NPSVC++: Nonparallel Classifiers Encounter Representation Learning
CoRR(2024)
摘要
This paper focuses on a specific family of classifiers called nonparallel
support vector classifiers (NPSVCs). Different from typical classifiers, the
training of an NPSVC involves the minimization of multiple objectives,
resulting in the potential concerns of feature suboptimality and class
dependency. Consequently, no effective learning scheme has been established to
improve NPSVCs' performance through representation learning, especially deep
learning. To break this bottleneck, we develop NPSVC++ based on multi-objective
optimization, enabling the end-to-end learning of NPSVC and its features. By
pursuing Pareto optimality, NPSVC++ theoretically ensures feature optimality
across classes, hence effectively overcoming the two issues above. A general
learning procedure via duality optimization is proposed, based on which we
provide two applicable instances, K-NPSVC++ and D-NPSVC++. The experiments show
their superiority over the existing methods and verify the efficacy of NPSVC++.
更多查看译文
AI 理解论文
溯源树
样例
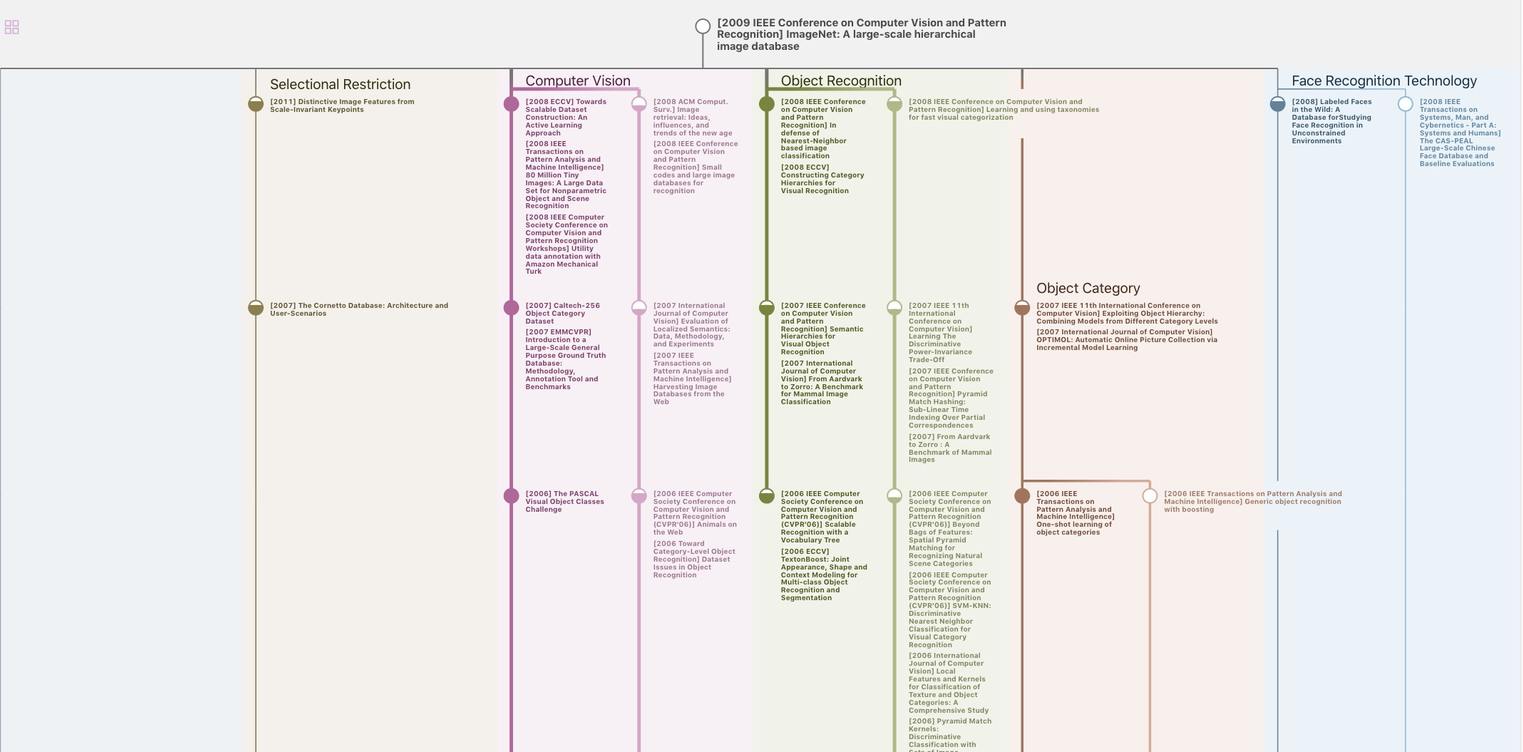
生成溯源树,研究论文发展脉络
Chat Paper
正在生成论文摘要