Digital design of a spatial-pow-STDP learning block with high accuracy utilizing pow CORDIC for large-scale image classifier spatiotemporal SNN
Scientific Reports(2024)
摘要
The paramount concern of highly accurate energy-efficient computing in machines with significant cognitive capabilities aims to enhance the accuracy and efficiency of bio-inspired Spiking Neural Networks (SNNs). This paper addresses this main objective by introducing a novel spatial power spike-timing-dependent plasticity (Spatial-Pow-STDP) learning rule as a digital block with high accuracy in a bio-inspired SNN model. Motivated by the demand for precise and accelerated computation that reduces high-cost resources in neural network applications, this paper presents a methodology based on COordinate Rotation DIgital Computer (CORDIC) definitions. The proposed designs of CORDIC algorithms for exponential (Exp CORDIC), natural logarithm (Ln CORDIC), and arbitrary power function (Pow CORDIC) are meticulously detailed and evaluated to ensure optimal acceleration and accuracy, which respectively show average errors near 10 –9 , 10 –6 , and 10 –5 with 4, 4, and 6 iterations. The engineered architectures for the Exp, Ln, and Pow CORDIC implementations are illustrated and assessed, showcasing the efficiency achieved through high frequency, leading to the introduction of a Spatial-Pow-STDP learning block design based on Pow CORDIC that facilitates efficient and accurate hardware computation with 6.93 × 10 –3 average error with 9 iterations. The proposed learning mechanism integrates this structure into a large-scale spatiotemporal SNN consisting of three layers with reduced hyper-parameters, enabling unsupervised training in an event-based paradigm using excitatory and inhibitory synapses. As a result, the application of the developed methodology and equations in the computational SNN model for image classification reveals superior accuracy and convergence speed compared to existing spiking networks by achieving up to 97.5%, 97.6%, 93.4%, and 93% accuracy, respectively, when trained on the MNIST, EMNIST digits, EMNIST letters, and CIFAR10 datasets with 6, 2, 2, and 6 training epochs.
更多查看译文
关键词
Bio-Inspired SNN,AMPA and GABA neurotransmitters,LIF neurons,Image classification,Spatial-Pow-STDP,Unsupervised learning,Exp CORDIC,Ln CORDIC,Pow CORDIC,FPGA implementation
AI 理解论文
溯源树
样例
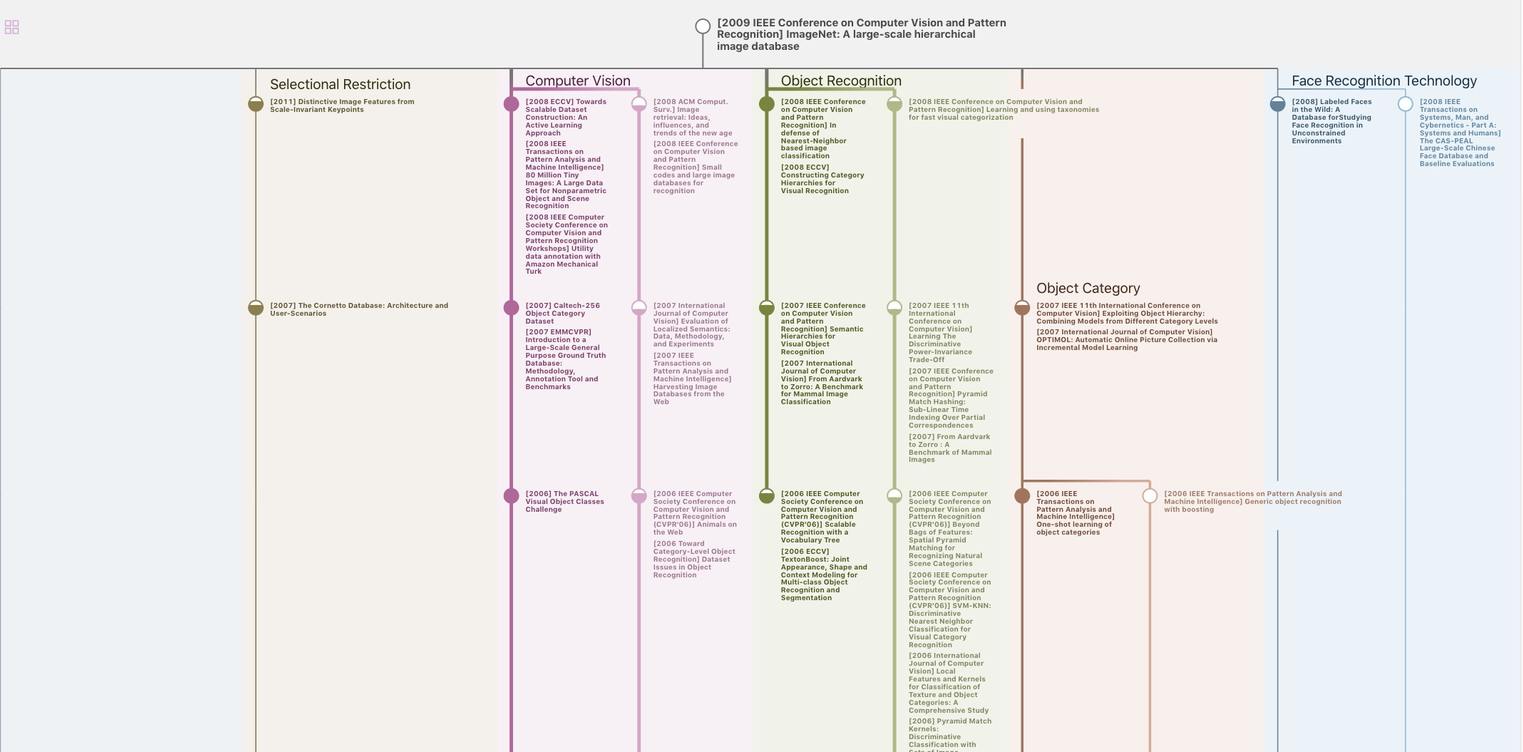
生成溯源树,研究论文发展脉络
Chat Paper
正在生成论文摘要