Brief Industry Paper: Evaluating Robustness of Deep Learning-Based Recommendation Systems Against Hardware Errors: A Case Study.
2023 IEEE Real-Time Systems Symposium (RTSS)(2023)
摘要
Deep learning-based recommendation systems (DL-RMs) are industry-scale recommendation models developed by Meta, designed to make use of both categorical and numerical inputs to make personalized recommendations. To serve billions of users in real-time, DLRMs rely on high-performance hardware and accelerators within our data centers, optimizing for execution latency and recommendation quality. However, continuous technology scaling, expanding workload, and increasing hardware heterogeneity could lead to increased risk of hardware errors. Addressing this risk often involves introducing extra design redundancy, which can pose a non-negligible overhead in performance and latency. In this paper, we present a case study of evaluating DLRM robustness against hardware errors by performing an extensive error injection campaign to DLRM. Our findings unveil that DLRM is notably robust to hardware errors and we further find that embedding tables in DLRM show an especially strong robustness. Additionally, we explore a software-level error mitigation techniques, activation clipping, for mitigating the hardware errors, which improves the DLRM robustness further. This industrial case study of understanding and improving DLRM robustness can enable the system to continue to deliver timely recommendations even in the presence of hardware challenges, or reduce the timing latency overhead posed by design redundancy, enhancing overall recommendation system performance.
更多查看译文
关键词
recommendation systems,hardware resilience,deep learning,ai robustness
AI 理解论文
溯源树
样例
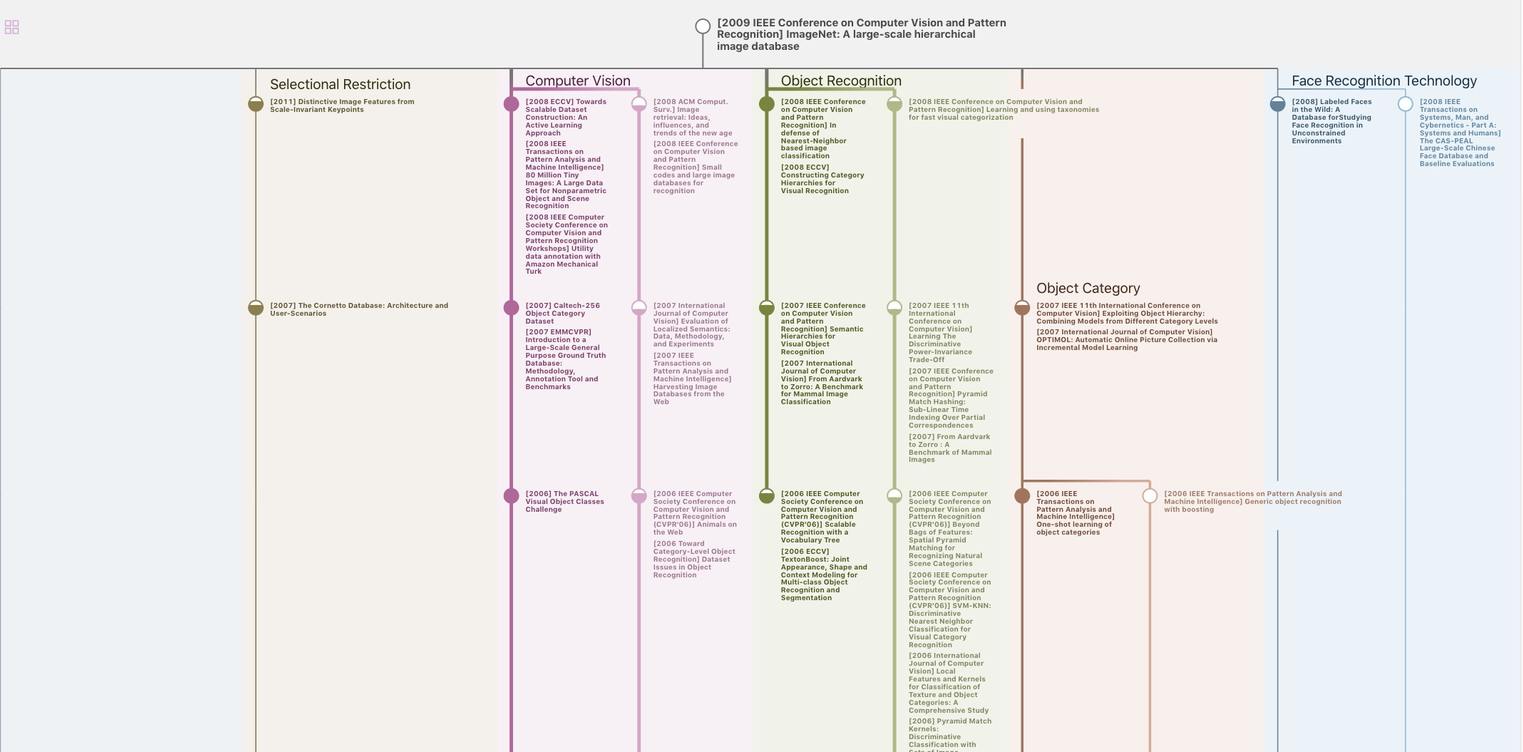
生成溯源树,研究论文发展脉络
Chat Paper
正在生成论文摘要