Federated Learning for Privacy -Preserving Prediction of Occupational Group Mobility Using Multi -Source Mobile Data
23RD IEEE INTERNATIONAL CONFERENCE ON DATA MINING, ICDM 2023(2023)
摘要
This paper focuses on the mobility prediction problem of specific occupational groups that rely on mobile devices, predicting the mobilities of these groups using data shared across different platforms. While the mobility patterns of general populations have been studied extensively, predicting the movements of specific occupational groups like ride-hailing drivers, who frequently move over long distances, requires a more nuanced approach. This paper introduces FedOGM, a federated learning framework designed to predict the mobility of specific occupational groups while preserving user privacy. The framework utilizes a dynamic bidirectional graph attention network model DyBGAT for predicting the edge weights of an occupational dynamic Origin-Destination graph, representing the mobility behavior. In order to address key challenges in federated learning, FedOGM integrates By leveraging multi-source data from users' mobile devices and extracting unique occupational features and occupational features into the framework, such as applications usage duration feature, location switching feature, and call duration feature. Assisted by these personalized occupational features, we have devised a client selection algorithm, generated occupational dynamic OD graphs to diminish communication overhead, and proposed a group-level FedAvg aggregation method. Experiments conducted on real-world datasets of ride-hailing drivers, ride-hailing truck drivers, and real estate salespersons, including scenarios with false data, confirm the efficacy of the proposed methods in both mobility prediction and privacy protection.
更多查看译文
关键词
Federated Learning,Group Mobility Behaviour,Multi-source Mobile Data,Mobile Edge Networks
AI 理解论文
溯源树
样例
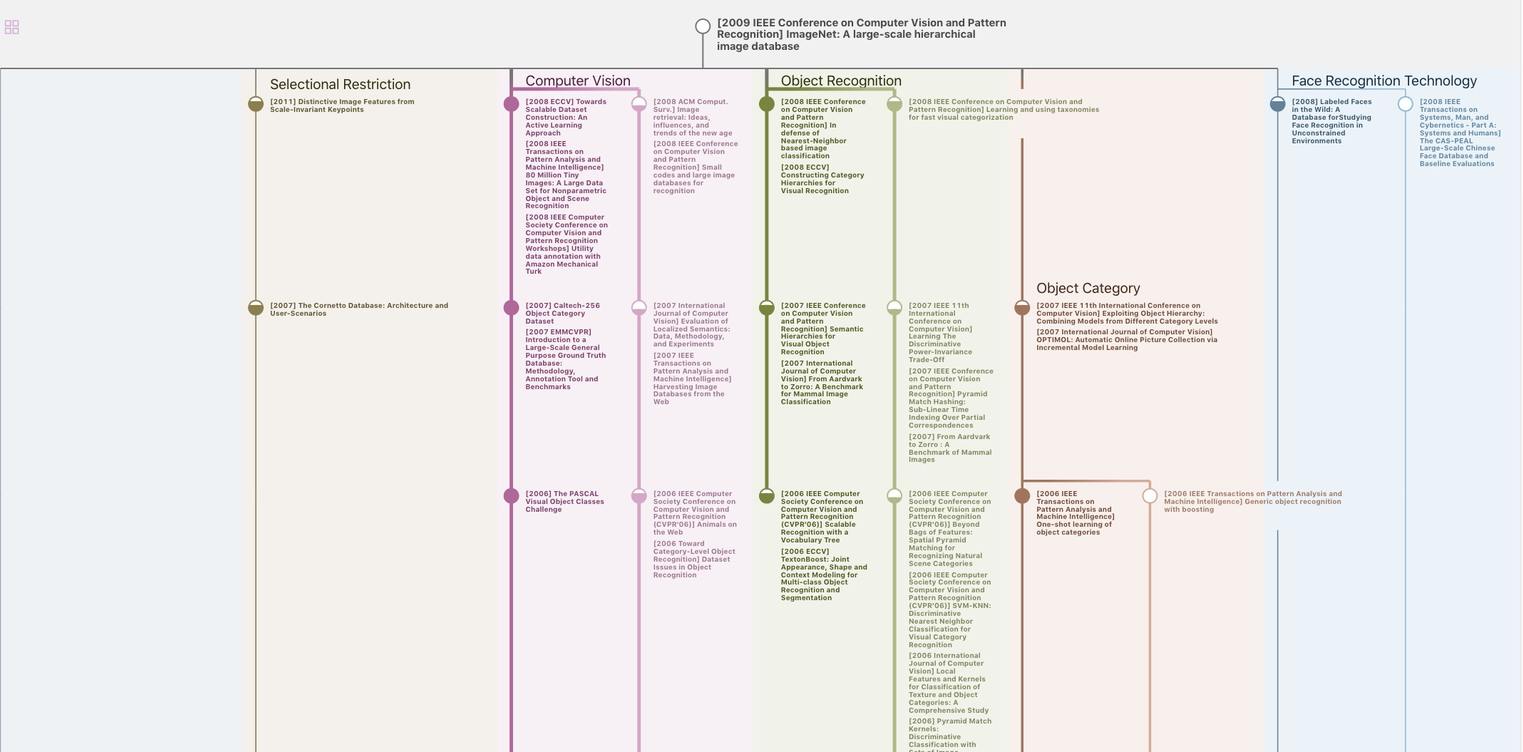
生成溯源树,研究论文发展脉络
Chat Paper
正在生成论文摘要