LFSMIM: A Low-Frequency Spectral Masked Image Modeling Method for Hyperspectral Image Classification.
IEEE Geoscience and Remote Sensing Letters(2024)
摘要
Masked image modeling (MIM) has made significant advancements across various fields in recent years. Previous research in the hyperspectral (HS) domain often utilizes conventional Transformers to model spectral sequences, overlooking the impact of local details on HS image classification. Furthermore, training models using raw image features as reconstruction targets entail significant challenges. In this study, we specifically focus on the reconstruction targets and feature modeling capabilities of the Vision Transformer (ViT) to address the limitations of MIM methods in the HS domain. As a proposed solution, we introduce a novel and effective method called LFSMIM, which incorporates two key strategies: 1) filtering out high-frequency components from the reconstruction target to mitigate the network’s sensitivity to noise and 2) enhancing the local and global modeling capabilities of the ViT to effectively capture weakened texture details and exploit global spectral features. LFSMIM demonstrated superior performance in overall accuracy (OA) compared to other methods on the Indian Pines (IP), Pavia University (PU), and Houston 2013 (HT) datasets, achieving accuracies of 95.522%, 98.820%, and 98.160% respectively. The code will be made available at
https://github.com/yuweikong/LFSMIM
.
更多查看译文
关键词
Hyperspectral image (HSI),masked image modeling (MIM),self-supervised learning,vision transformer (ViT)
AI 理解论文
溯源树
样例
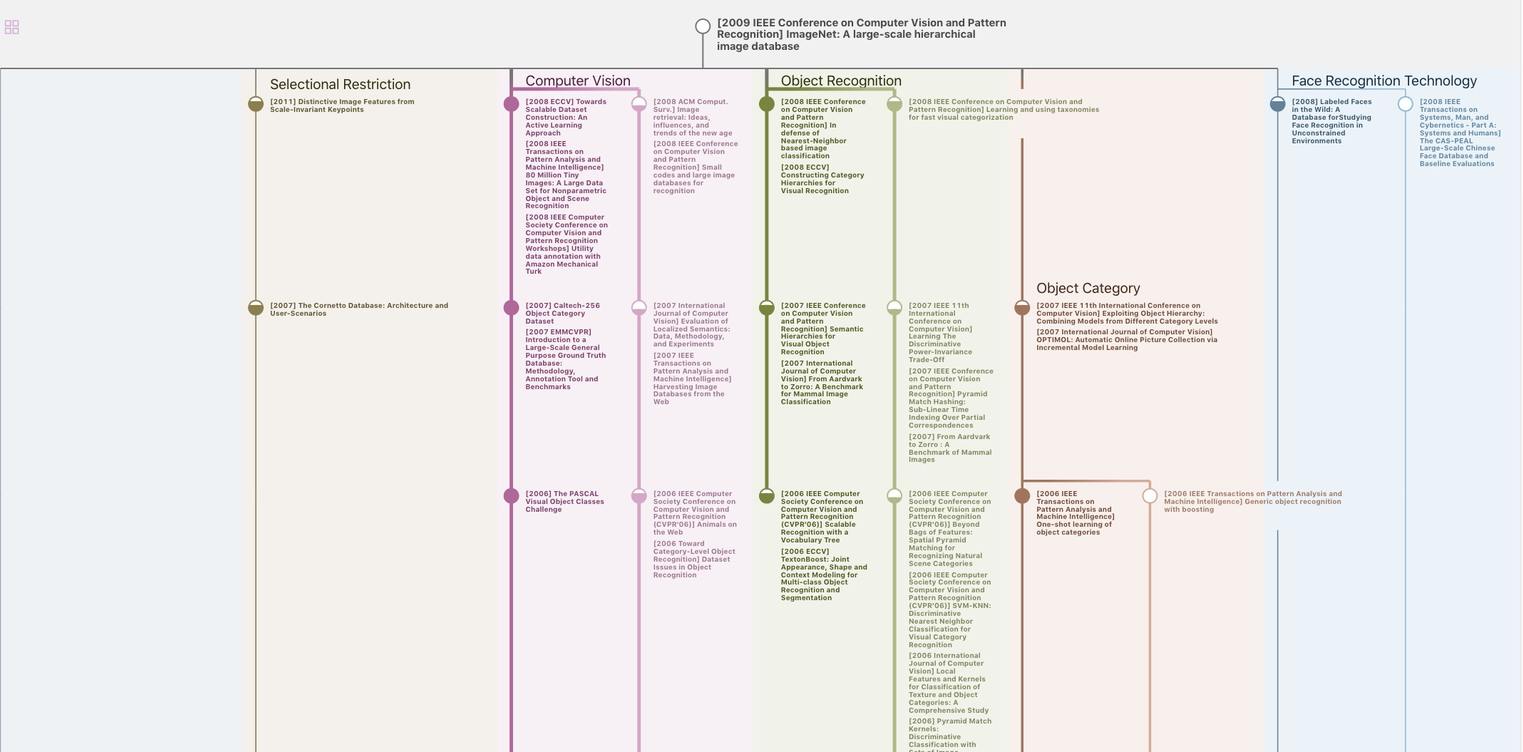
生成溯源树,研究论文发展脉络
Chat Paper
正在生成论文摘要