Assistive Large Language Model Agents for Socially-Aware Negotiation Dialogues
CoRR(2024)
摘要
In this work, we aim to develop LLM agents to mitigate social norm violations
in negotiations in a multi-agent setting. We simulate real-world negotiations
by letting two large Language Models (LLMs) play the roles of two negotiators
in each conversation. A third LLM acts as a remediation agent to rewrite
utterances violating norms for improving negotiation outcomes. As it is a novel
task, no manually constructed data is available. To address this limitation, we
introduce a value impact based In-Context Learning (ICL) method to identify
high-quality ICL examples for the LLM-based remediation agents, where the value
impact function measures the quality of negotiation outcomes. We show the
connection of this method to policy learning and provide rich empirical
evidence to demonstrate its effectiveness in negotiations across three
different topics: product sale, housing price, and salary negotiation. The
source code and the generated dataset will be publicly available upon
acceptance.
更多查看译文
AI 理解论文
溯源树
样例
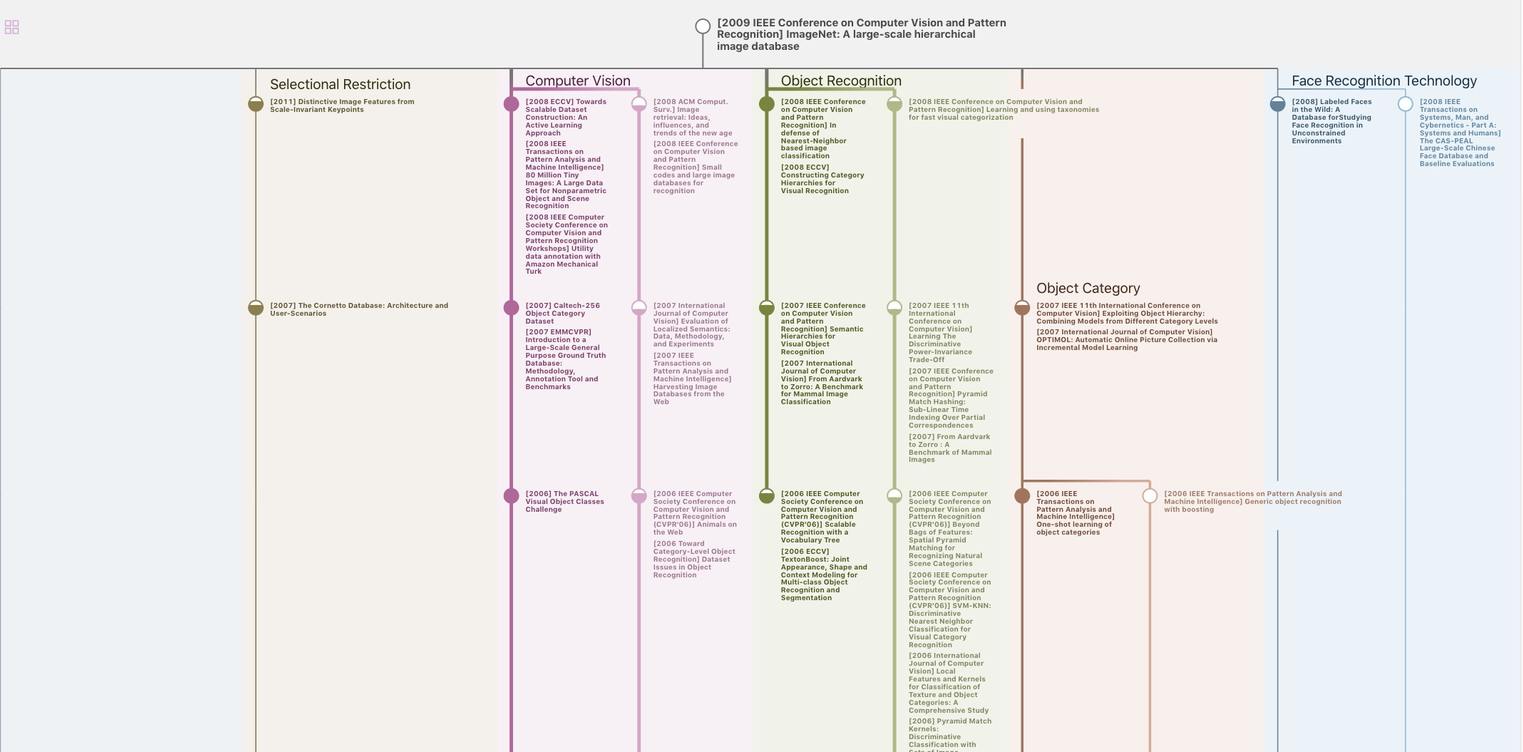
生成溯源树,研究论文发展脉络
Chat Paper
正在生成论文摘要