Computational analysis of a class of singular nonlinear fractional multi-order heat conduction model of the human head
Scientific Reports(2024)
摘要
The subject of the article is devoted to the development of a matrix collocation technique based upon the combination of the fractional-order shifted Vieta–Lucas functions (FSVLFs) and the quasilinearization method (QLM) for the numerical evaluation of the fractional multi-order heat conduction model related to the human head with singularity and nonlinearity. The fractional operators are adopted in accordance with the Liouville–Caputo derivative. The quasilinearization method (QLM) is first utilized in order to defeat the inherent nonlinearity of the problem, which is converted to a family of linearized subequations. Afterward, we use the FSVLFs along with a set of collocation nodes as the zeros of these functions to reach a linear algebraic system of equations at each iteration. In the weighted L_2 norm, the convergence analysis of the FSVLFs series solution is established. We especially assert that the expansion series form of FSVLFs is convergent in the infinity norm with order 𝒪(1/K^3) , where K represents the number of FSVLFs used in approximating the unknown solution. Diverse computational experiments by running the presented combined QLM-FSVLFs are conducted using various fractional orders and nonlinearity parameters. The outcomes indicate that the QLM-FSVLFs produces efficient approximate solutions to the underlying model with high-order accuracy, especially near the singular point. Furthermore, the methodology of residual error functions is employed to measure the accuracy of the proposed hybrid algorithm. Comparisons with existing numerical models show the superiority of QLM-FSVLFs, which also is straightforward in implementation.
更多查看译文
关键词
Collocation points,Convergence analysis,Human head,Heat conduction,Liouville–Caputo fractional derivative,Shifted Vieta–Lucas functions,Singular ODEs,Strongly nonlinearity
AI 理解论文
溯源树
样例
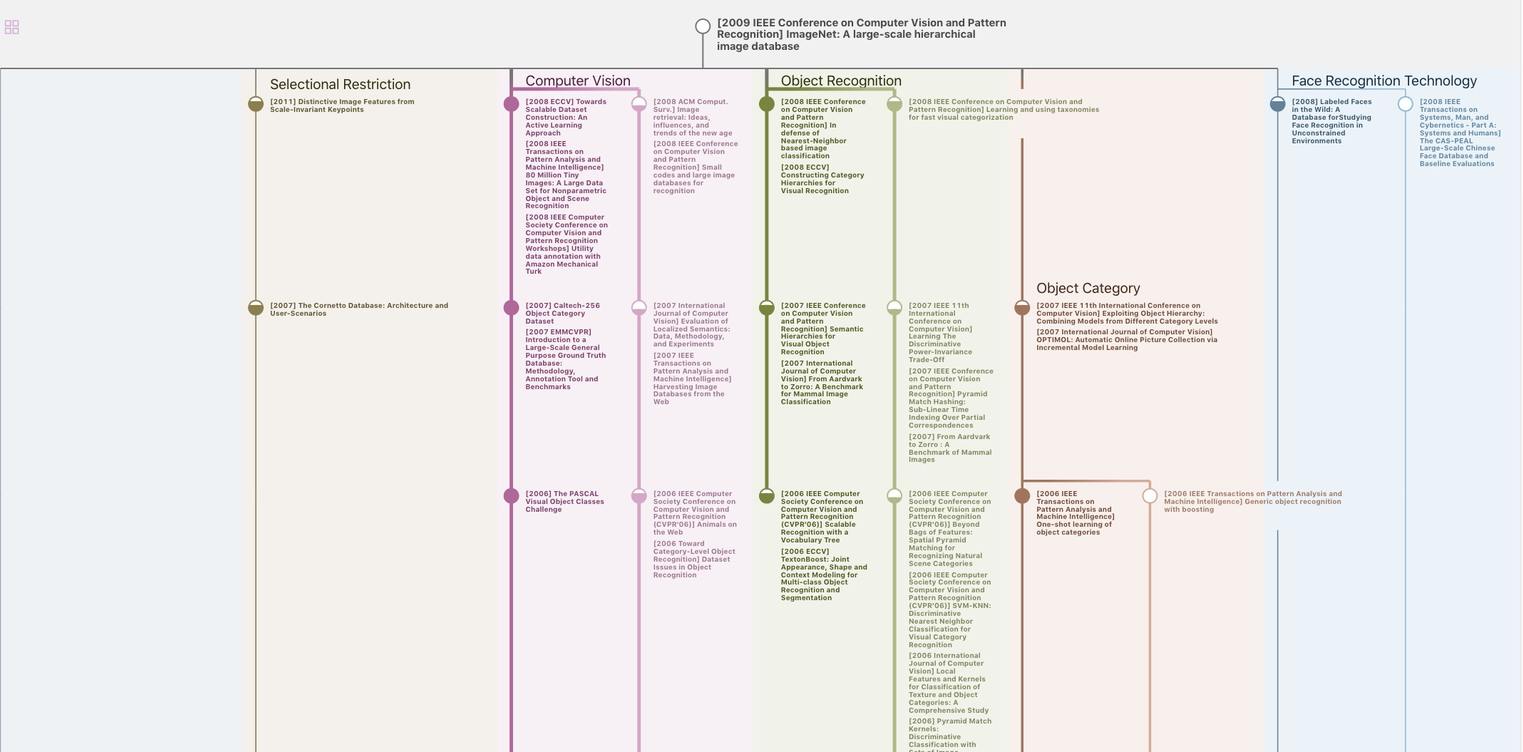
生成溯源树,研究论文发展脉络
Chat Paper
正在生成论文摘要