Weakly Supervised Target-Speaker Voice Activity Detection.
International Conference on Big Data Computing and Communications(2023)
摘要
Target Speaker Voice Activity Detection (TS-VAD) is a widely used technique for detecting the voice of a target speaker in the input audio stream. However, training TS-VAD model requires accurate frame-level labels indicating the temporal localization of the target speaker, which is labor-intensive for human-annotators especially when input audio contains overlapping segments. We aim to investigate how to train TS-VAD with clip-level labels which indicate the presence or absence of the target speaker’s voice in the audio stream, without accurate temporal duration information. This problem falls under the category of weakly supervised learning, however, we find that multiple instance learning, a popular weakly supervised learning framework, is not an effective solution for weakly supervised TS-VAD. In this work, we propose a novel weakly supervised training method for TS-VAD to explore the correlation between frame-level decisions and clip-level labels. Our method takes the frame-level decisions as weights of frame features of the input audio, and extracts the speaker embedding by using the weighted features. Our model is optimized to minimize the loss between speaker embedding similarity and clip-level label. Experiments show that our weakly supervised TS-VAD achieves 18.3% Event-F1, while the Event-F1 is only 5.8% by using the existing weakly supervised method.
更多查看译文
关键词
TS-VAD,Multiple Instance Learning,speaker diarization
AI 理解论文
溯源树
样例
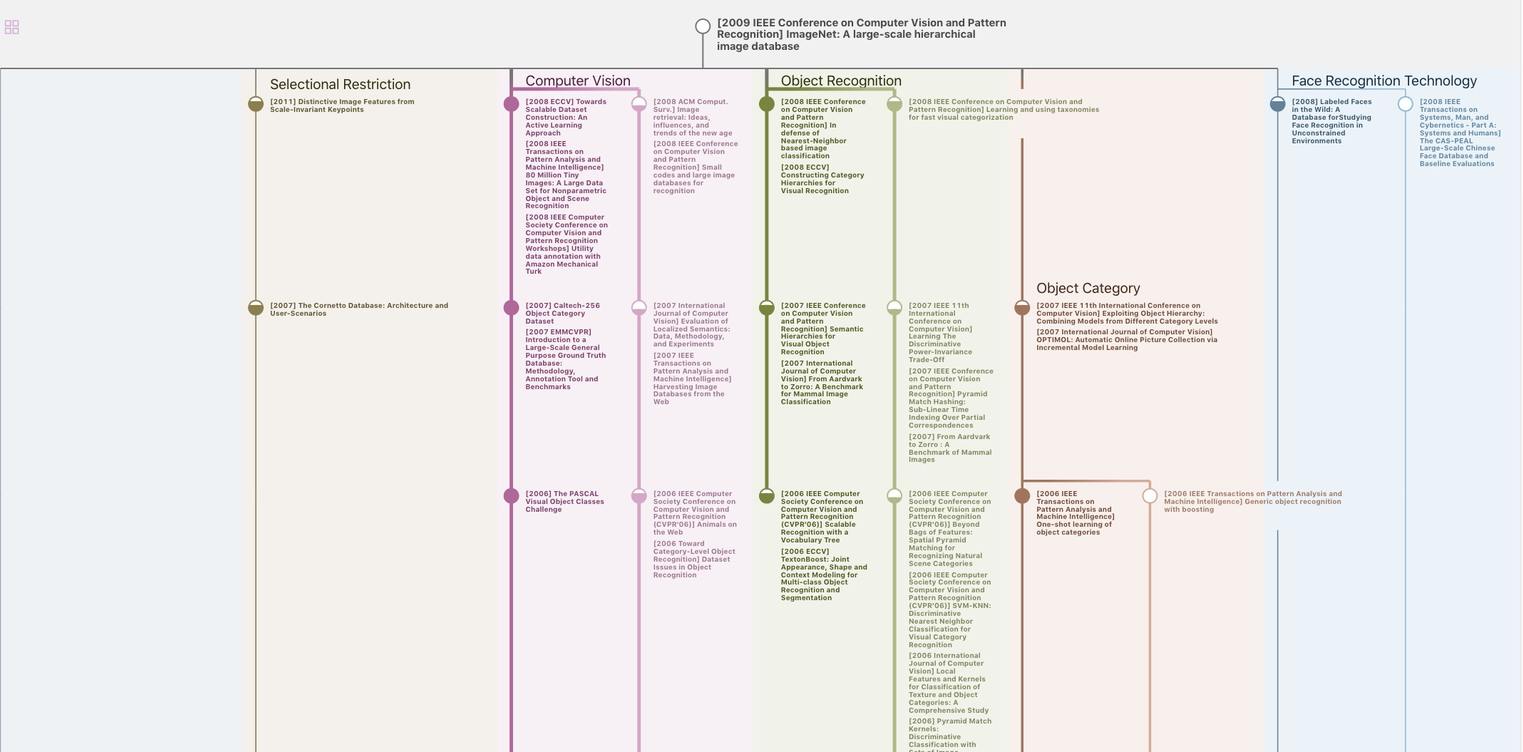
生成溯源树,研究论文发展脉络
Chat Paper
正在生成论文摘要