Graph Sampling based Fairness -aware Recommendation over Sensitive Attribute Removal
23RD IEEE INTERNATIONAL CONFERENCE ON DATA MINING, ICDM 2023(2023)
摘要
Discrimination against different user groups has received growing attention in the recommendation field. To address this problem, existing works typically remove sensitive attributes that may cause discrimination through adversary learning to achieve fair recommendations. However, these approaches leverage all available interactions for learning user representations and overlook the fact that different interactions have varying relevance to users' sensitive attributes. Ignoring this issue may weaken the effectiveness of adversary learning in removing sensitive attributes. To tackle this challenge, we propose a novel model called GS-FairRec, which distinguishes between user interactions to achieve better removal of sensitive attributes. The model consists of three modules: graph sampling -based representation learning, pseudo -user representation learning, and adversarial learning. Firstly, the graph sampling-based representation learning module removes some irrelevant neighbors from a user-item bipartite graph and employs a graph convolutional network (GCN) to learn user/item representations. Next, items that are relevant to a user's sensitive information but do not match their preferences are defined as the user's pseudo-interest items, which are leveraged to learn the pseudo-user representation. In the adversarial learning module, the user's two kinds of representations are fused for adversarial learning to remove sensitive information. Additionally, we design a new metric to measure the model's ability to remove sensitive attributes based on how a generated recommendation list discloses the user's sensitive attributes. Finally, we conduct experiments on two real-world datasets, and our results demonstrate the superiority of our proposed model in fairness tasks.
更多查看译文
关键词
Terms Recommender System,Graph Neural Network,Fairness
AI 理解论文
溯源树
样例
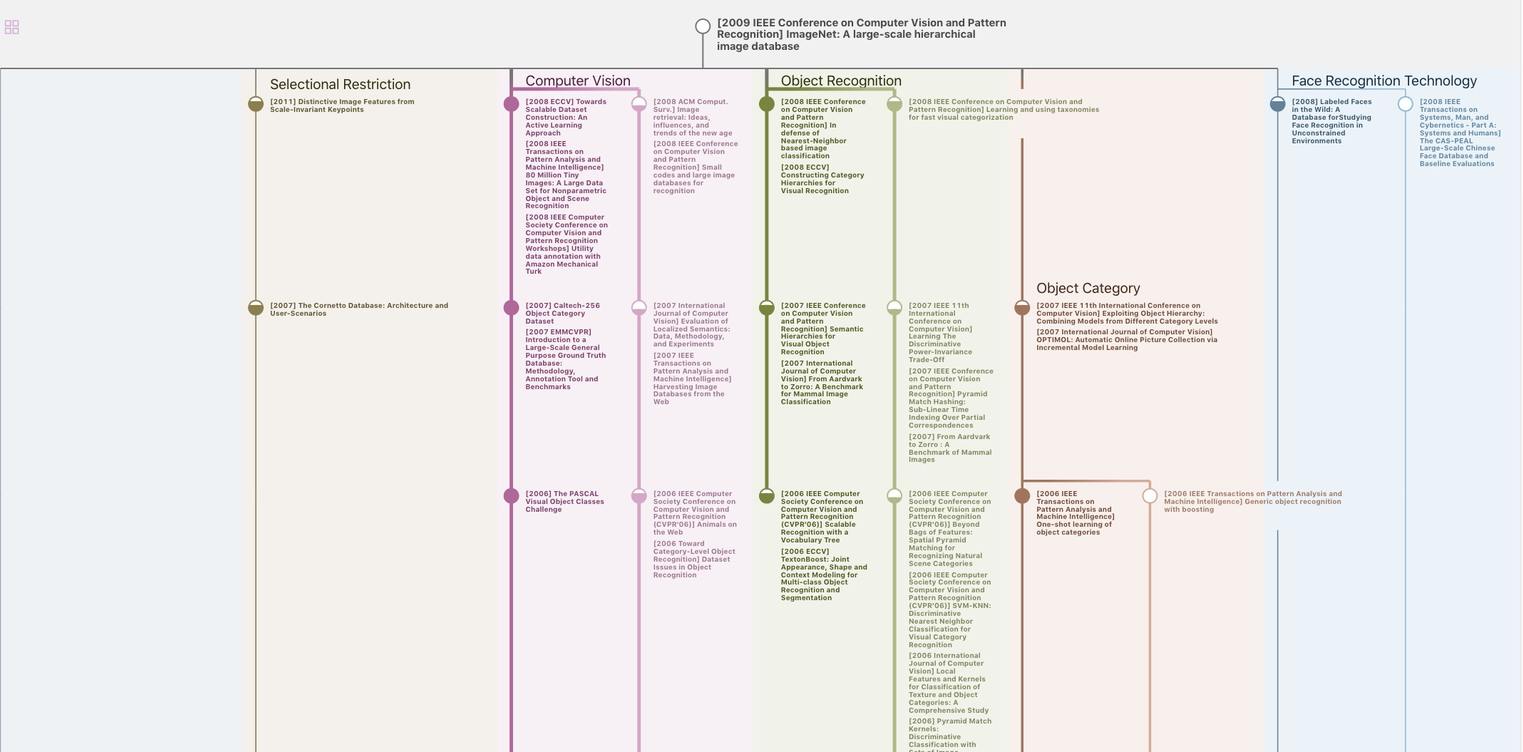
生成溯源树,研究论文发展脉络
Chat Paper
正在生成论文摘要