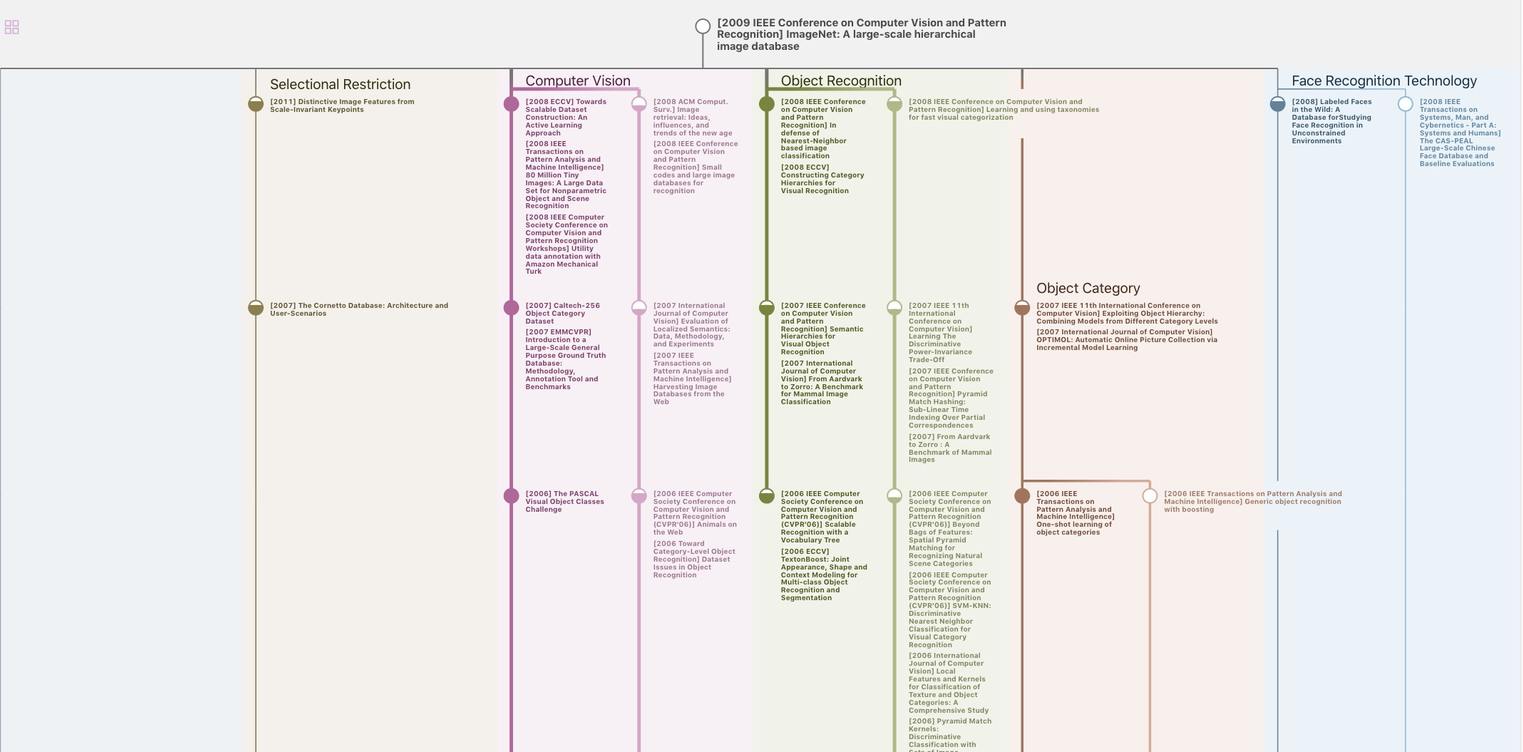
生成溯源树,研究论文发展脉络
Chat Paper
正在生成论文摘要