Personalized Federated Semi -Supervised Learning with Black -Box Models
23RD IEEE INTERNATIONAL CONFERENCE ON DATA MINING, ICDM 2023(2023)
摘要
Federated Semi -Supervised Learning alleviates the necessity for fully labeled data in Federated Learning. However, it does not sufficiently prioritize model privacy or the personalized requirements of clients. To address these concerns, our key idea is to communicate no longer individual model parameters but their black-box models, implying to provide other clients with only an input-output interface of individual models. The communication mechanism is model -agnostic, thereby facilitating adaption to heterogeneous models for each client through customization. Consequently, we propose a framework called Personalized Federated Semi -Supervised Learning with Black-Box Models ((BPFSSL)-P-2) to enhance the privacy of communication. To prevent negative knowledge transfer due to data heterogeneity, we design a two-stage strategy that filters at both the model and data levels, enabling clients to obtain large training datasets by including more high-quality pseudo-labeled data under conditions of scarce labeled data. The experimental results indicate that (BPFSSL)-P-2 achieves competitive performance while reducing the amount of information exposed during communication. Furthermore, it can foster productive collaboration among diverse model architectures in model heterogeneous Federated Learning.
更多查看译文
关键词
n/a
AI 理解论文
溯源树
样例
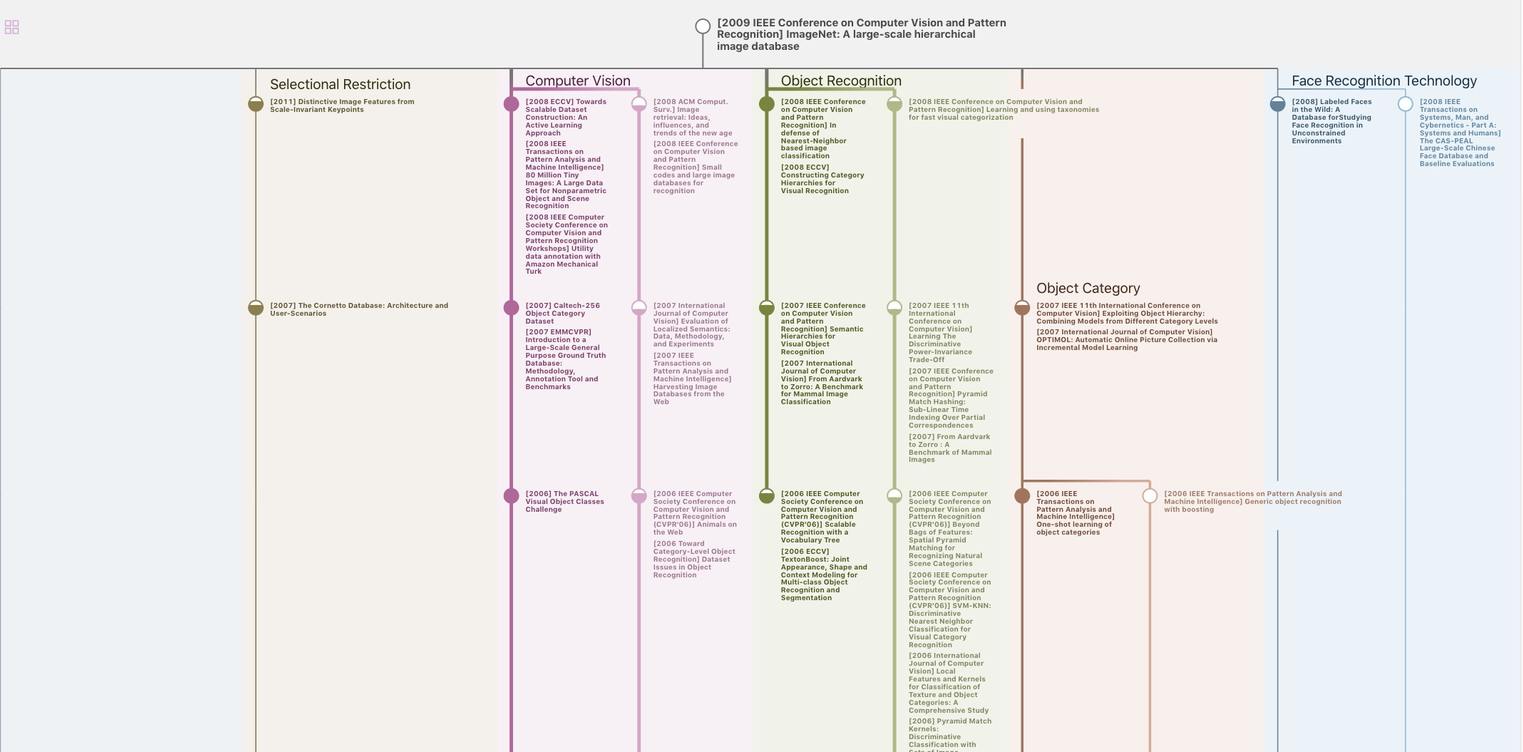
生成溯源树,研究论文发展脉络
Chat Paper
正在生成论文摘要