SynthDST: Synthetic Data is All You Need for Few-Shot Dialog State Tracking
CoRR(2024)
摘要
In-context learning with Large Language Models (LLMs) has emerged as a
promising avenue of research in Dialog State Tracking (DST). However, the
best-performing in-context learning methods involve retrieving and adding
similar examples to the prompt, requiring access to labeled training data.
Procuring such training data for a wide range of domains and applications is
time-consuming, expensive, and, at times, infeasible. While zero-shot learning
requires no training data, it significantly lags behind the few-shot setup.
Thus, `Can we efficiently generate synthetic data for any dialogue
schema to enable few-shot prompting?' Addressing this question, we propose
, a data generation framework tailored for DST, utilizing LLMs. Our
approach only requires the dialogue schema and a few hand-crafted dialogue
templates to synthesize natural, coherent, and free-flowing dialogues with DST
annotations. Few-shot learning using data from results in 4-5
improvement in Joint Goal Accuracy over the zero-shot baseline on MultiWOZ 2.1
and 2.4. Remarkably, our few-shot learning approach recovers nearly98
the performance compared to the few-shot setup using human-annotated training
data. Our synthetic data and code can be accessed at
https://github.com/apple/ml-synthdst
更多查看译文
AI 理解论文
溯源树
样例
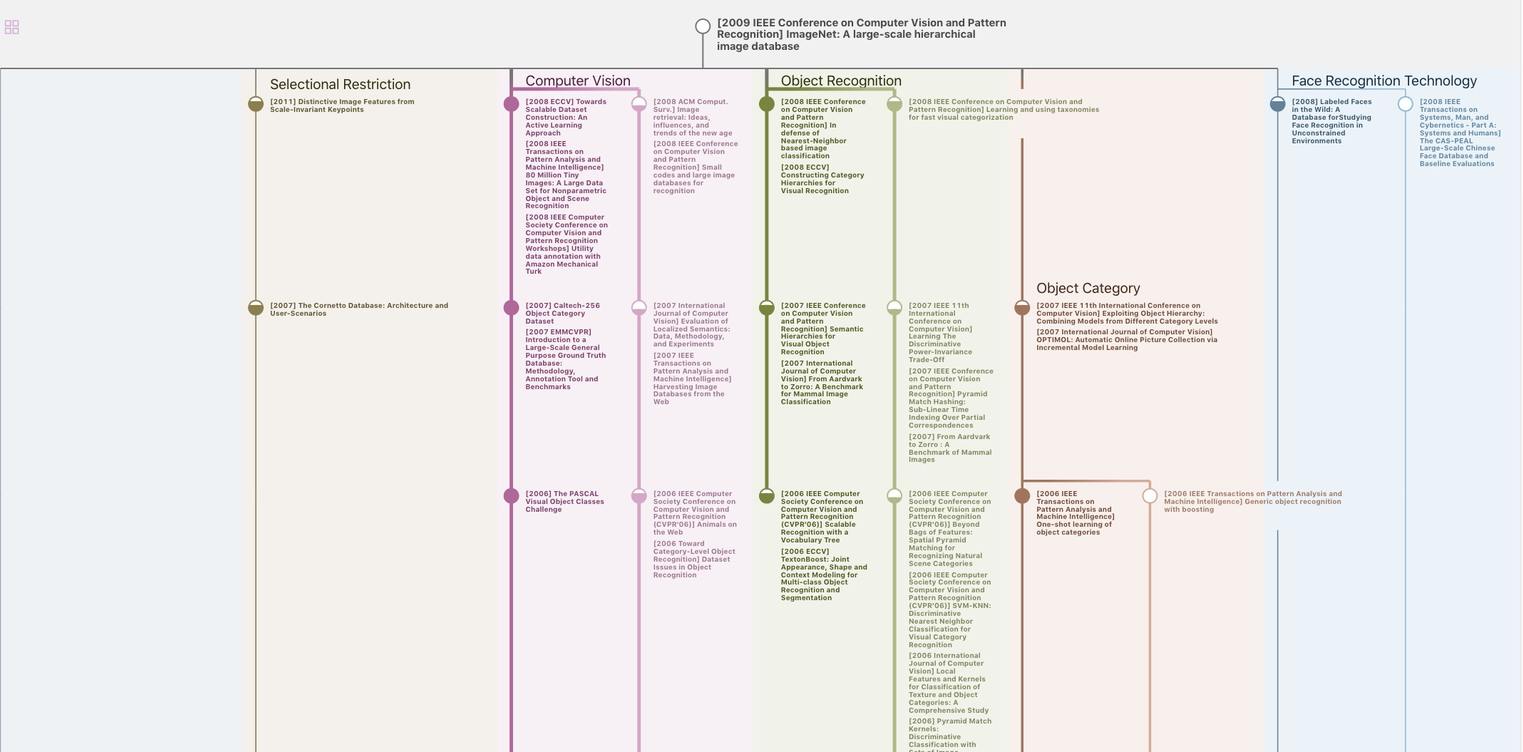
生成溯源树,研究论文发展脉络
Chat Paper
正在生成论文摘要