Global Model Selection for Semi-Supervised Support Vector Machine via Solution Paths.
IEEE transactions on neural networks and learning systems(2024)
摘要
Semi-supervised support vector machine (S 3 VM) is important because it can use plentiful unlabeled data to improve the generalization accuracy of traditional SVMs. In order to achieve good performance, it is necessary for S 3 VM to take some effective measures to select hyperparameters. However, model selection for semi-supervised models is still a key open problem. Existing methods for semi-supervised models to search for the optimal parameter values are usually computationally demanding, especially those ones with grid search. To address this challenging problem, in this article, we first propose solution paths of S 3 VM (SPS 3 VM), which can track the solutions of the nonconvex S 3 VM with respect to the hyperparameters. Specifically, we apply incremental and decremental learning methods to update the solution and let it satisfy the Karush-Kuhn-Tucker (KKT) conditions. Based on the SPS 3 VM and the piecewise linearity of model function, we can find the model with the minimum cross-validation (CV) error for the entire range of candidate hyperparameters by computing the error path of S 3 VM. Our SPS 3 VM is the first solution path algorithm for nonconvex optimization problem of semi-supervised learning models. We also provide the finite convergence analysis and computational complexity of SPS 3 VM. Experimental results on a variety of benchmark datasets not only verify that our SPS 3 VM can globally search the hyperparameters (regularization and ramp loss parameters) but also show a huge reduction of computational time while retaining similar or slightly better generalization performance compared with the grid search approach.
更多查看译文
关键词
Cross validation (CV),error path,semi-supervised support vector machine (S $^3$ VM),solution path
AI 理解论文
溯源树
样例
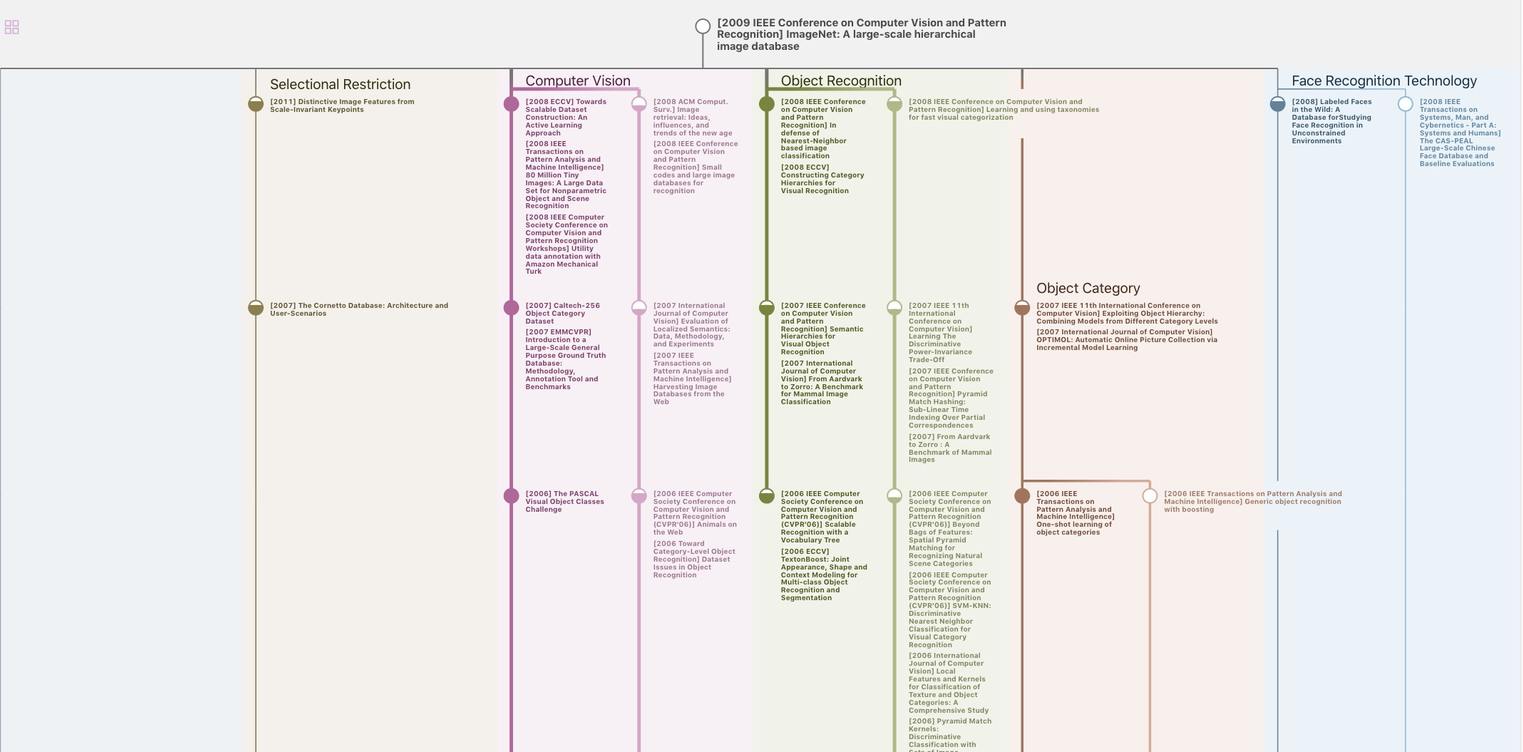
生成溯源树,研究论文发展脉络
Chat Paper
正在生成论文摘要