An Improved Nested U-Net Network for Fluorescence In Situ Hybridization Cell Image Segmentation
SENSORS(2024)
摘要
Fluorescence in situ hybridization (FISH) is a powerful cytogenetic method used to precisely detect and localize nucleic acid sequences. This technique is proving to be an invaluable tool in medical diagnostics and has made significant contributions to biology and the life sciences. However, the number of cells is large and the nucleic acid sequences are disorganized in the FISH images taken using the microscope. Processing and analyzing images is a time-consuming and laborious task for researchers, as it can easily tire the human eyes and lead to errors in judgment. In recent years, deep learning has made significant progress in the field of medical imaging, especially the successful application of introducing the attention mechanism. The attention mechanism, as a key component of deep learning, improves the understanding and interpretation of medical images by giving different weights to different regions of the image, enabling the model to focus more on important features. To address the challenges in FISH image analysis, we combined medical imaging with deep learning to develop the SEAM-Unet++ automated cell contour segmentation algorithm with integrated attention mechanism. The significant advantage of this algorithm is that it improves the accuracy of cell contours in FISH images. Experiments have demonstrated that by introducing the attention mechanism, our method is able to segment cells that are adherent to each other more efficiently.
更多查看译文
关键词
FISH images,cell contours,segmentation,Unet plus plus,attention
AI 理解论文
溯源树
样例
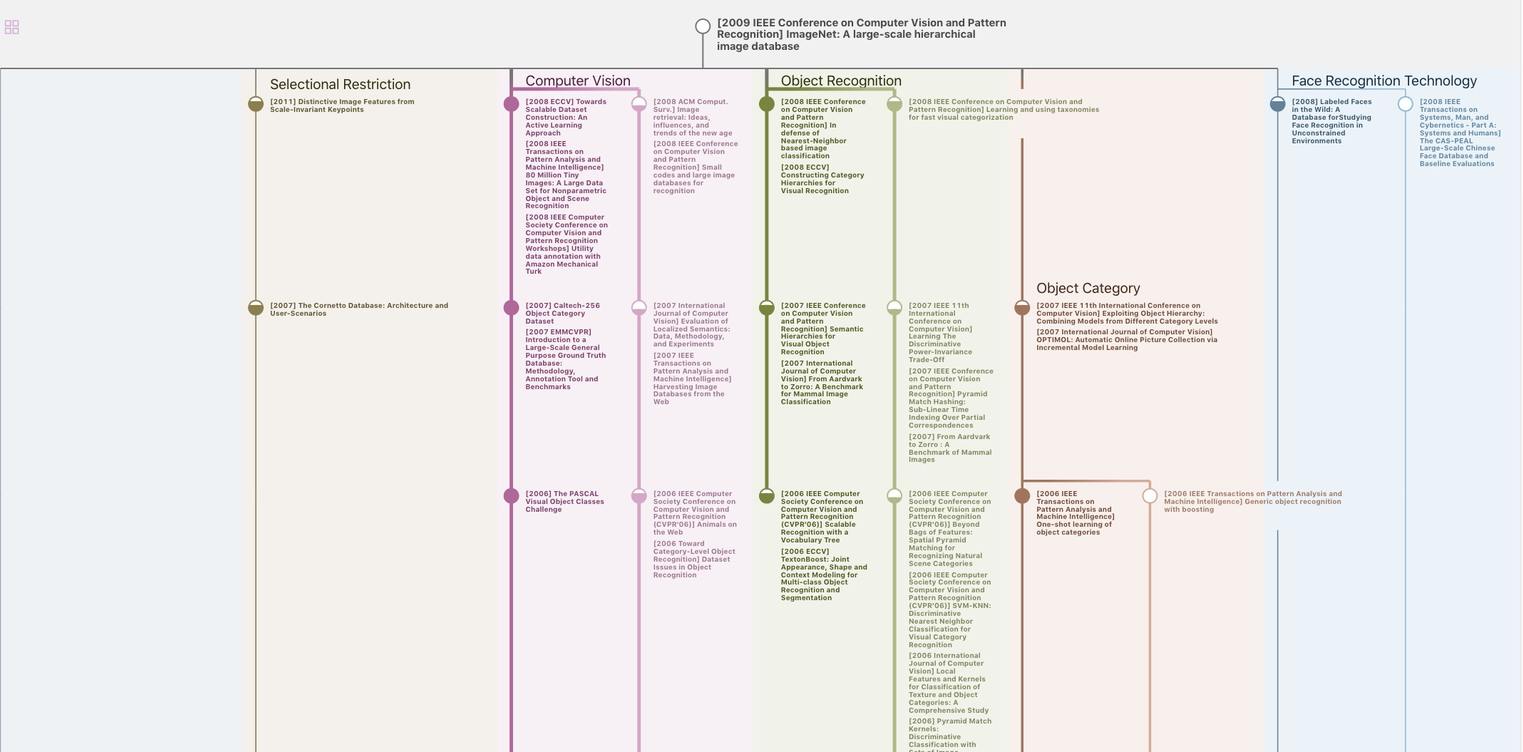
生成溯源树,研究论文发展脉络
Chat Paper
正在生成论文摘要