PROTEUS: Domain Adaptation for Dynamic Features in AI-assisted Windows Malware Detection
2023 23RD IEEE INTERNATIONAL CONFERENCE ON DATA MINING WORKSHOPS, ICDMW 2023(2023)
摘要
Machine learning and deep learning (ML/DL) have demonstrated their capability in malware detection. Many ML/DL models have been developed for malware detection using features extracted from dynamic analysis. Most of these models are based on API calls, e.g., API sequence, API occurrence, or API frequency, which may change due to the evolution of operating systems. For instance, the list of APIs available in Windows 10 differs from that of Windows 7. It causes the performance degradation of malware detection models when deploying across domains, e.g., using a model trained with Windows 7 features on a Windows 10 system. Maintaining multiple models, each for a specific domain, is challenging and costly due to the technical complexity and execution overhead of dynamic analysis. In this paper, we develop a domain adaptation technique named Proteus for malware detection models. Given a small number of feature vectors in both domains (namely source and target domains), we construct a transformation matrix using the least square approximation technique. The transformation matrix enables the conversion of feature vectors among domains. We run experiments with a recent dataset with around 84979 samples validated with VirusTotal. The experimental results show that the proposed technique achieves a detection accuracy of 91.15%, approximating the upper-bound performance of the model trained in the target domain with the entire dataset. The proposed approach also significantly reduces the overhead of training and maintaining the model of the target domain. The code is publicly available at https://github.com/keyplay/proteus.
更多查看译文
关键词
Malware Detection,Domain Adaptation,Machine,Learning,Dynamic Malware Analysis
AI 理解论文
溯源树
样例
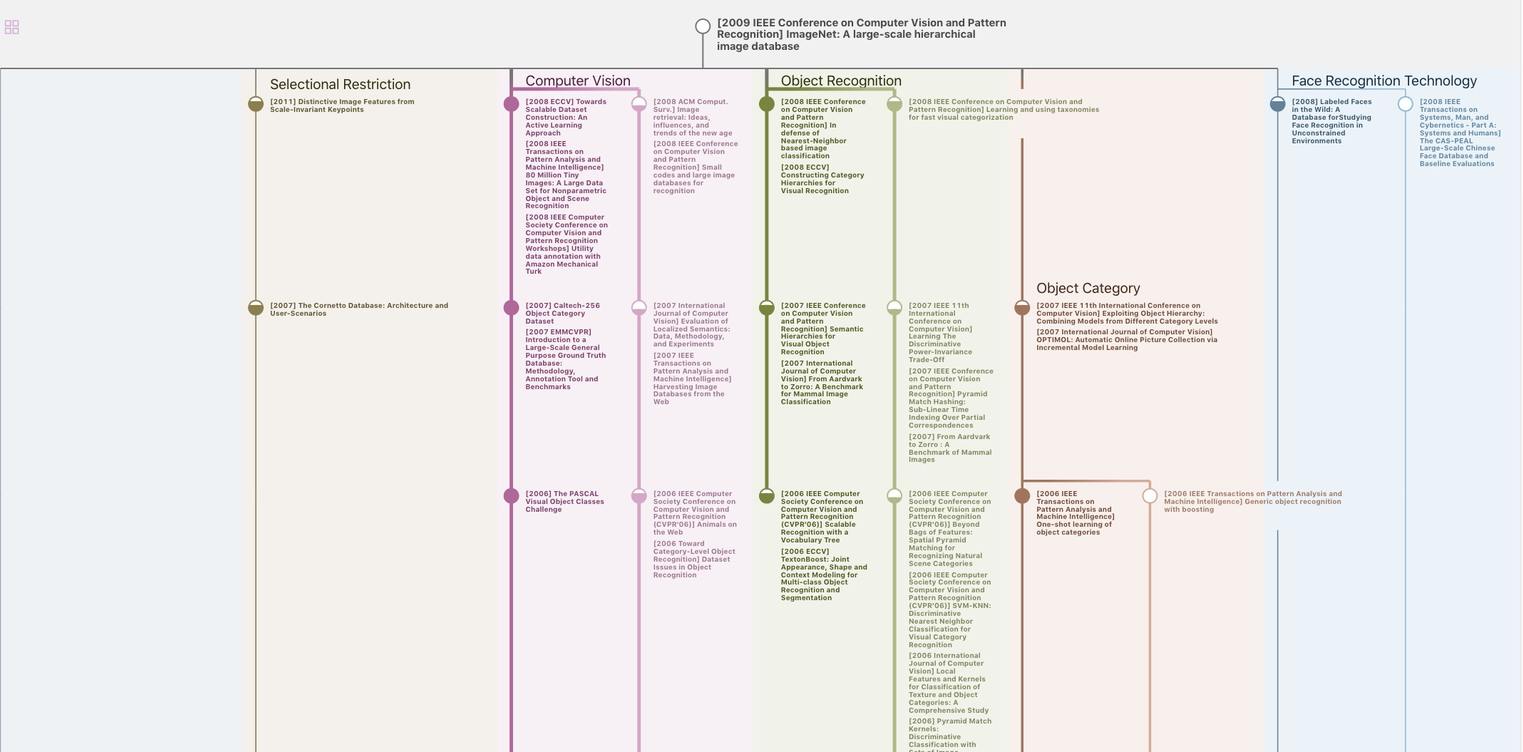
生成溯源树,研究论文发展脉络
Chat Paper
正在生成论文摘要