Convergence of Gradient Descent with Small Initialization for Unregularized Matrix Completion
CoRR(2024)
摘要
We study the problem of symmetric matrix completion, where the goal is to
reconstruct a positive semidefinite matrix X^⋆∈ℝ^d× d of rank-r, parameterized by UU^⊤,
from only a subset of its observed entries. We show that the vanilla gradient
descent (GD) with small initialization provably converges to the ground truth
X^⋆ without requiring any explicit regularization. This convergence
result holds true even in the over-parameterized scenario, where the true rank
r is unknown and conservatively over-estimated by a search rank r'≫ r.
The existing results for this problem either require explicit regularization, a
sufficiently accurate initial point, or exact knowledge of the true rank r.
In the over-parameterized regime where r'≥ r, we show that, with
Ω(dr^9) observations, GD with an initial point U_0≤ϵ converges near-linearly to an ϵ-neighborhood of
X^⋆. Consequently, smaller initial points result in increasingly
accurate solutions. Surprisingly, neither the convergence rate nor the final
accuracy depends on the over-parameterized search rank r', and they are only
governed by the true rank r. In the exactly-parameterized regime where
r'=r, we further enhance this result by proving that GD converges at a faster
rate to achieve an arbitrarily small accuracy ϵ>0, provided the
initial point satisfies U_0 = O(1/d). At the crux of our method lies
a novel weakly-coupled leave-one-out analysis, which allows us to establish the
global convergence of GD, extending beyond what was previously possible using
the classical leave-one-out analysis.
更多查看译文
AI 理解论文
溯源树
样例
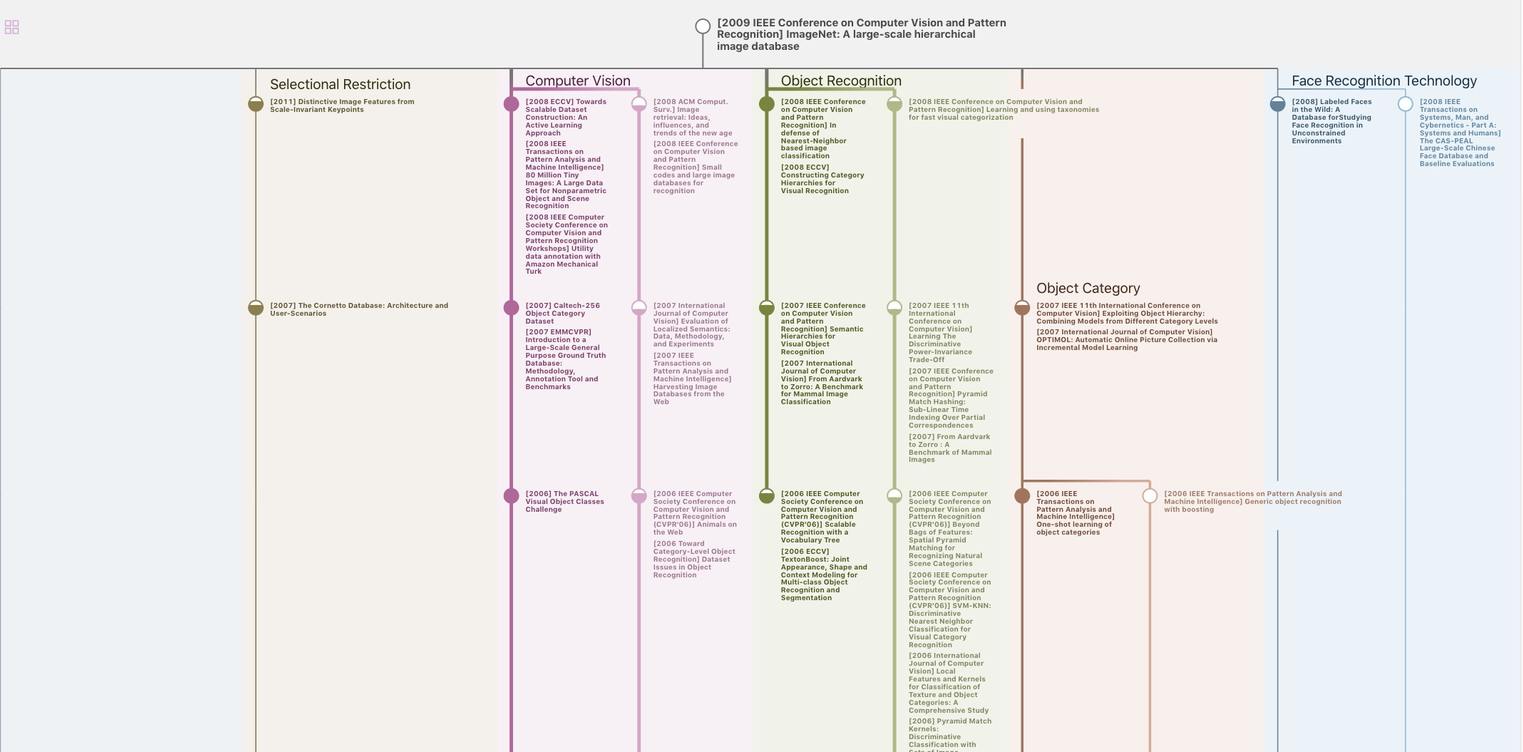
生成溯源树,研究论文发展脉络
Chat Paper
正在生成论文摘要