Impact on Public Health Decision Making by Utilizing Big Data Without Domain Knowledge
CoRR(2024)
摘要
New data sources, and artificial intelligence (AI) methods to extract
information from them are becoming plentiful, and relevant to decision making
in many societal applications. An important example is street view imagery,
available in over 100 countries, and considered for applications such as
assessing built environment aspects in relation to community health outcomes.
Relevant to such uses, important examples of bias in the use of AI are evident
when decision-making based on data fails to account for the robustness of the
data, or predictions are based on spurious correlations. To study this risk, we
utilize 2.02 million GSV images along with health, demographic, and
socioeconomic data from New York City. Initially, we demonstrate that built
environment characteristics inferred from GSV labels at the intra-city level
may exhibit inadequate alignment with the ground truth. We also find that the
average individual-level behavior of physical inactivity significantly mediates
the impact of built environment features by census tract, as measured through
GSV. Finally, using a causal framework which accounts for these mediators of
environmental impacts on health, we find that altering 10
two lowest tertiles would result in a 4.17 (95
CI 14.4 to 21.3) times bigger decrease on the prevalence of obesity or
diabetes, than the same proportional intervention on the number of crosswalks
by census tract. This work illustrates important issues of robustness and model
specification for informing effective allocation of interventions using new
data sources.
更多查看译文
AI 理解论文
溯源树
样例
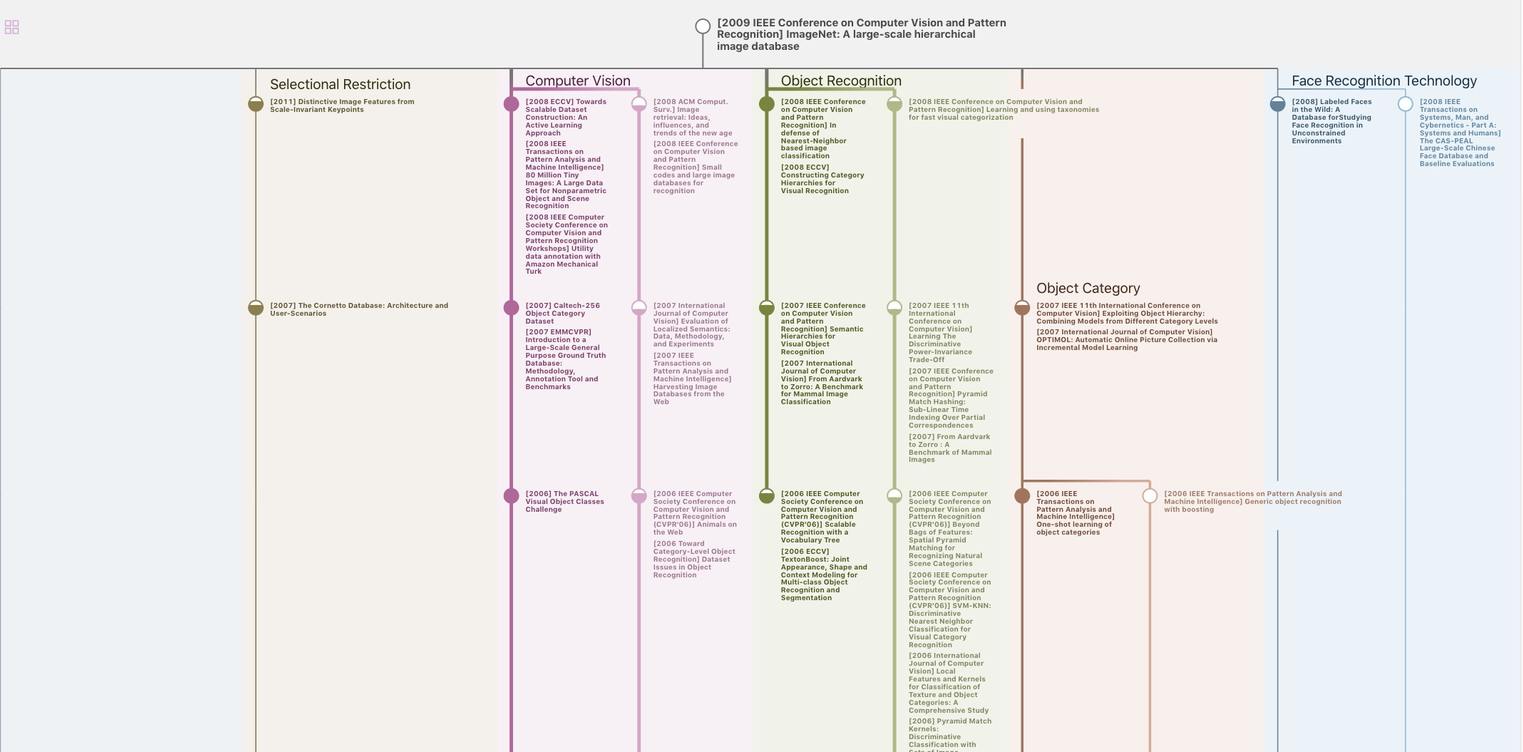
生成溯源树,研究论文发展脉络
Chat Paper
正在生成论文摘要