Spatial relation categorization in infants and deep neural networks
COGNITION(2024)
摘要
Spatial relations, such as above, below, between, and containment, are important mediators in children's understanding of the world (Piaget, 1954). The development of these relational categories in infancy has been extensively studied (Quinn, 2003) yet little is known about their computational underpinnings. Using developmental tests, we examine the extent to which deep neural networks, pretrained on a standard vision benchmark or egocentric video captured from one baby's perspective, form categorical representations for visual stimuli depicting relations. Notably, the networks did not receive any explicit training on relations. We then analyze whether these networks recover similar patterns to ones identified in development, such as reproducing the relative difficulty of categorizing different spatial relations and different stimulus abstractions. We find that the networks we evaluate tend to recover many of the patterns observed with the simpler relations of "above versus below"or "between versus outside", but struggle to match developmental findings related to "containment". We identify factors in the choice of model architecture, pretraining data, and experimental design that contribute to the extent the networks match developmental patterns, and highlight experimental predictions made by our modeling results. Our results open the door to modeling infants' earliest categorization abilities with modern machine learning tools and demonstrate the utility and productivity of this approach.
更多查看译文
关键词
Spatial relation categorization,Cognitive development,Neural Networks,Connectionist models,Pretrained computer vision models
AI 理解论文
溯源树
样例
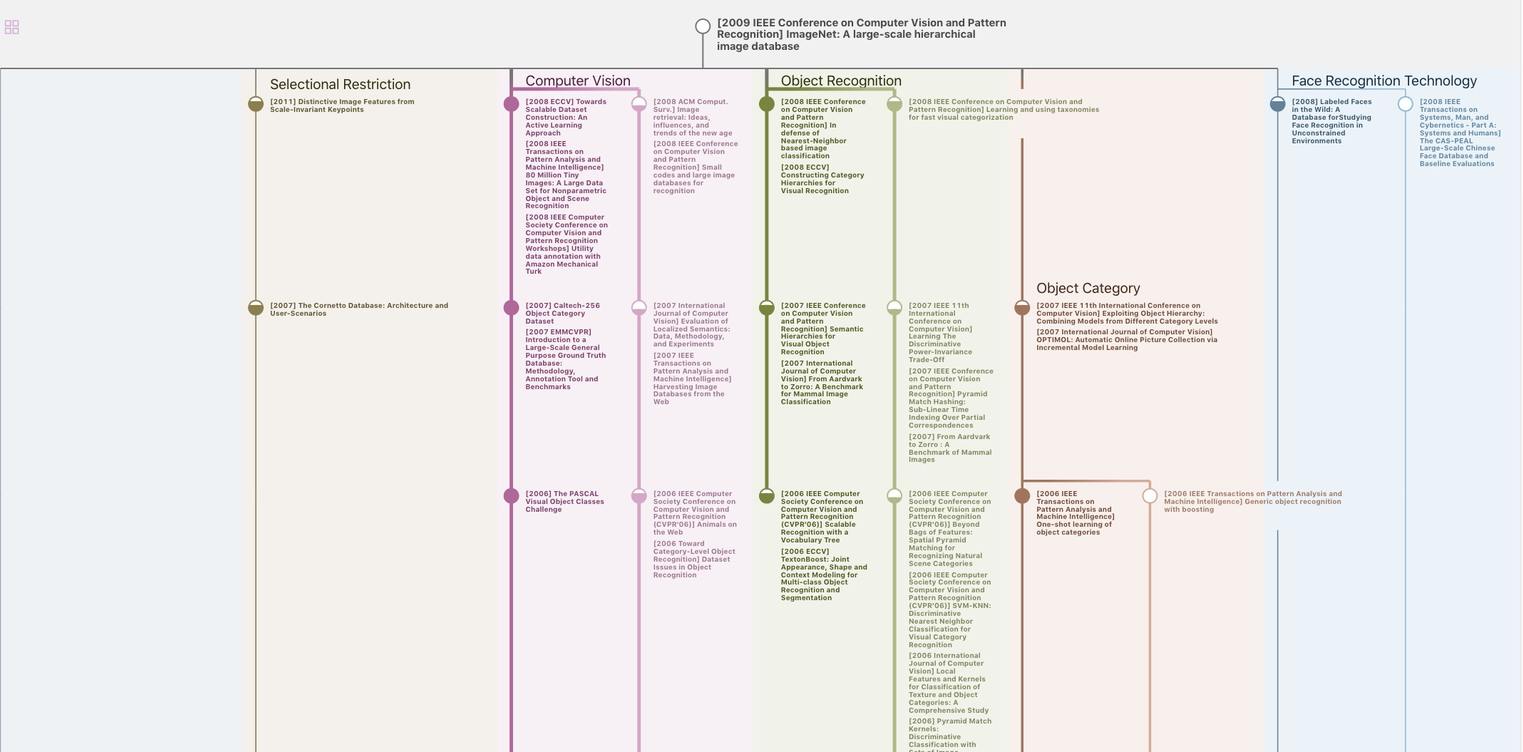
生成溯源树,研究论文发展脉络
Chat Paper
正在生成论文摘要