Algorithm-hardware co-design for Energy-Efficient A/D conversion in ReRAM-based accelerators
CoRR(2024)
摘要
Deep neural networks are widely deployed in many fields. Due to the in-situ
computation (known as processing in memory) capacity of the Resistive Random
Access Memory (ReRAM) crossbar, ReRAM-based accelerator shows potential in
accelerating DNN with low power and high performance. However, despite power
advantage, such kind of accelerators suffer from the high power consumption of
peripheral circuits, especially Analog-to-Digital Converter (ADC), which
account for over 60 percent of total power consumption. This problem hinders
the ReRAM-based accelerator to achieve higher efficiency.
Some redundant Analog-to-Digital conversion operations have no contribution
to maintaining inference accuracy, and such operations can be eliminated by
modifying the ADC searching logic. Based on such observations, we propose an
algorithm-hardware co-design method and explore the co-design approach in both
hardware design and quantization algorithms. Firstly, we focus on the
distribution output along the crossbar's bit-lines and identify the
fine-grained redundant ADC sampling bits.
ADC bits, we propose a hardware-friendly quantization method and coding scheme,
in which different quantization strategy was applied to the partial results in
different intervals. To support the two features above, we propose a
lightweight architectural design based on SAR-ADC. It's worth mentioning that
our method is not only more energy efficient but also retains the flexibility
of the algorithm. Experiments demonstrate that our method can reduce about 1.6
∼ 2.3 × ADC power reduction.
更多查看译文
AI 理解论文
溯源树
样例
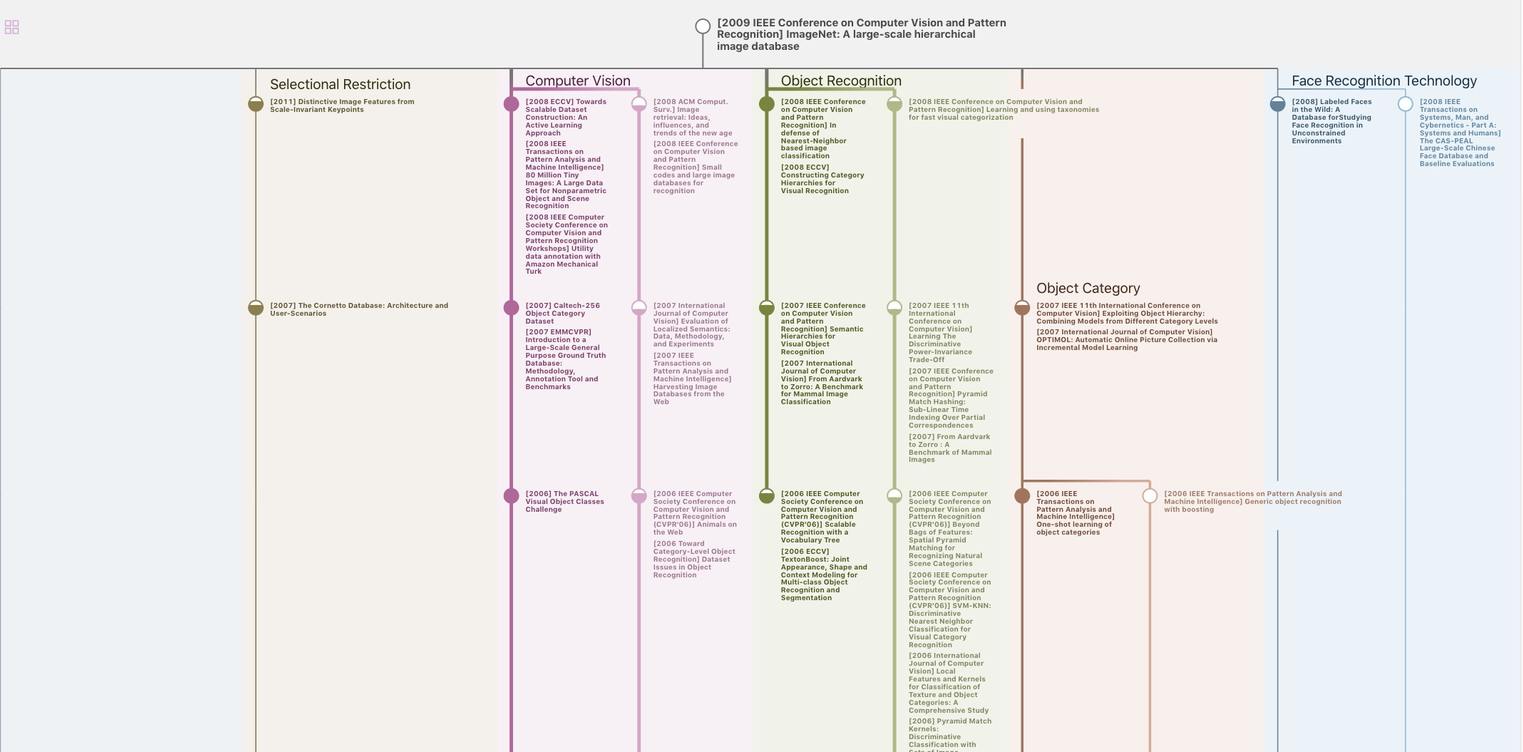
生成溯源树,研究论文发展脉络
Chat Paper
正在生成论文摘要