Gaussian Process-Based Nonlinear Moving Horizon Estimation
CoRR(2024)
摘要
In this paper, we propose a novel Gaussian process-based moving horizon
estimation (MHE) framework for unknown nonlinear systems. In the proposed
scheme, we take advantage of the properties of Gaussian processes. On the one
hand, we approximate the system dynamics by the posterior means of the learned
Gaussian processes (GPs). On the other hand, we exploit the posterior variances
of the Gaussian processes to design the weighting matrices in the MHE cost
function and account for the uncertainty in the learned system dynamics. The
data collection and the tuning of the hyperparameters are done offline. We
prove robust stability of the GP-based MHE scheme using a Lyapunov-based proof
technique. Furthermore, as additional contribution, we analyze under which
conditions incremental input/output-to-state stability (a nonlinear
detectability notion) is preserved when approximating the system dynamics
using, e.g., machine learning techniques. Finally, we illustrate the
performance of the GP-based MHE scheme in a simulation case study and show how
the chosen weighting matrices can lead to an improved performance compared to
standard cost functions.
更多查看译文
AI 理解论文
溯源树
样例
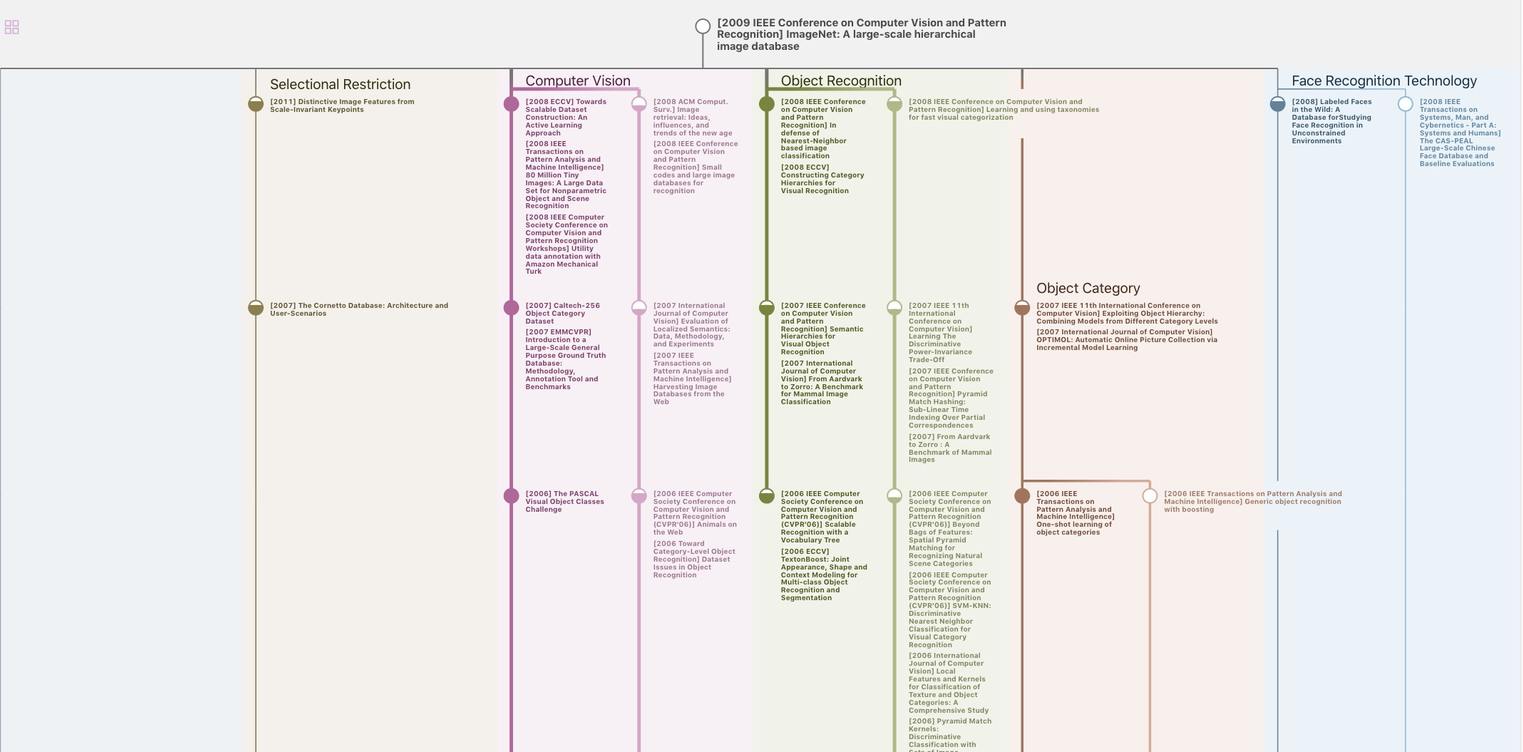
生成溯源树,研究论文发展脉络
Chat Paper
正在生成论文摘要