Compound Returns Reduce Variance in Reinforcement Learning
CoRR(2024)
摘要
Multistep returns, such as n-step returns and λ-returns, are
commonly used to improve the sample efficiency of reinforcement learning (RL)
methods. The variance of the multistep returns becomes the limiting factor in
their length; looking too far into the future increases variance and reverses
the benefits of multistep learning. In our work, we demonstrate the ability of
compound returns – weighted averages of n-step returns – to reduce
variance. We prove for the first time that any compound return with the same
contraction modulus as a given n-step return has strictly lower variance. We
additionally prove that this variance-reduction property improves the
finite-sample complexity of temporal-difference learning under linear function
approximation. Because general compound returns can be expensive to implement,
we introduce two-bootstrap returns which reduce variance while remaining
efficient, even when using minibatched experience replay. We conduct
experiments showing that two-bootstrap returns can improve the sample
efficiency of n-step deep RL agents, with little additional computational
cost.
更多查看译文
AI 理解论文
溯源树
样例
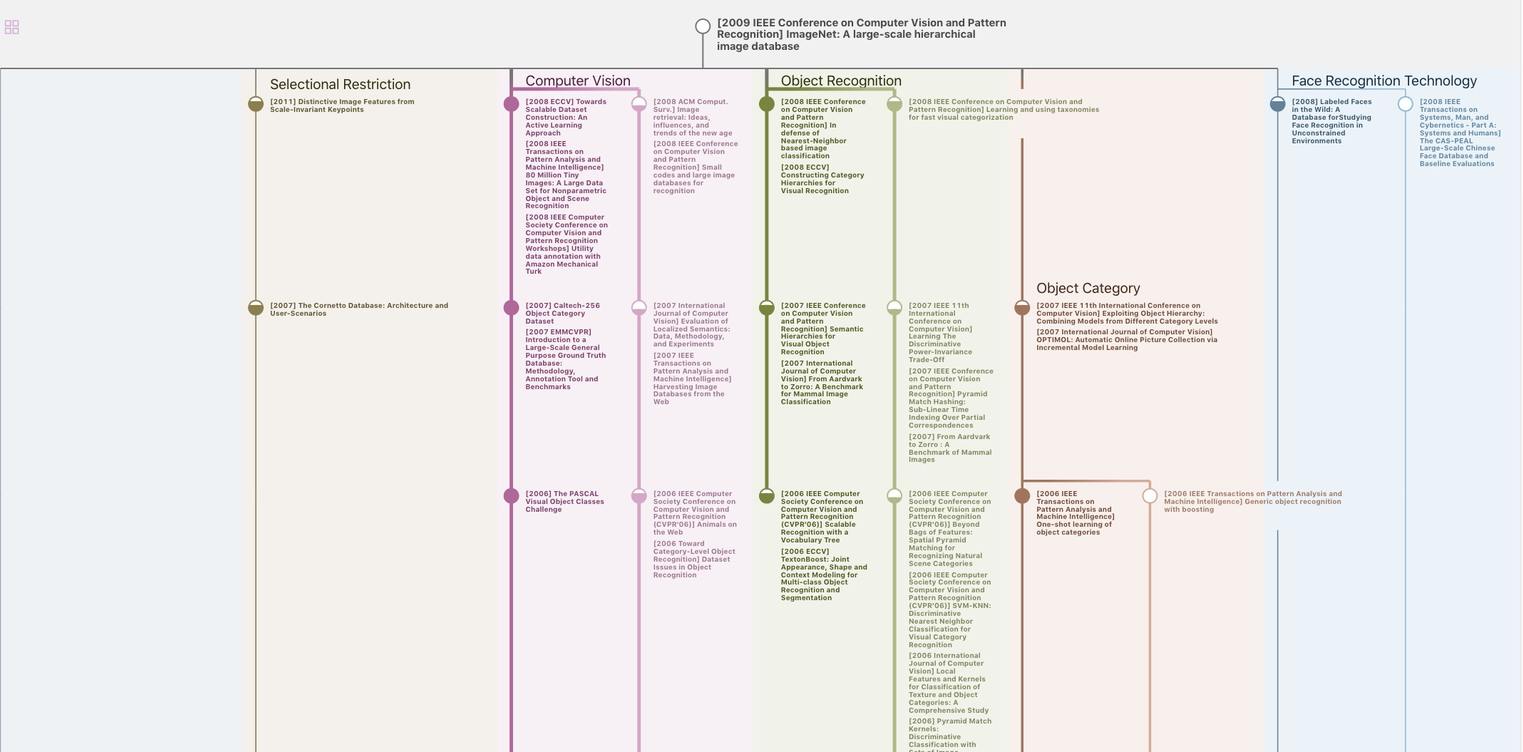
生成溯源树,研究论文发展脉络
Chat Paper
正在生成论文摘要