RDKG: A Reinforcement Learning Framework for Disease Diagnosis on Knowledge Graph
23RD IEEE INTERNATIONAL CONFERENCE ON DATA MINING, ICDM 2023(2023)
摘要
Automatic disease diagnosis from symptoms has attracted much attention in medical practices. It can assist doctors and medical practitioners in narrowing down disease candidates, reducing testing costs, improving diagnosis efficiency, and more importantly, saving human lives. Existing research has made significant progress in diagnosing disease but was limited by the gap between interpretability and accuracy. To fill this gap, in this paper, we propose a method called Reinforced Disease Diagnosis on Knowlege Graph (RDKG). Specifically, we first construct a knowledge graph containing all information from electronic medical records. To capture informative embeddings, we propose an enhanced knowledge graph embedding method that can embed information outside the knowledge graph into entity embedding. Then we transform the automatic disease diagnosis task into a Markov decision process on the knowledge graph. After that, we design a reinforcement learning method with a soft reward mechanism and a pruning strategy to solve the Markov decision process. We accomplish automated disease diagnosis by finding a path from symptoms to disease. The experimental results show that our model can effectively utilize heterogeneous information in the knowledge graph to complete the automatic disease diagnosis. Besides, our model demonstrates supreme performance in both accuracy and interpretability.
更多查看译文
关键词
Electronic Medical Records,medical knowledge graph,reinforcement learning,automatic disease diagnosis
AI 理解论文
溯源树
样例
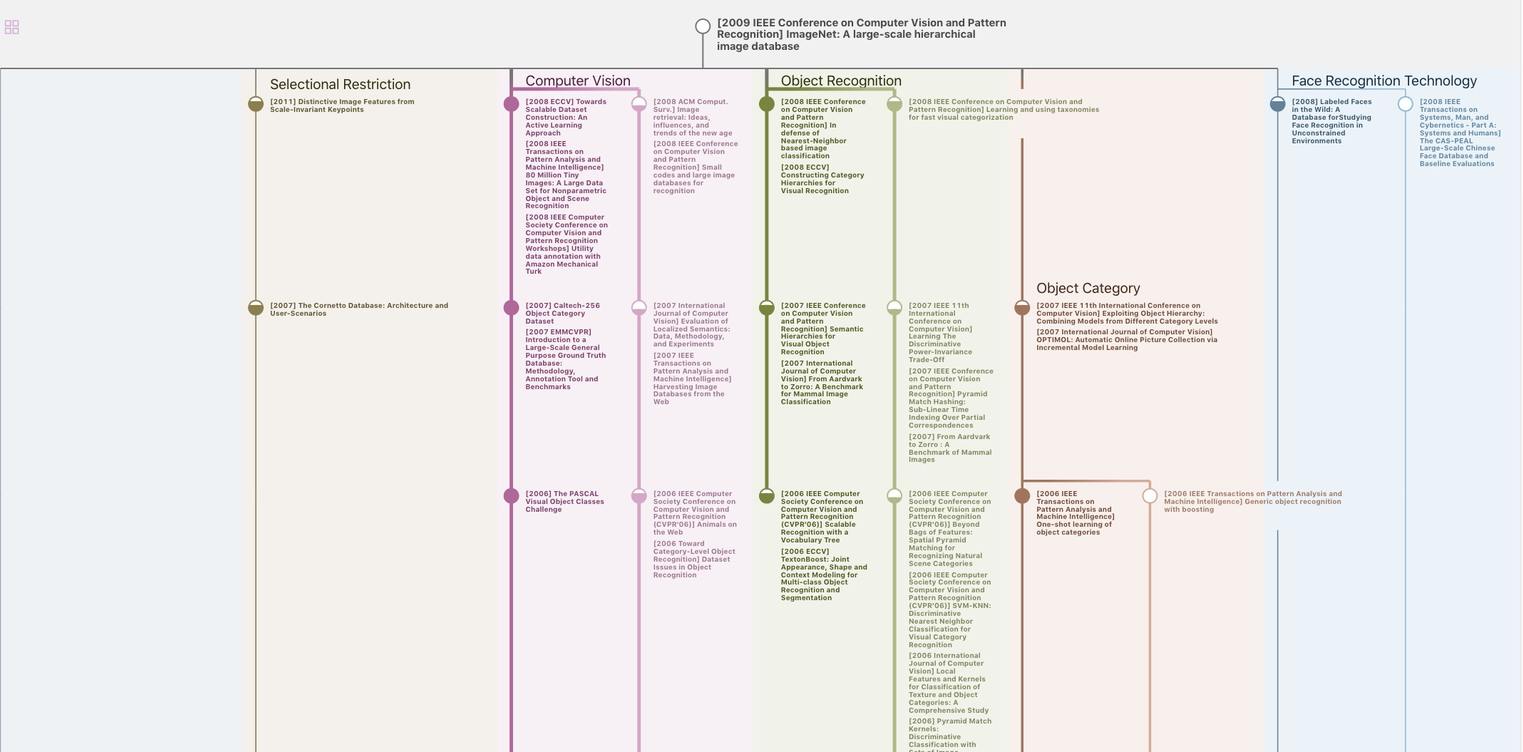
生成溯源树,研究论文发展脉络
Chat Paper
正在生成论文摘要