2AFC Prompting of Large Multimodal Models for Image Quality Assessment
CoRR(2024)
摘要
While abundant research has been conducted on improving high-level visual
understanding and reasoning capabilities of large multimodal models (LMMs),
their visual quality assessment (IQA) ability has been relatively
under-explored. Here we take initial steps towards this goal by employing the
two-alternative forced choice (2AFC) prompting, as 2AFC is widely regarded as
the most reliable way of collecting human opinions of visual quality.
Subsequently, the global quality score of each image estimated by a particular
LMM can be efficiently aggregated using the maximum a posterior estimation.
Meanwhile, we introduce three evaluation criteria: consistency, accuracy, and
correlation, to provide comprehensive quantifications and deeper insights into
the IQA capability of five LMMs. Extensive experiments show that existing LMMs
exhibit remarkable IQA ability on coarse-grained quality comparison, but there
is room for improvement on fine-grained quality discrimination. The proposed
dataset sheds light on the future development of IQA models based on LMMs. The
codes will be made publicly available at https://github.com/h4nwei/2AFC-LMMs.
更多查看译文
AI 理解论文
溯源树
样例
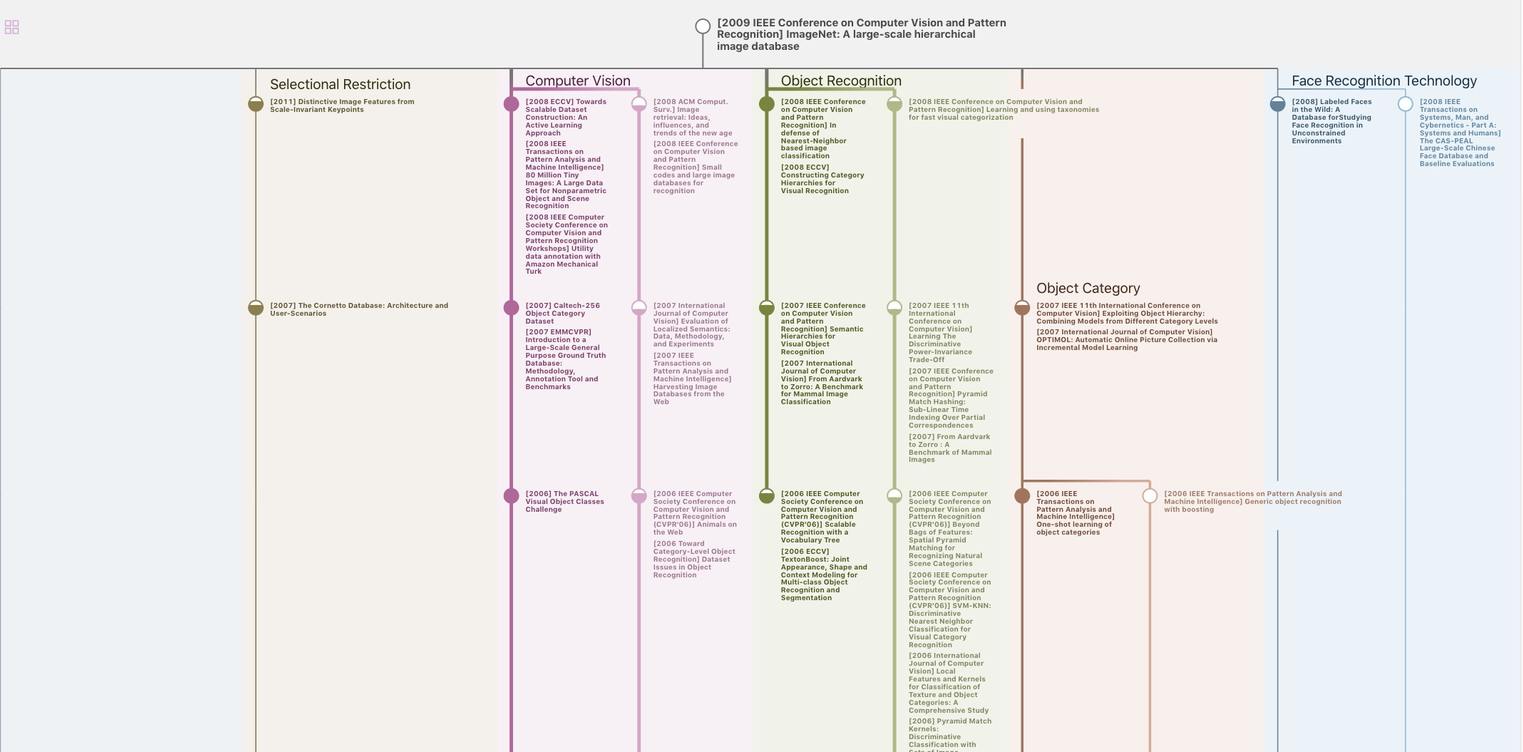
生成溯源树,研究论文发展脉络
Chat Paper
正在生成论文摘要