SwinTFNet: Dual-Stream Transformer With Cross Attention Fusion for Land Cover Classification
IEEE GEOSCIENCE AND REMOTE SENSING LETTERS(2024)
摘要
Land cover classification (LCC) is an important application in remote sensing data interpretation. As two common data sources, synthetic aperture radar (SAR) images can be regarded as an effective complement to optical images, which will reduce the influence caused by single-modal data. However, common LCC methods focus on designing advanced network architectures to process single-modal remote sensing data. Few works have been oriented toward improving segmentation performance through fusing multimodal data. In order to deeply integrate SAR and optical features, we propose SwinTFNet, a dual-stream deep fusion network. Through the global context modeling capability of Transformer structure, SwinTFNet models teleconnections between pixels in other regions and pixels in cloud regions for better prediction in cloud regions. In addition, a cross-attention fusion module (CAFM) is proposed to fuse features from optical and SAR data. Experimental results show that our method improves greatly in the classification of clouded images compared with other excellent segmentation methods and achieves the best performance on multimodal data. The source code of SwinTFNet is publicly available at https://github.com/XD-MG/SwinTFNet.
更多查看译文
关键词
Optical imaging,Feature extraction,Optical sensors,Transformers,Adaptive optics,Optical fiber networks,Fuses,Data fusion,land cover classification (LCC),multimodality,synthetic aperture radar (SAR)-optical
AI 理解论文
溯源树
样例
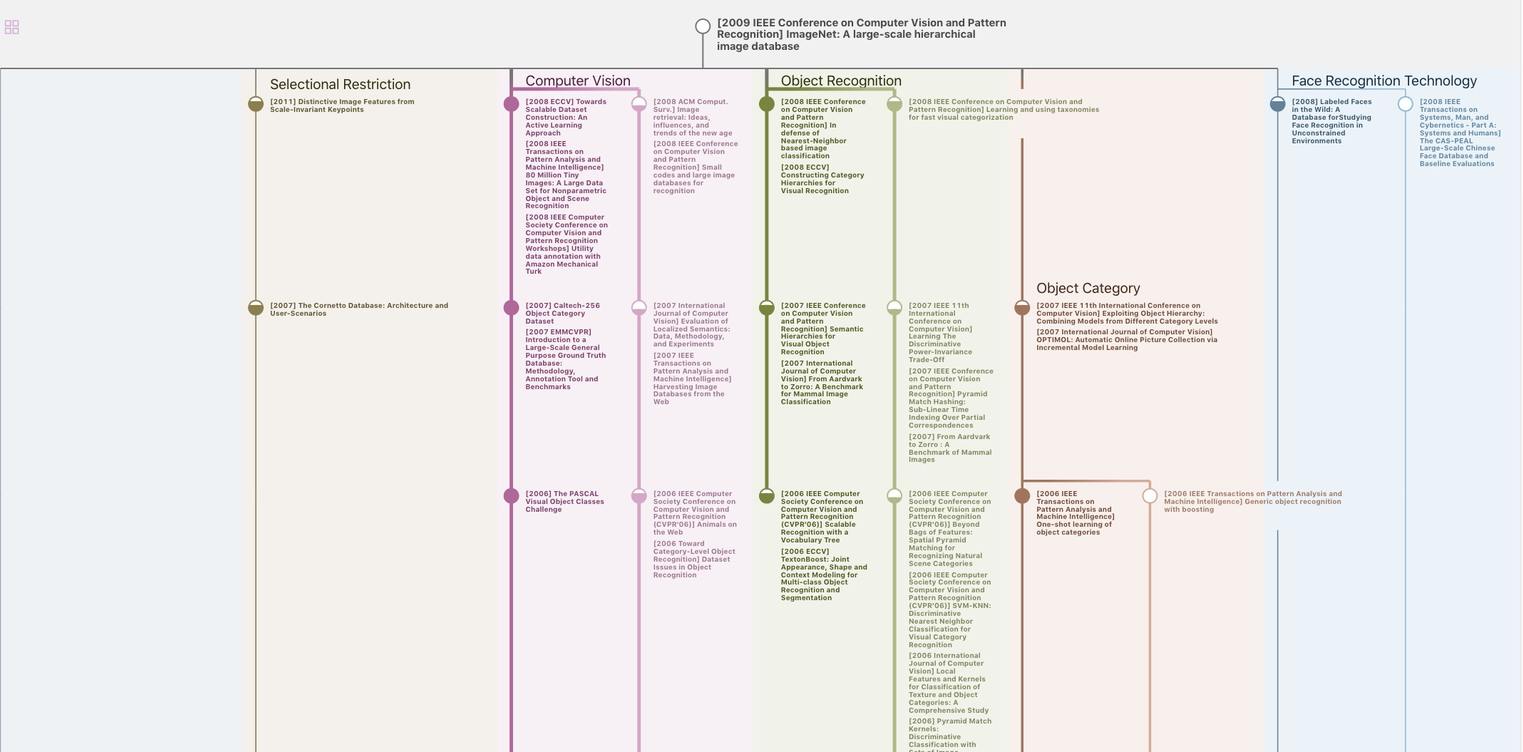
生成溯源树,研究论文发展脉络
Chat Paper
正在生成论文摘要