Poster: Fast GPU Inference with Unstructurally pruned DNNs for Explainable DOC
2023 IEEE/ACM SYMPOSIUM ON EDGE COMPUTING, SEC 2023(2023)
摘要
We have developed a code compiler to compress unstructurally pruned DNN models and demonstrated inference time less than 1 msec with AUC accuracy over 90 % for an anomaly detection task using MVTec AD dataset and edge Graphics Processing Unit (GPU) devices. Reduced RepVGG convolutional neural network (CNN) architecture is applied to an explainable deep one-class classification (XDOC) algorithm and such fast inference is obtained without sacrificing the accuracy by using a training scheme, CutPaste, to keep the accuracy high under an extremely higher pruning rate condition.
更多查看译文
关键词
unstructured pruning at initialization,Synaptic Flow,compiler,SparseRT,TensorRT,GPU,MVTec AD,CutPaste
AI 理解论文
溯源树
样例
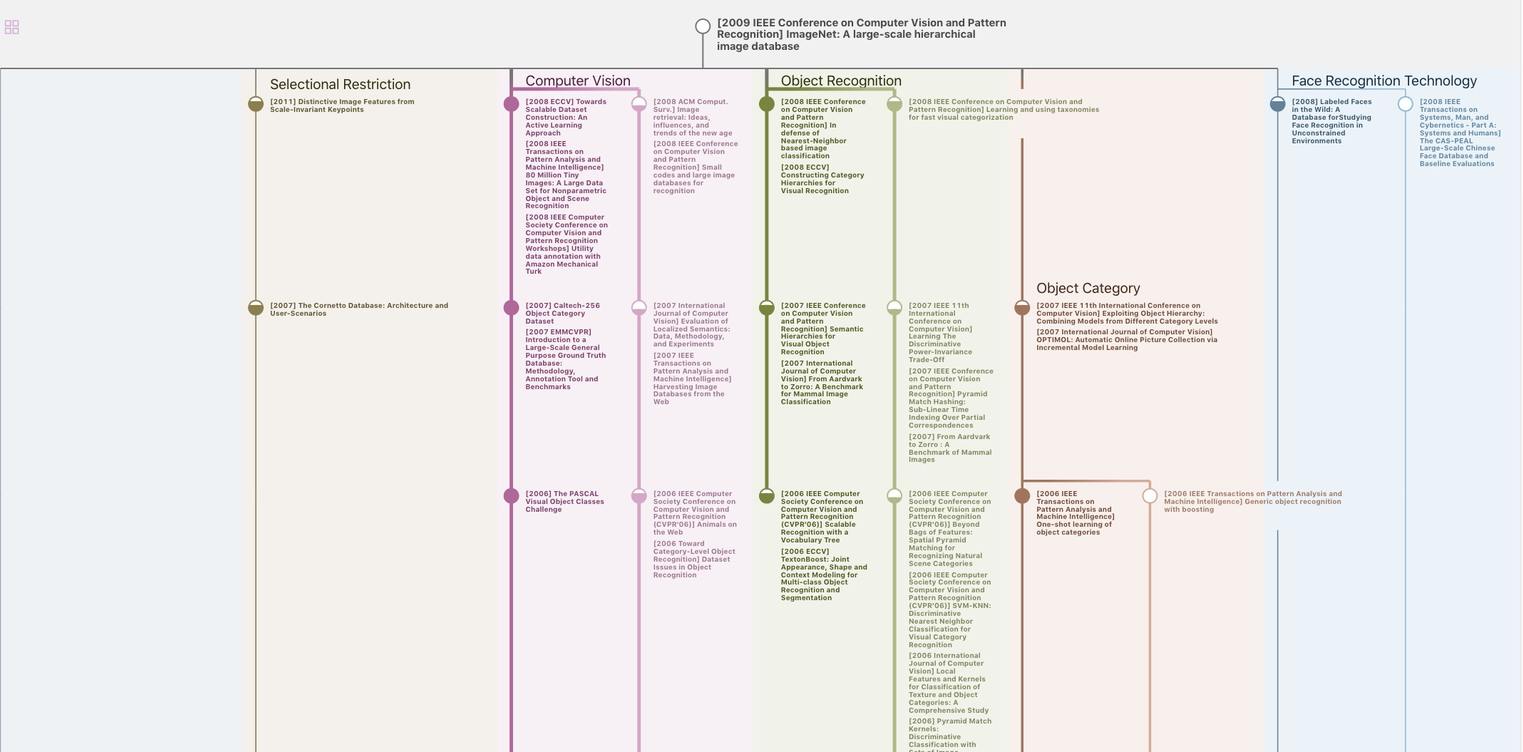
生成溯源树,研究论文发展脉络
Chat Paper
正在生成论文摘要