Model-Based Decomposition Feature Learning With Adversarial Prior
IEEE GEOSCIENCE AND REMOTE SENSING LETTERS(2024)
摘要
Model-based target decomposition (TD) method has been widely applied due to its clear physical scattering significance. However, after establishing decomposition basis, the process of solving the scattering components and parameters is usually underdetermined, which will lead to issues such as component negative power and overestimation. For this problem, this letter examines the TD task from the perspective of deep learning and proposes an adversarial decomposition feature learning (ADFL) model. This model could learn decomposition features suitable for current terrain characteristics according to input data. At the same time, the model-based adversarial feature prior is embedded in ADFL to maintain the physical scattering meanings. On real polarimetric synthetic aperture radar (PolSAR) datasets, the learned features of the proposed model are well correlated with real terrain scattering characteristics. Furthermore, it avoids negative decomposition features and makes more accurate fitting of scattering components, effectively alleviating the above problems.
更多查看译文
关键词
Scattering,Representation learning,Matrix decomposition,Image restoration,Decoding,Feature extraction,Mathematical models,Adversarial decomposition feature learning (ADFL),adversarial prior,polarimetric synthetic aperture radar (PolSAR) image,target decomposition (TD)
AI 理解论文
溯源树
样例
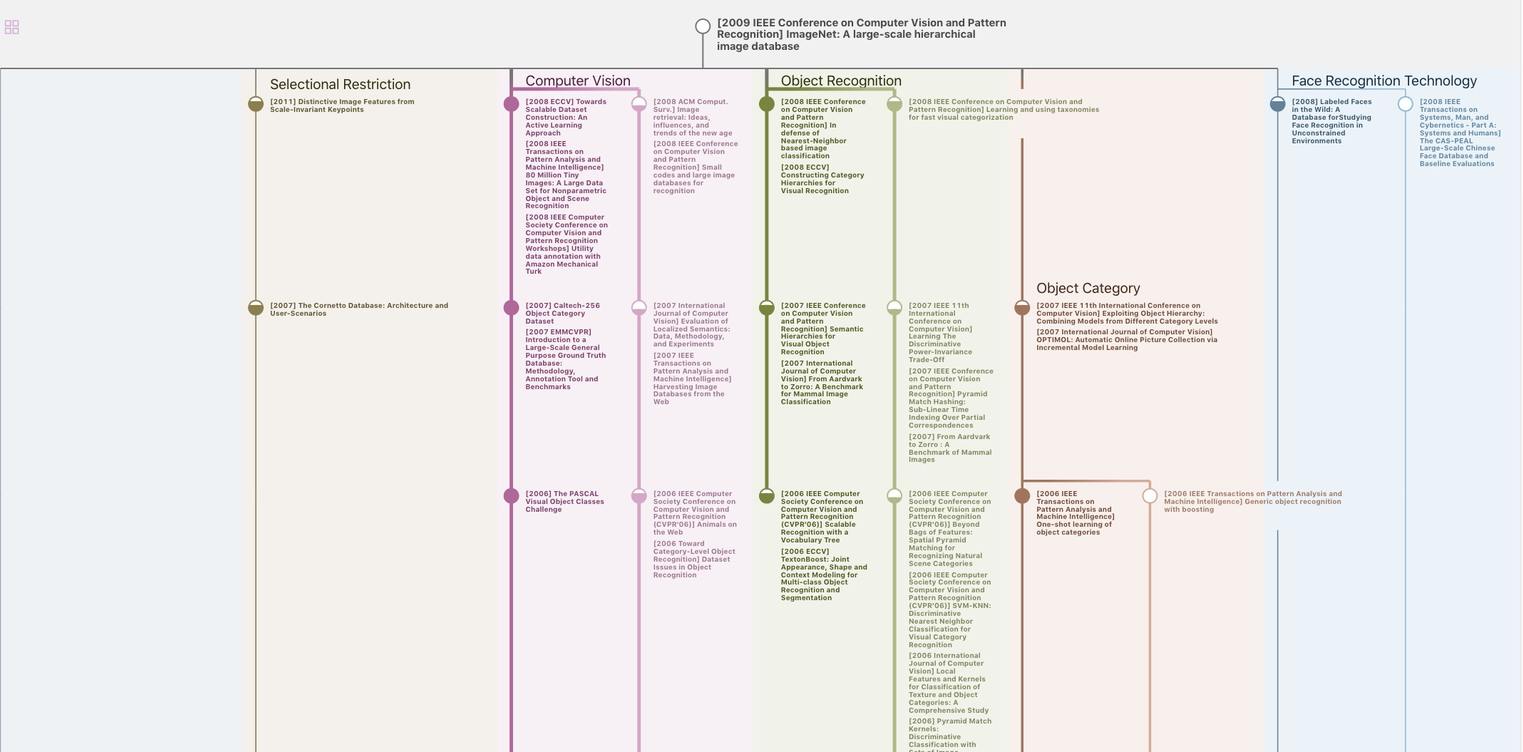
生成溯源树,研究论文发展脉络
Chat Paper
正在生成论文摘要