Combinatorial Optimization and Machine Learning for Dynamic Inventory Routing
arxiv(2024)
摘要
We introduce a combinatorial optimization-enriched machine learning pipeline
and a novel learning paradigm to solve inventory routing problems with
stochastic demand and dynamic inventory updates. After each inventory update,
our approach reduces replenishment and routing decisions to an optimal solution
of a capacitated prize-collecting traveling salesman problem for which
well-established algorithms exist. Discovering good prize parametrizations is
non-trivial; therefore, we have developed a machine learning approach. We
evaluate the performance of our pipeline in settings with steady-state and more
complex demand patterns. Compared to previous works, the policy generated by
our algorithm leads to significant cost savings, achieves lower inference time,
and can even leverage contextual information.
更多查看译文
AI 理解论文
溯源树
样例
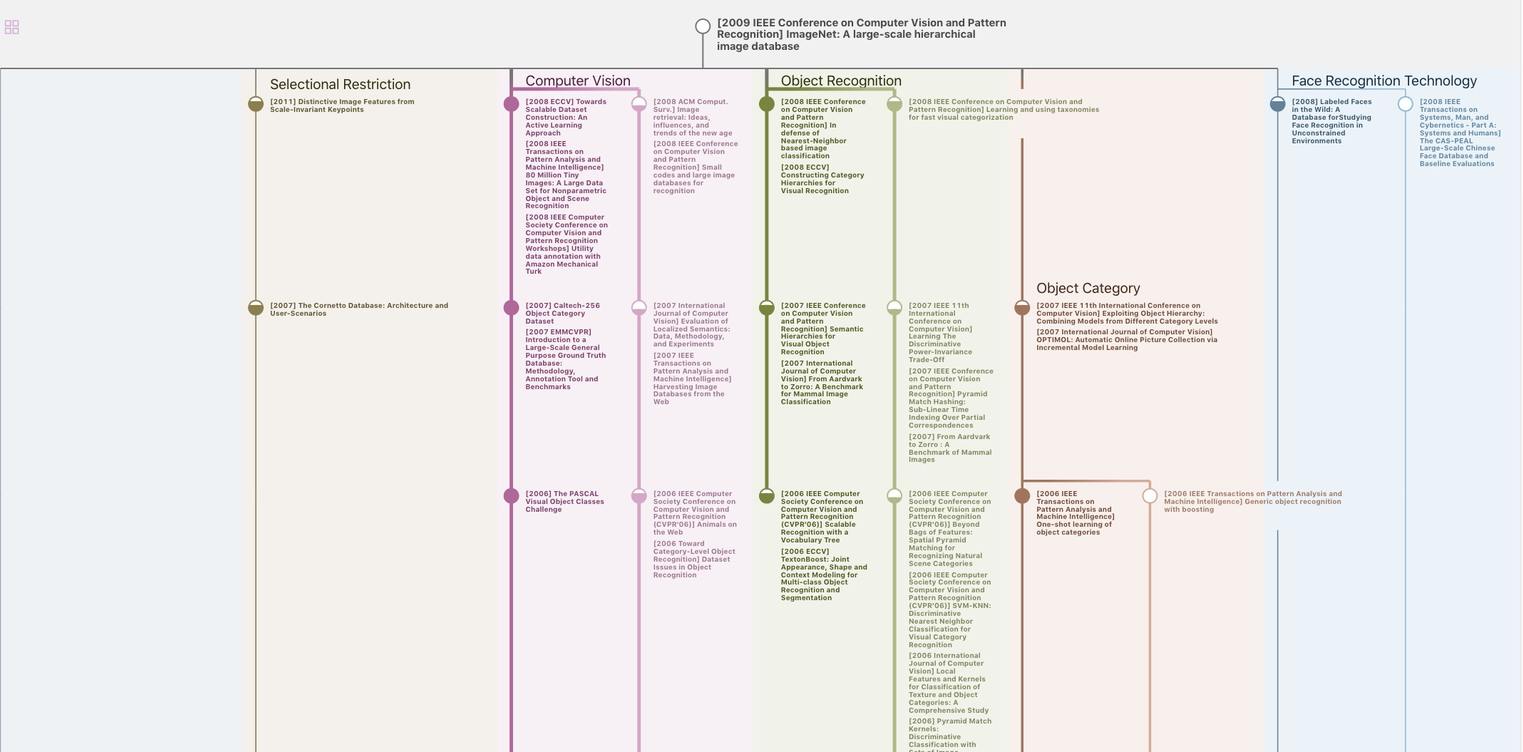
生成溯源树,研究论文发展脉络
Chat Paper
正在生成论文摘要