Hardware Implementations of a Deep Learning Approach to Optimal Configuration of Reconfigurable Intelligence Surfaces
SENSORS(2024)
摘要
Reconfigurable intelligent surfaces (RIS) offer the potential to customize the radio propagation environment for wireless networks, and will be a key element for 6G communications. However, due to the unique constraints in these systems, the optimization problems associated to RIS configuration are challenging to solve. This paper illustrates a new approach to the RIS configuration problem, based on the use of artificial intelligence (AI) and deep learning (DL) algorithms. Concretely, a custom convolutional neural network (CNN) intended for edge computing is presented, and implementations on different representative edge devices are compared, including the use of commercial AI-oriented devices and a field-programmable gate array (FPGA) platform. This FPGA option provides the best performance, with x20 performance increase over the closest FP32, GPU-accelerated option, and almost x3 performance advantage when compared with the INT8-quantized, TPU-accelerated implementation. More noticeably, this is achieved even when high-level synthesis (HLS) tools are used and no custom accelerators are developed. At the same time, the inherent reconfigurability of FPGAs opens a new field for their use as enabler hardware in RIS applications.
更多查看译文
关键词
6G,reconfigurable intelligent surfaces,artificial intelligence,neural networks,FPGA
AI 理解论文
溯源树
样例
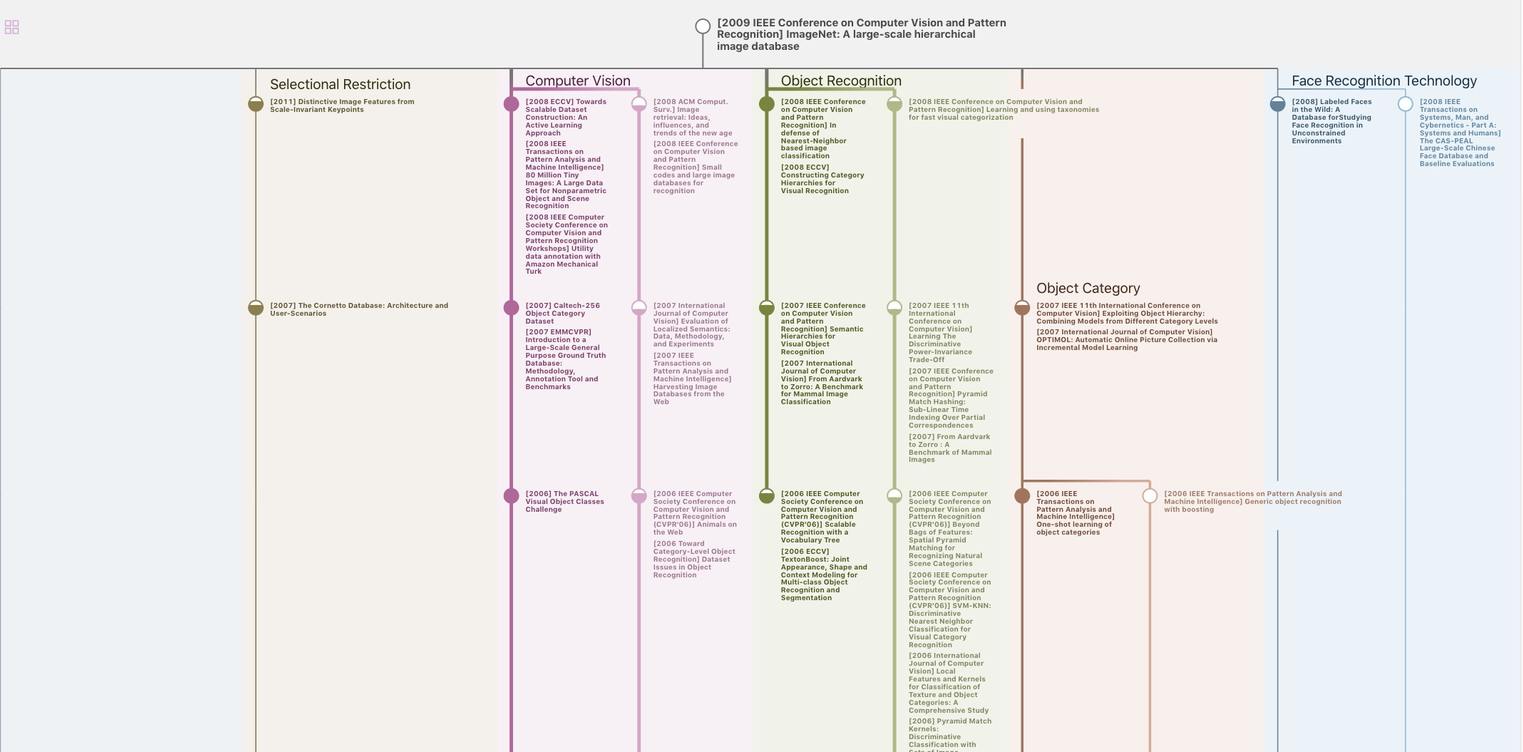
生成溯源树,研究论文发展脉络
Chat Paper
正在生成论文摘要